1. Smart Grid Management
AI optimizes the operation and energy flow of smart grids, enabling dynamic power adjustments based on real-time demand and supply conditions.
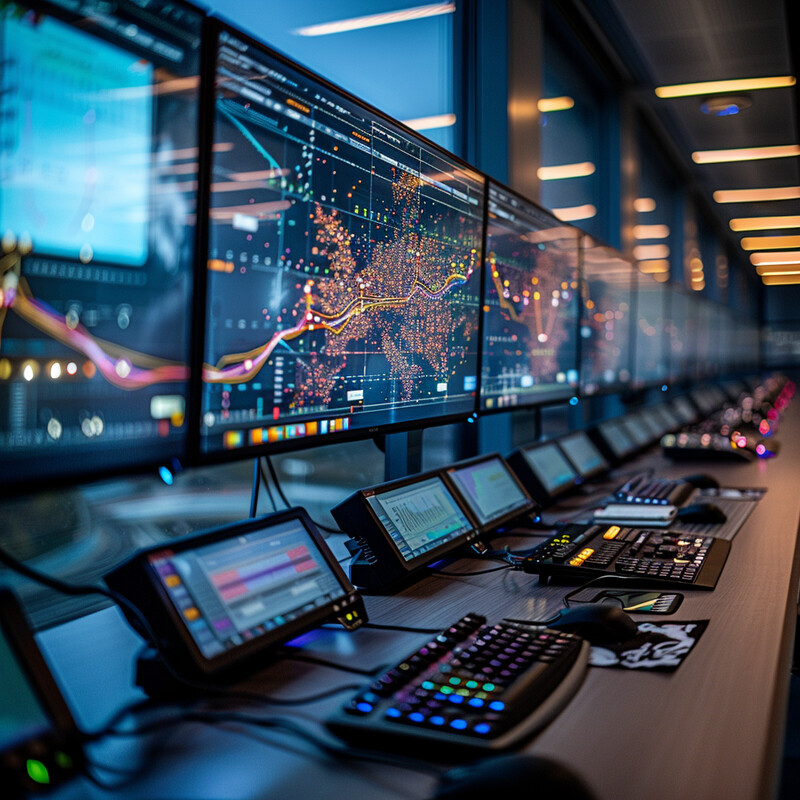
AI optimizes the operation of smart grids by dynamically managing energy flow based on real-time data on electricity demand, supply conditions, and grid stability. Using advanced algorithms, AI can predict peak load times and adjust the distribution of power to maintain grid efficiency and prevent outages. This dynamic management helps balance load, integrate renewable energy sources effectively, and reduce energy wastage.
2. Predictive Maintenance
AI predicts when equipment and systems require maintenance before they fail or become less efficient, thus preventing energy wastage and ensuring optimal performance.
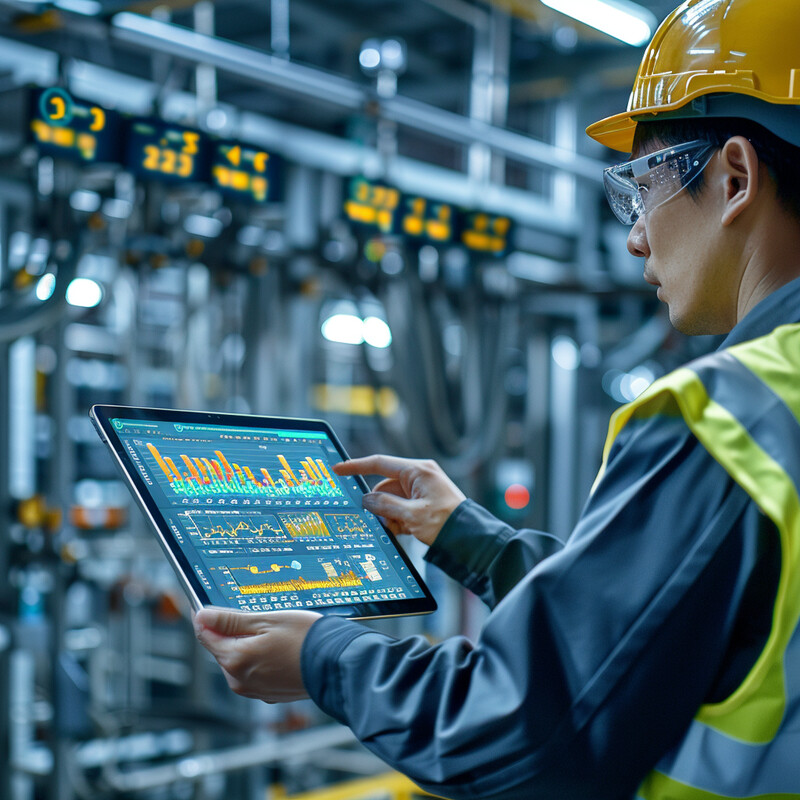
AI-driven predictive maintenance uses data from sensors embedded in equipment to monitor their condition continuously. By analyzing patterns and predicting potential failures before they occur, AI ensures that machinery and systems are maintained optimally. This not only prevents unexpected energy losses due to equipment inefficiency or downtime but also extends the lifespan of the assets.
3. HVAC Optimization
AI algorithms manage heating, ventilation, and air conditioning systems to optimize energy use while maintaining comfort levels based on occupancy and weather conditions.
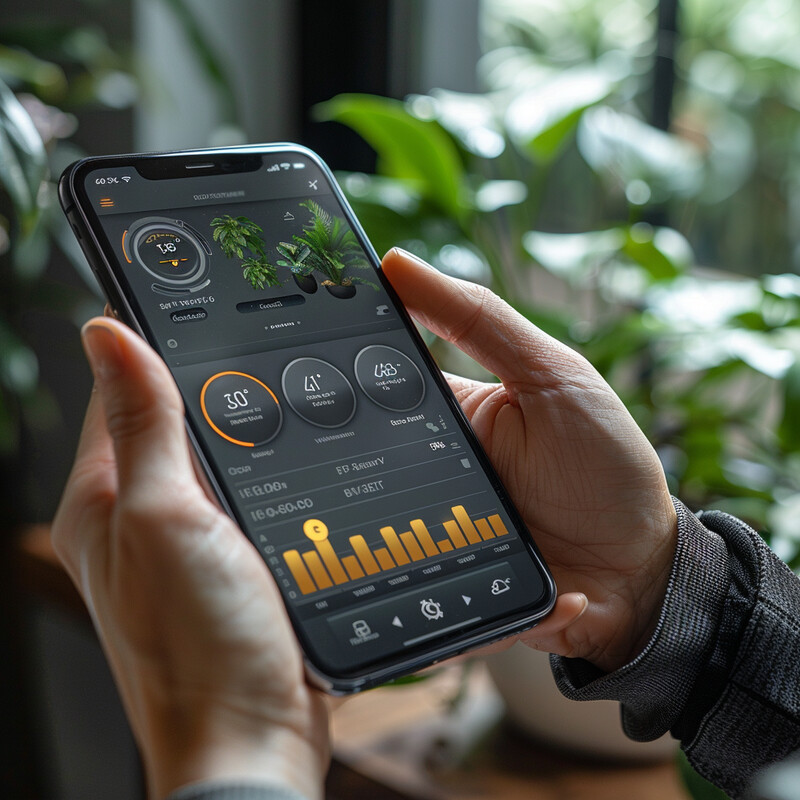
AI algorithms control HVAC systems to optimize energy usage without compromising comfort. By considering factors such as external weather conditions, time of day, and occupancy levels within buildings, AI adjusts temperatures and ventilation rates precisely. This targeted control significantly reduces energy consumption while maintaining an ideal indoor environment.
4. Energy Demand Forecasting
AI uses historical data and predictive analytics to forecast future energy demands, allowing utilities and businesses to adjust their energy production and consumption strategies accordingly.
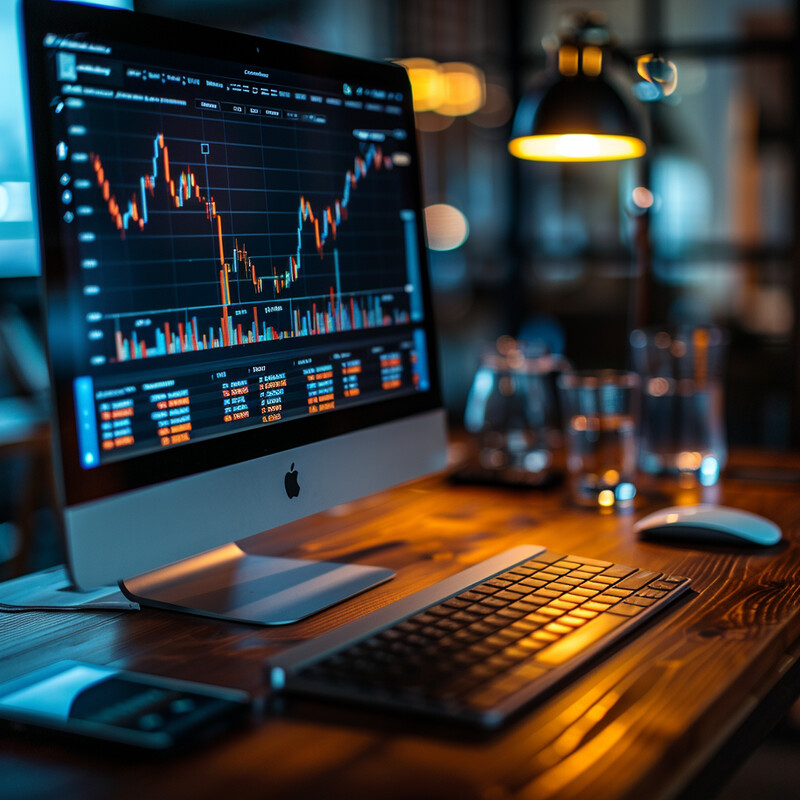
AI employs machine learning techniques to analyze historical energy usage data alongside predictive analytics to forecast future energy demands accurately. This allows energy providers and businesses to plan and adjust their energy generation and consumption strategies more effectively, ensuring they can meet demand without excessive energy production, which can be costly and inefficient.
5. Renewable Energy Integration
AI helps in managing the integration of renewable energy sources like solar and wind into the existing grid, optimizing when and how much renewable energy to use based on availability and grid demand.
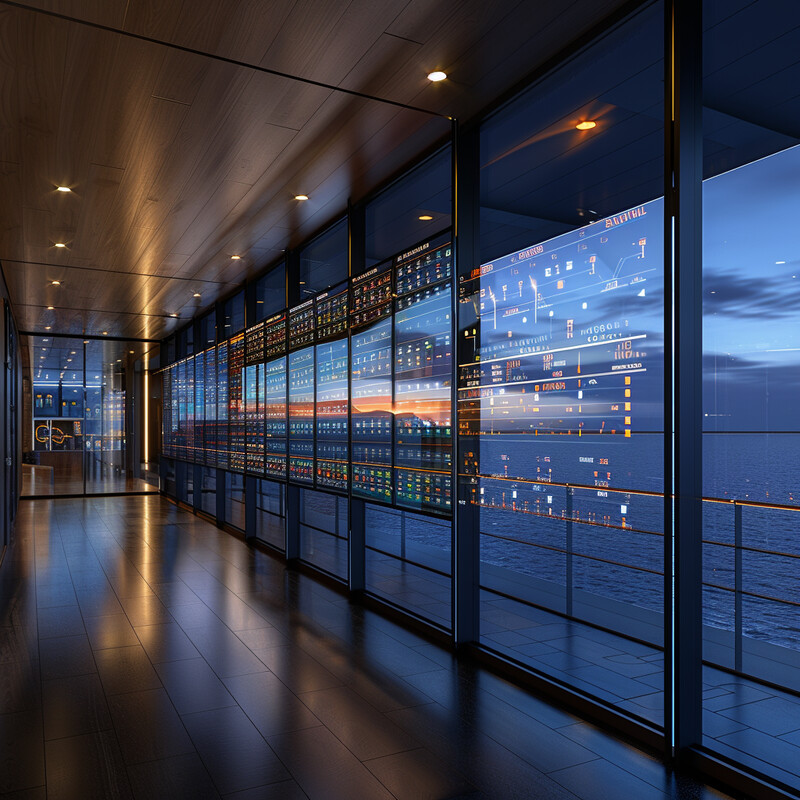
AI enhances the integration of renewable energy sources, such as solar and wind, into the power grid. It predicts the variability in renewable energy production and adjusts grid operations accordingly. AI also optimizes the storage and release of energy from batteries, ensuring that excess energy generated from renewables is stored and used efficiently, minimizing reliance on less sustainable energy sources.
6. Building Energy Management
AI systems analyze data from building sensors and devices to optimize energy usage across lighting, heating, and cooling systems, reducing overall building energy consumption.
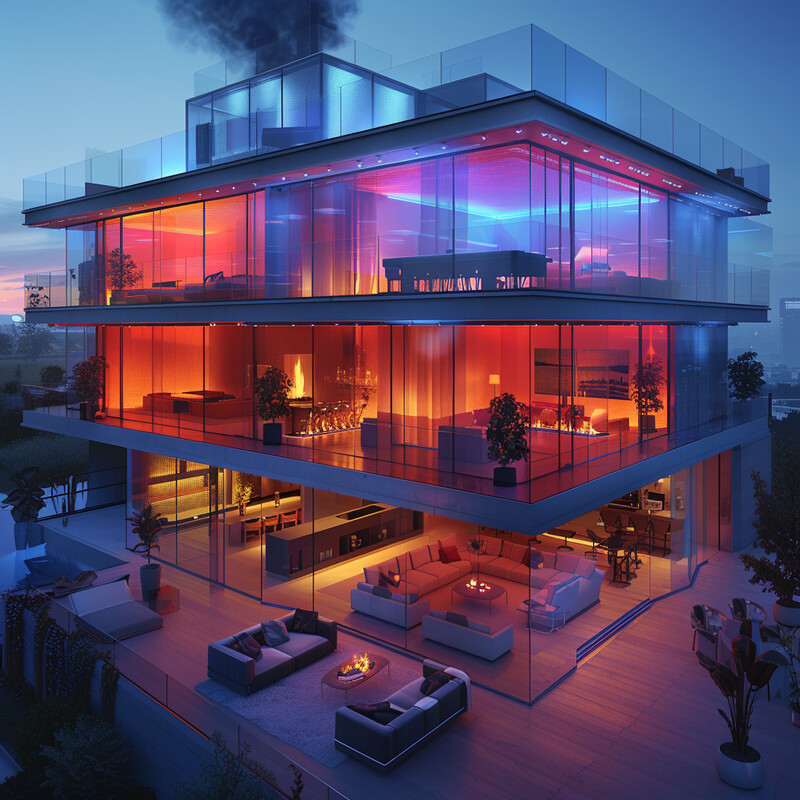
AI systems in building management use data from various sensors and devices to optimize the energy usage of lighting, heating, and air conditioning based on actual building use. For instance, AI can automatically dim lights or reduce heating in unoccupied areas, leading to significant energy savings across commercial and residential buildings.
7. Industrial Automation
AI automates and optimizes manufacturing processes to minimize energy use, making production lines more efficient and less energy-intensive.
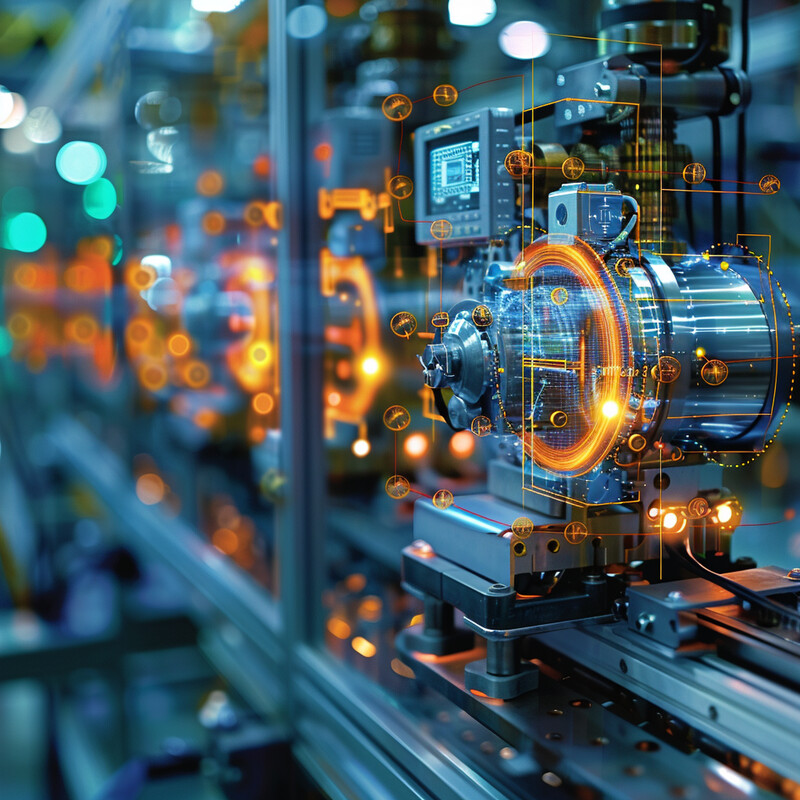
In industrial settings, AI optimizes manufacturing processes to consume less energy. By automating and refining production lines, AI ensures operations are not only faster and less labor-intensive but also more energy-efficient. This application of AI reduces the overall energy footprint of manufacturing activities, enhancing both economic and environmental outcomes.
8. Transportation and Fleet Management
AI optimizes routes and schedules for logistics and transportation systems, reducing fuel consumption and increasing operational efficiency.
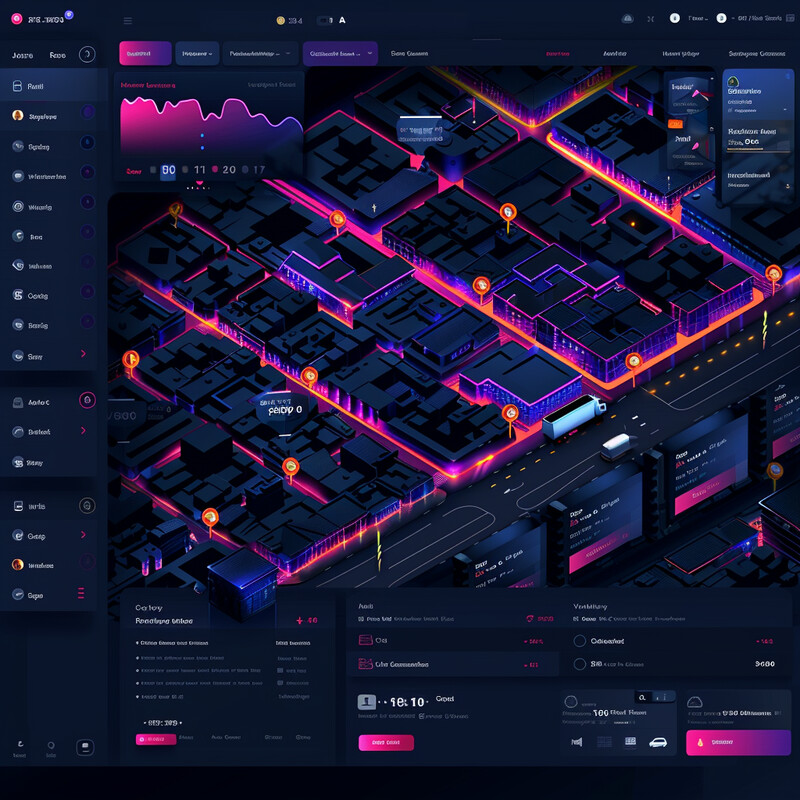
AI optimizes routes and schedules for transportation and logistics, reducing fuel consumption and improving delivery times. By analyzing traffic patterns, weather conditions, and vehicle performance data, AI determines the most efficient routes and anticipates possible delays, thereby optimizing operational efficiency and reducing energy costs.
9. Real-Time Energy Monitoring
AI tools provide real-time insights into energy consumption patterns, enabling immediate adjustments and proactive energy-saving measures.
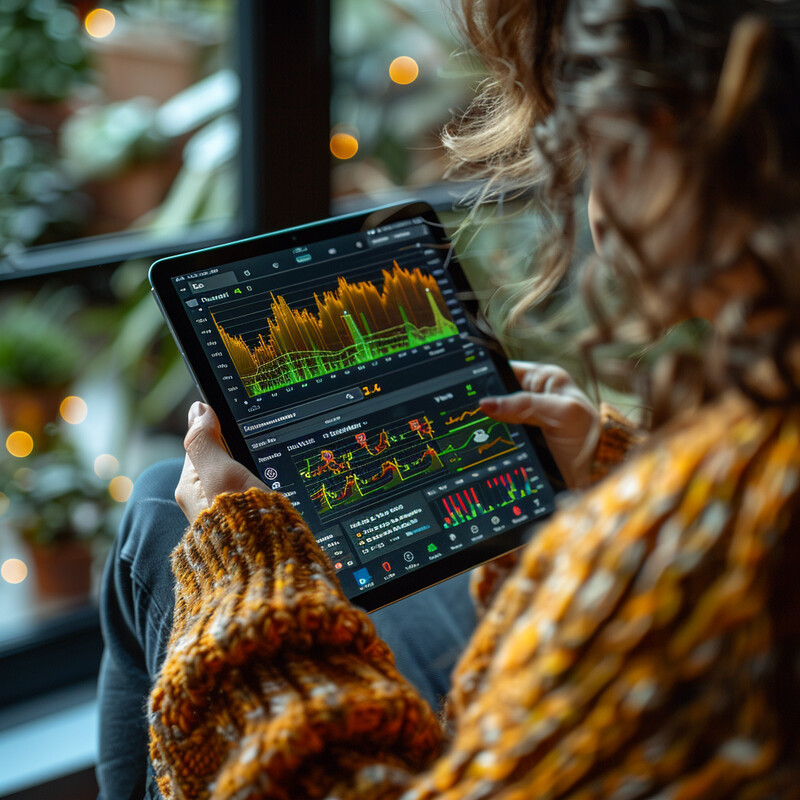
AI tools provide detailed real-time monitoring of energy usage, enabling businesses and households to understand and manage their energy consumption effectively. These AI systems can identify areas of high energy use and suggest immediate adjustments, such as shutting down unnecessary appliances, which can lead to significant energy savings.
10. Behavioral Energy Efficiency
AI analyzes user behavior and provides feedback and recommendations to encourage energy-saving practices, helping individuals and organizations reduce their energy footprint.
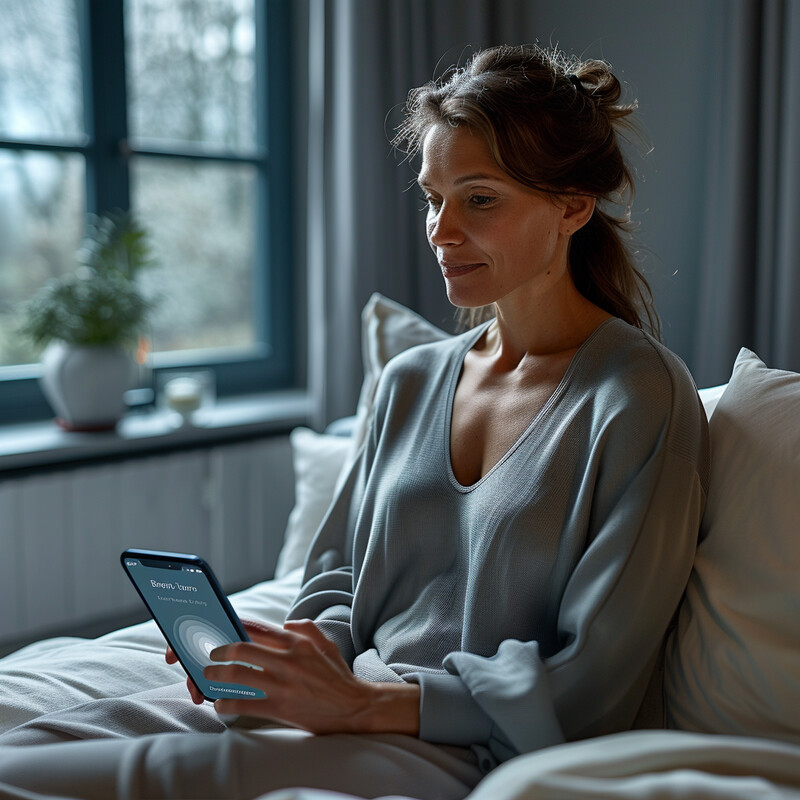
AI analyzes energy usage patterns and user behaviors to provide personalized feedback and recommendations for energy conservation. By educating users about their energy consumption habits and suggesting practical ways to reduce energy use, AI encourages more sustainable behavior, which can lead to considerable energy and cost savings over time.