1. Market Prediction
AI analyzes vast amounts of historical and real-time market data to predict market trends and price movements, enabling traders to make more informed decisions.
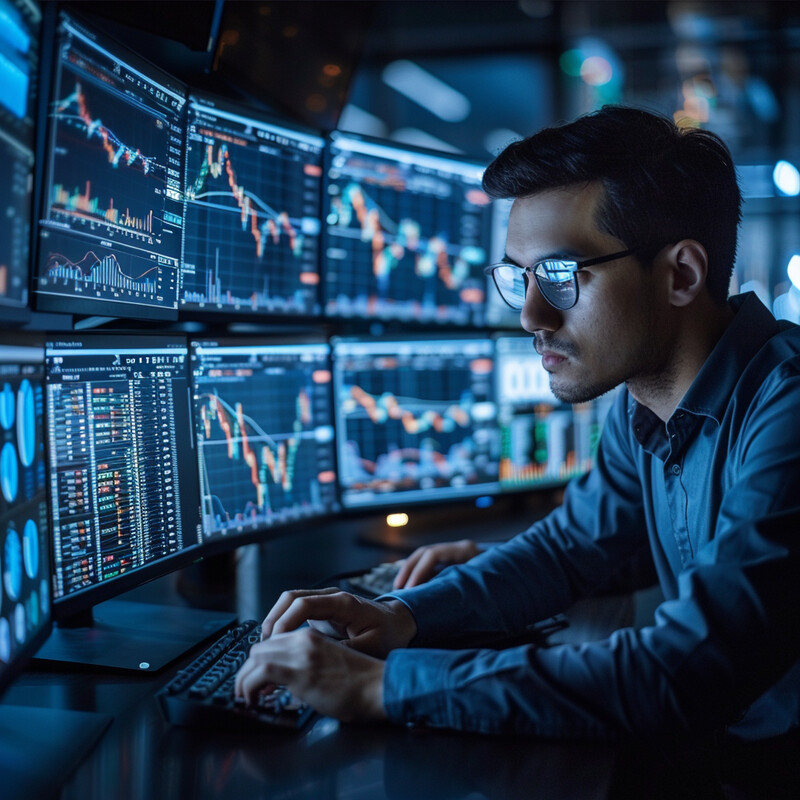
AI leverages historical data, real-time market updates, and complex mathematical models to predict market trends and asset price movements. These predictions are based on patterns and correlations identified within vast datasets that human analysts might not readily discern. By providing forward-looking insights, AI enables traders and investors to anticipate market movements and position their portfolios accordingly.
2. High-Frequency Trading (HFT)
AI excels in high-frequency trading, where algorithms execute thousands of orders at speeds faster than any human could, exploiting tiny price differences and market inefficiencies.
-1.jpg)
In high-frequency trading, AI algorithms play a crucial role by executing orders at speeds and volumes that are impossible for human traders. These algorithms can capitalize on very small price discrepancies and market inefficiencies, executing transactions in milliseconds based on pre-set criteria, thereby maximizing profits from minimal price movements across many transactions.
3. Risk Management
AI helps in assessing and managing risk by analyzing market conditions, portfolio exposure, and other risk factors more accurately and dynamically.
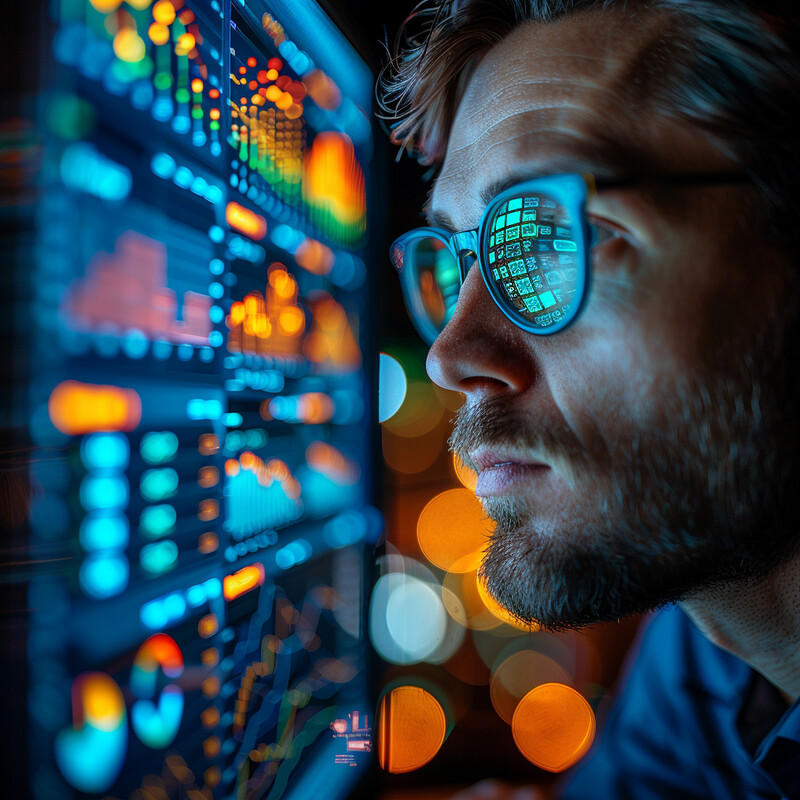
AI significantly enhances risk management capabilities by dynamically analyzing market data and external variables to assess potential risks and exposures. These systems can adjust trading strategies in real time, managing risk according to the level of volatility, market liquidity, or other financial indicators, thus protecting investment capital more effectively.
4. Automated Trading Strategies
AI develops and tests automated trading strategies by simulating different market scenarios and optimizing them for higher returns and reduced risks.
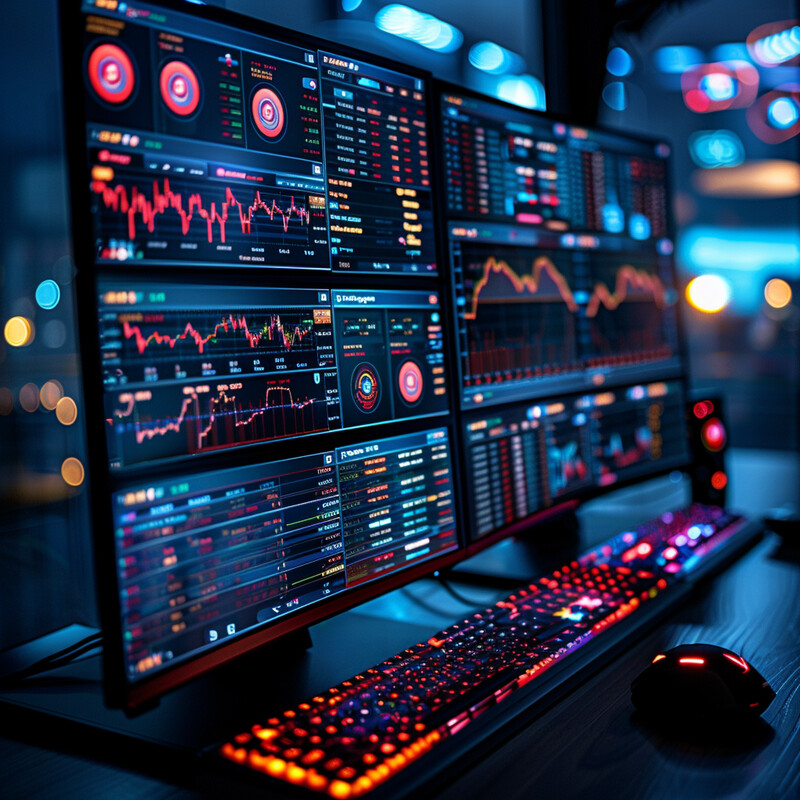
AI can automatically develop and backtest trading strategies using historical data and simulated market conditions. This allows for the optimization of strategies according to various performance metrics like maximum drawdown, Sharpe ratio, or return on investment. AI continuously refines these strategies based on new data, improving their effectiveness and adaptability to new market conditions.
5. Sentiment Analysis
AI processes qualitative data like news articles and social media posts to gauge market sentiment and predict its impact on financial markets, guiding investment decisions.
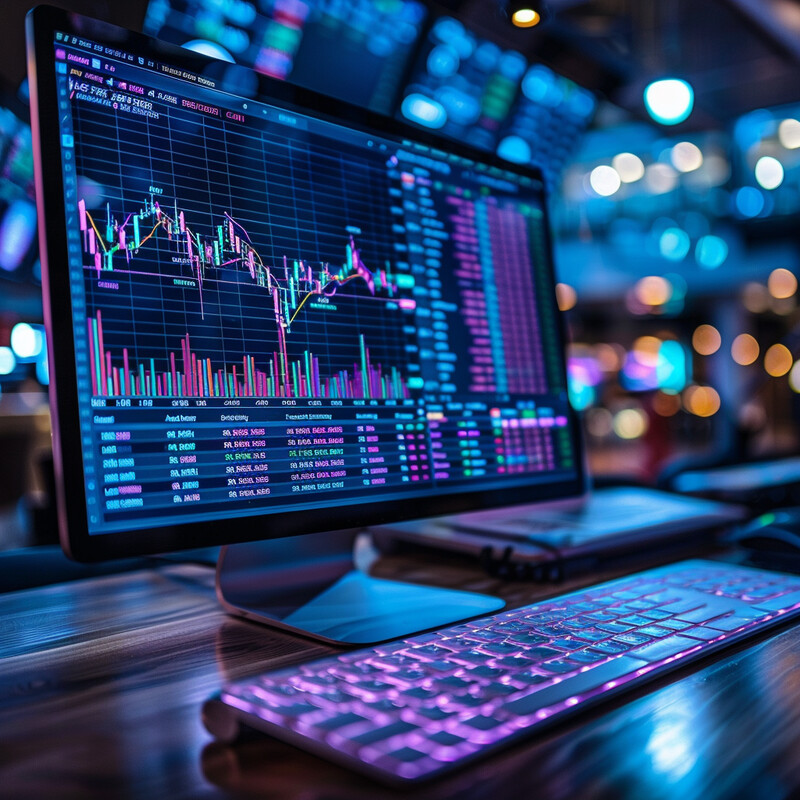
AI processes vast amounts of qualitative data from news articles, blog posts, social media, and other digital platforms to perform sentiment analysis. By understanding public sentiment towards particular markets, companies, or products, AI can predict how these sentiments might influence market movements, helping traders to anticipate significant price changes driven by public opinion.
6. Fraud Detection
AI enhances the detection of unusual trading patterns and potential fraud within financial markets, ensuring compliance and security.
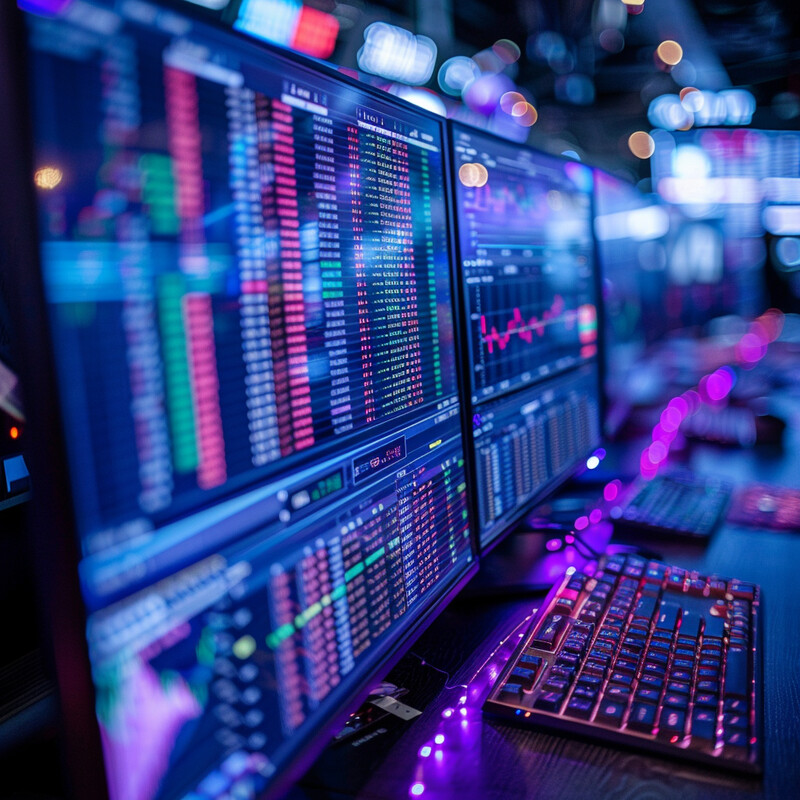
AI algorithms are employed to monitor trading patterns and flag activities that deviate from the norm which could indicate fraudulent behavior or market manipulation. This capability is crucial for maintaining market integrity and compliance with regulatory standards, as AI can quickly identify suspicious activities and alert human supervisors.
7. Portfolio Management
AI algorithms assist in creating and managing investment portfolios, balancing asset allocations based on changing market conditions and individual investor goals.
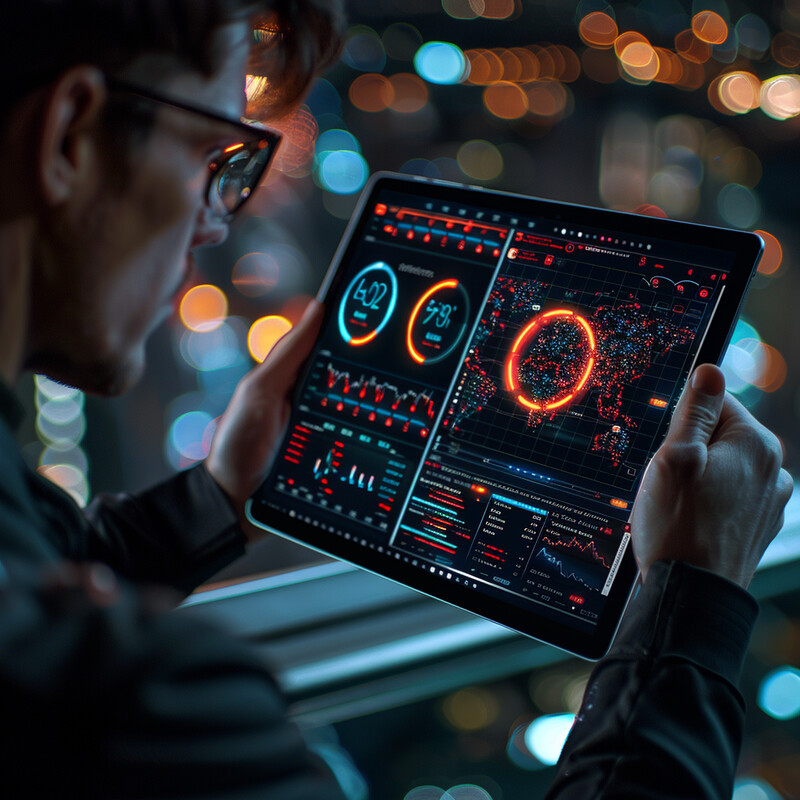
AI aids in the management of investment portfolios by continuously analyzing market conditions and optimizing asset allocation to achieve the best possible performance based on the investor's risk tolerance and investment goals. AI can make real-time adjustments to the portfolio to respond to market changes or to take advantage of new investment opportunities.
8. Optimization of Execution
AI optimizes trade execution by determining the most favorable times to buy or sell securities, thus minimizing slippage and improving price execution.
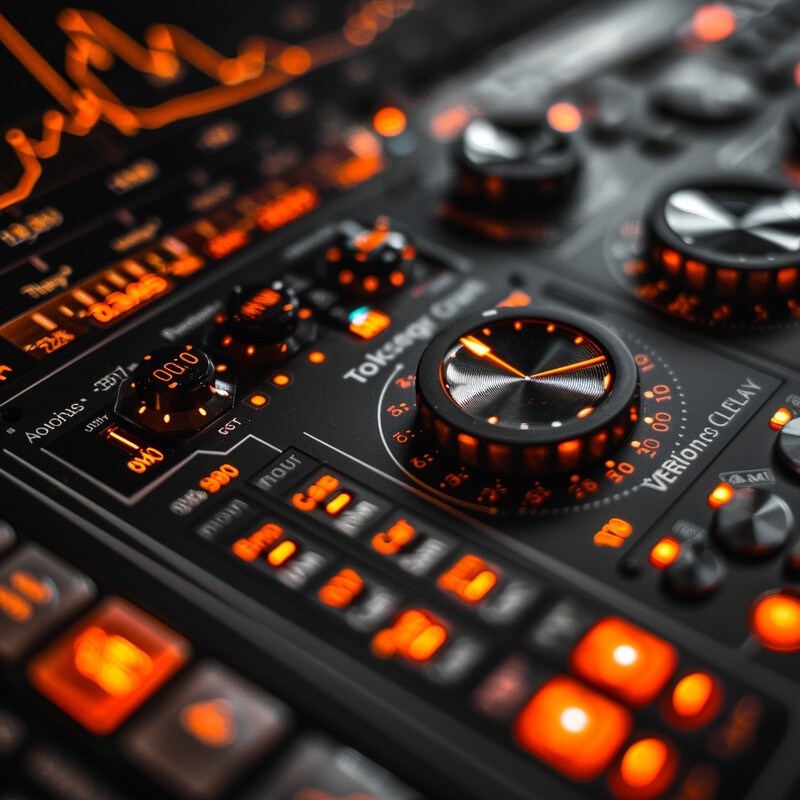
AI optimizes the timing and pricing of trades to ensure the best possible execution. By analyzing market liquidity, price trends, and other order book dynamics, AI determines the optimal moments to place buy or sell orders, thereby reducing the cost of trading and minimizing the market impact of large orders.
9. Real-time Analytics
AI provides real-time analytics and dashboards that help traders understand market conditions instantly and react accordingly.
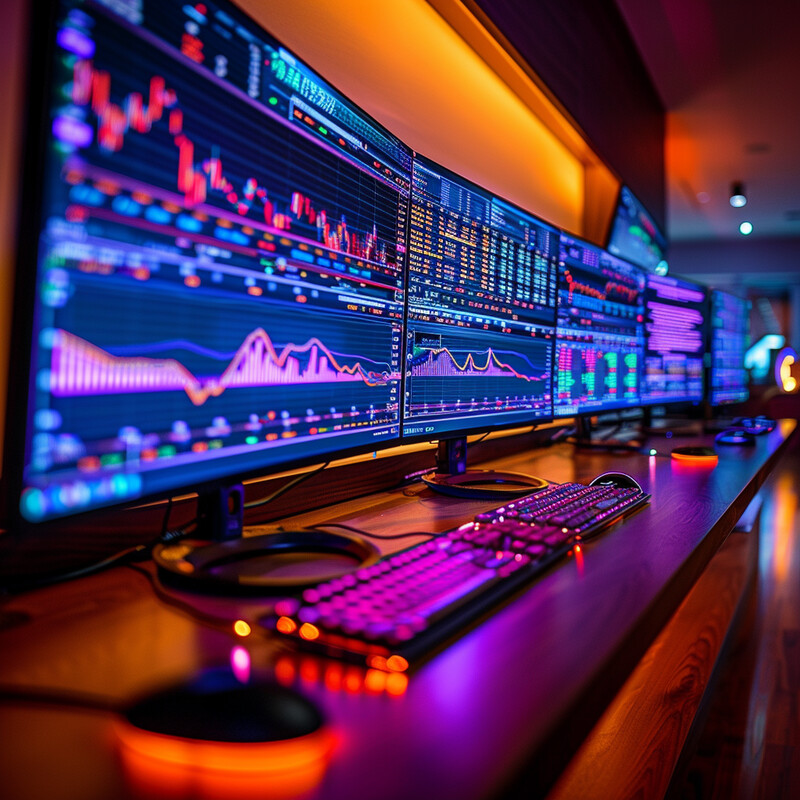
AI provides traders with real-time analytics and visualizations of market data, enabling them to make quicker and more informed decisions. This includes the ability to track market developments as they happen, analyze trading performance in real time, and adjust strategies on the fly.
10. Predictive Maintenance of Trading Systems
AI monitors the health of trading systems and infrastructure, predicting issues before they cause system downtime or affect trading activities.
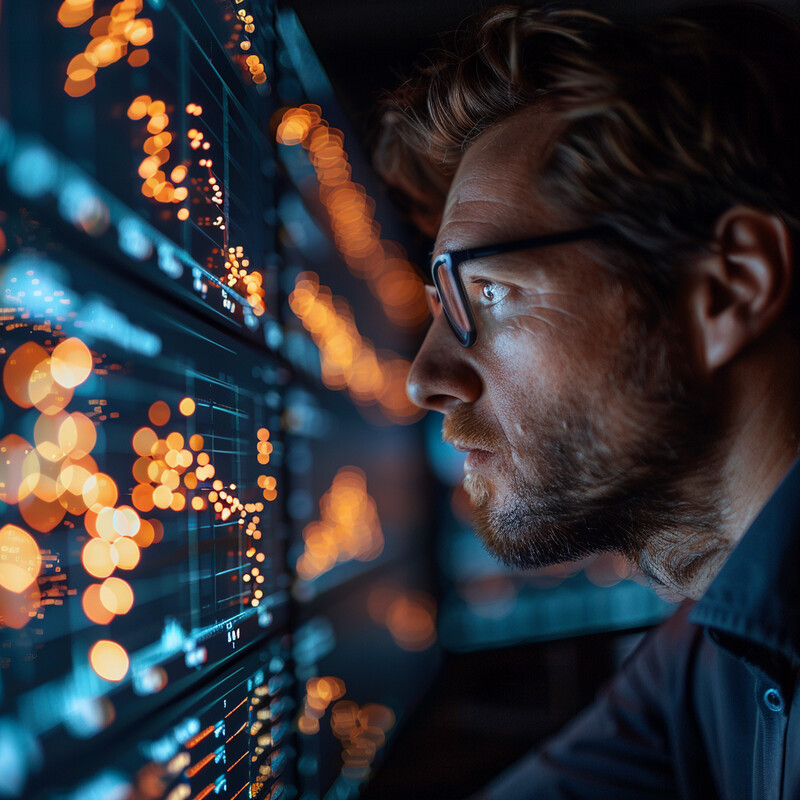
AI monitors the operational health of trading systems to predict and prevent potential failures. By analyzing system performance data, AI can identify patterns or anomalies that may precede a system breakdown, allowing for preemptive maintenance and reducing downtime in trading activities.