1. Battery Management
AI optimizes battery performance by analyzing usage patterns and environmental factors, adjusting charging rates and discharging strategies to maximize battery life and efficiency.
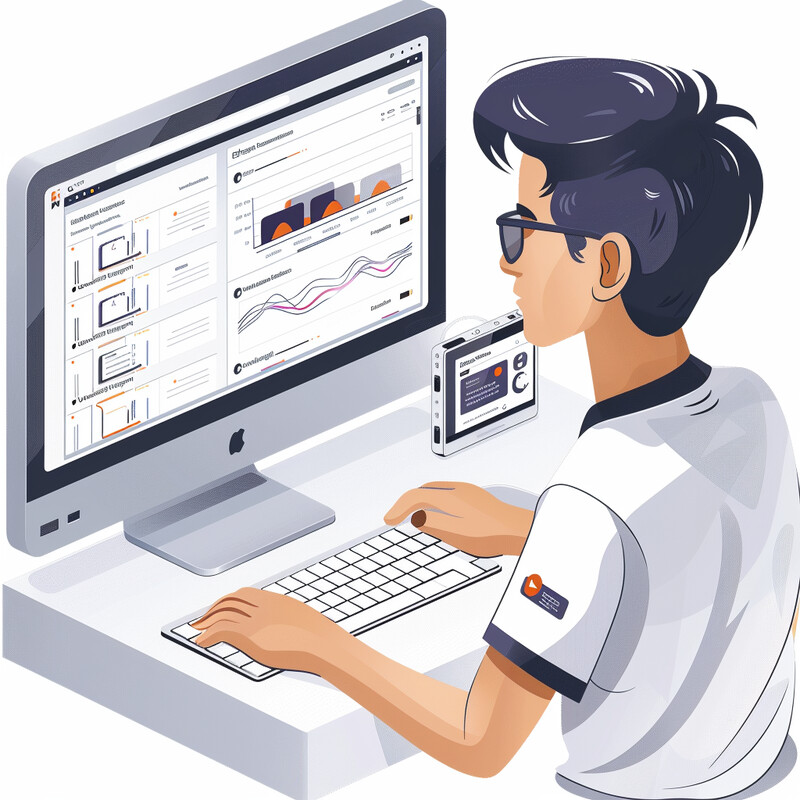
AI optimizes battery performance in electric vehicles by continuously analyzing data from usage patterns, charging habits, and environmental conditions. It adjusts charging rates and discharging strategies to extend battery life and maintain optimal efficiency. By predicting and managing the health and capacity of the battery over time, AI ensures maximum performance and longevity, reducing the need for frequent replacements and enhancing overall vehicle sustainability.
2. Range Prediction
AI improves range accuracy by analyzing driving habits, route topography, and current traffic conditions to provide more accurate estimates of remaining range, reducing range anxiety for drivers.
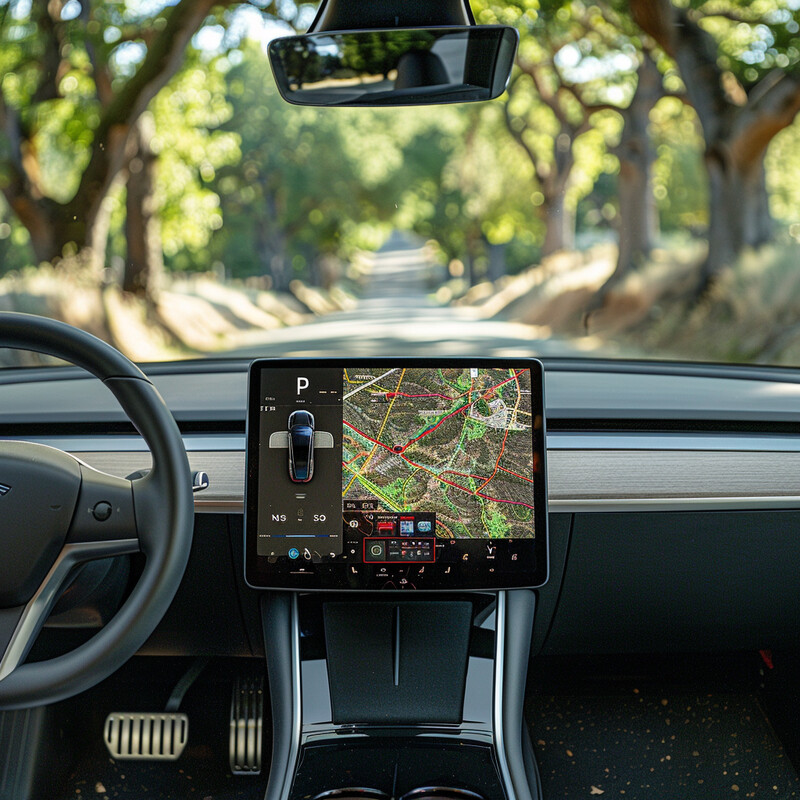
AI significantly enhances the accuracy of range estimations in electric vehicles by considering factors such as driving habits, route topography, current traffic conditions, and weather. This allows for more precise predictions of how far the vehicle can travel on a single charge, helping to alleviate range anxiety among drivers and making route planning more reliable.
3. Energy Consumption Optimization
AI algorithms dynamically adjust energy consumption based on driving conditions, vehicle load, and battery status to improve overall energy efficiency.
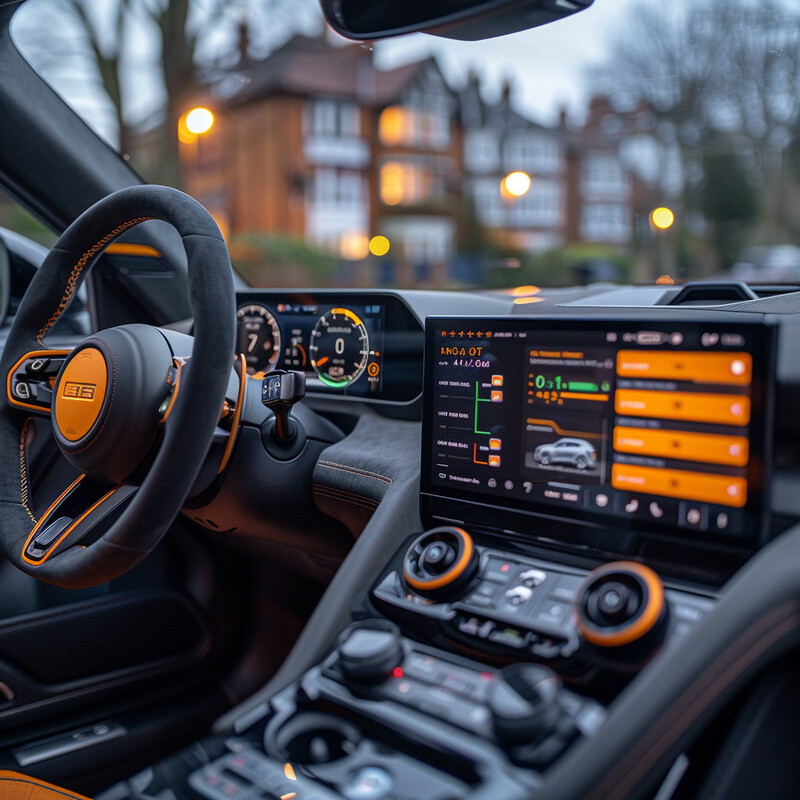
AI algorithms dynamically manage the energy consumption of electric vehicles based on real-time data such as driving conditions, vehicle load, and battery status. By optimizing energy use during different phases of a journey, AI improves the vehicle's overall energy efficiency, extending the range and reducing the environmental impact.
4. Autonomous Driving Features
AI powers advanced driver-assistance systems (ADAS) and fully autonomous driving capabilities, improving safety and allowing for more efficient route management and traffic navigation.
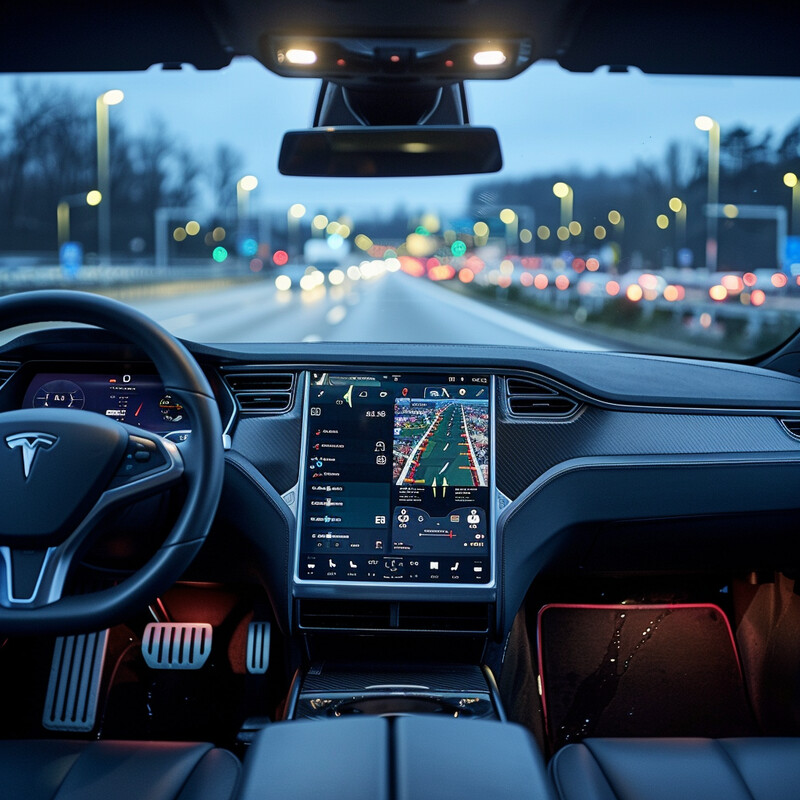
AI is at the core of developing and improving autonomous driving technologies in electric vehicles. It powers advanced driver-assistance systems (ADAS) that enhance vehicle safety through features like automatic braking, lane-keeping assist, and adaptive cruise control. Furthermore, AI enables more complex autonomous driving capabilities, which can lead to more efficient traffic management and reduced human driving errors.
5. Charging Station Management
AI helps manage the demand and distribution of power in charging stations, optimizing charging schedules and managing peak load to improve the availability of charging infrastructure.
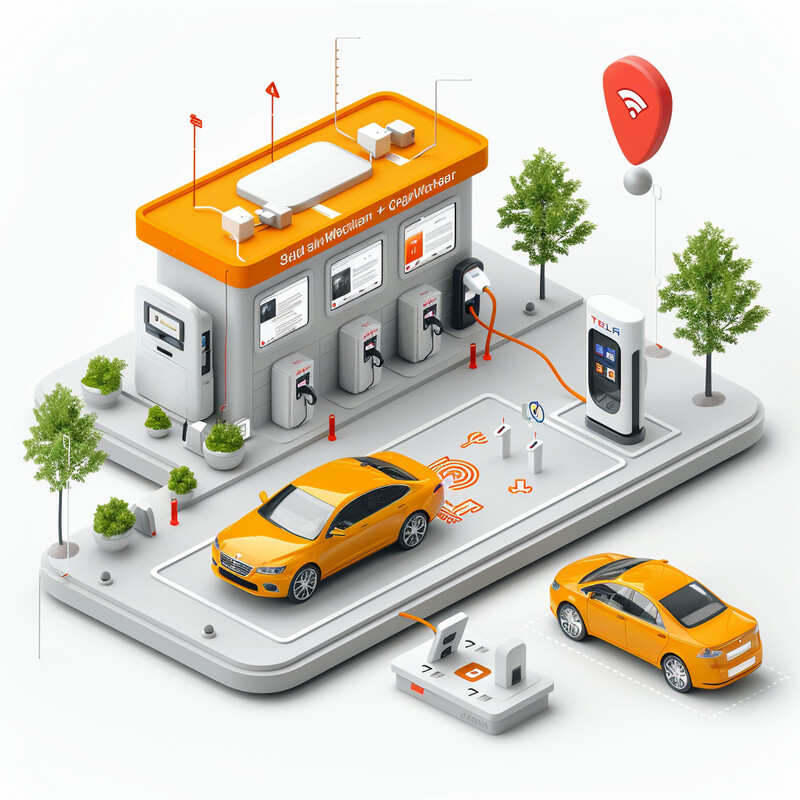
AI helps manage the infrastructure for electric vehicle charging stations by optimizing the distribution of power and managing the scheduling of charging sessions. This is particularly important during peak times to avoid overloading the power grid. AI can also predict peak demand times and adjust pricing or availability to balance supply and demand, thereby improving the charging experience for users.
6. Predictive Maintenance
AI uses data from vehicle sensors to predict when maintenance is needed, preventing breakdowns and reducing maintenance costs by addressing issues before they become more severe.
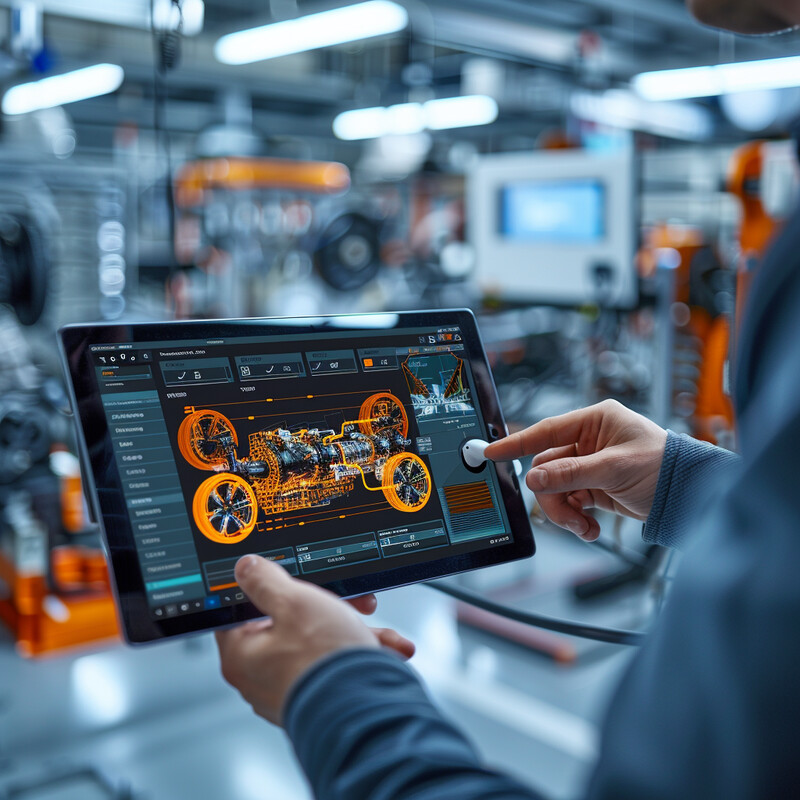
AI uses data from various sensors within the vehicle to predict maintenance needs before issues arise. This proactive approach to maintenance helps prevent unexpected breakdowns and reduces the overall cost of vehicle upkeep by addressing potential problems early, often before they require more significant and costly repairs.
7. Thermal Management
AI monitors and controls the temperature of the battery and other critical components, enhancing performance and safety under various operating conditions.
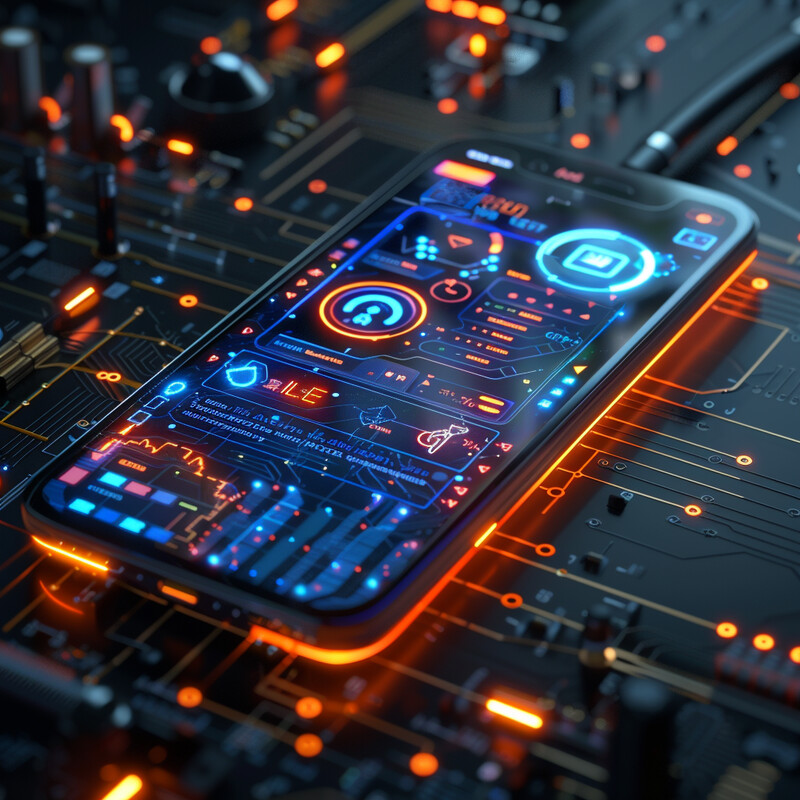
Effective thermal management is crucial for maintaining battery efficiency and longevity. AI monitors and adjusts the temperature of the battery and other critical components of electric vehicles, ensuring optimal performance across different environmental conditions. This management helps prevent overheating and ensures the vehicle operates safely under a variety of usage scenarios.
8. User Experience Customization
AI tailors vehicle settings such as climate control, audio preferences, and driving modes to individual user profiles, enhancing comfort and driving pleasure.
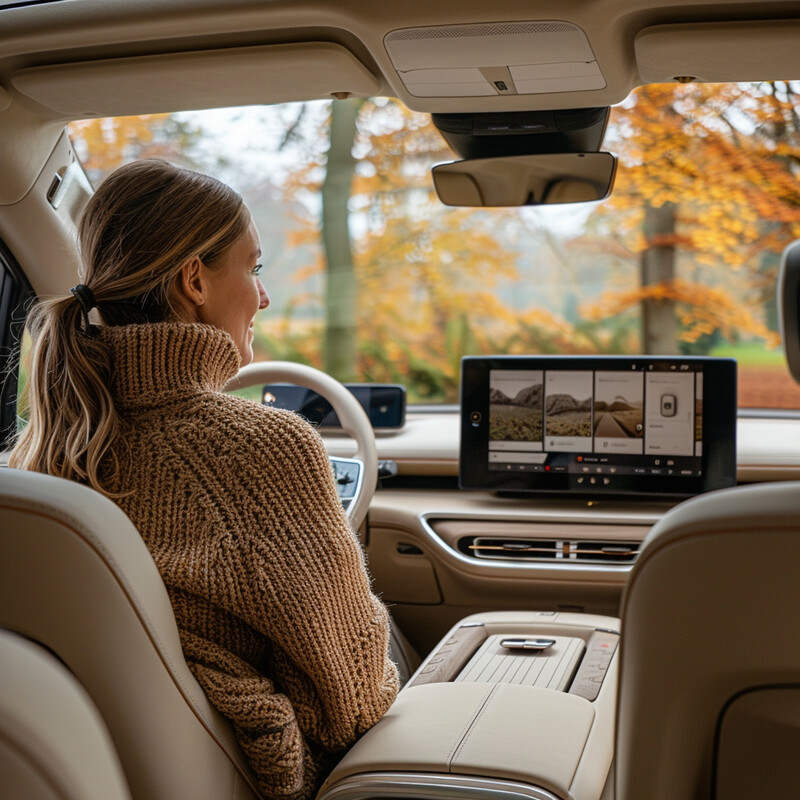
AI enhances the user experience in electric vehicles by learning individual preferences and customizing various aspects of the vehicle’s environment. This includes automatic adjustments to climate control, seat positioning, multimedia settings, and driving modes tailored to each user’s profile, enhancing comfort and driving pleasure.
9. Vehicle-to-Grid (V2G) Integration
AI manages the interaction between electric vehicles and the power grid, allowing vehicles to return energy to the grid during peak demand periods, which can help stabilize the grid and provide additional revenue streams for vehicle owners.
-integration-1.jpg)
AI plays a key role in integrating electric vehicles with the energy grid through vehicle-to-grid (V2G) technologies. It manages how and when vehicles can return energy to the grid, especially during periods of high demand. This not only helps stabilize the grid but also allows vehicle owners to potentially earn revenue by providing power back to the grid.
10. Recycling and Life Cycle Management
AI analyzes battery health and predicts end-of-life timing, facilitating the efficient recycling of battery components and helping manufacturers design more sustainable vehicles.
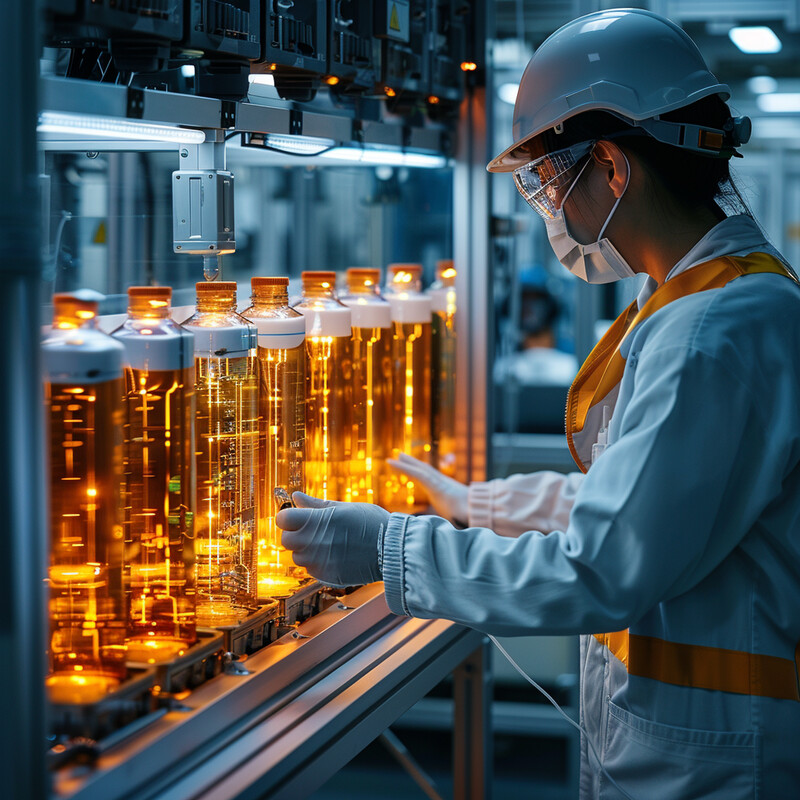
At the end of their life, electric vehicle batteries pose significant environmental challenges. AI assists in predicting the end-of-life for vehicle batteries and facilitates their recycling process. By analyzing battery health data, AI can help in efficiently recycling materials and redesigning future batteries to be more sustainable, closing the loop in the battery lifecycle.