1. Personalized Product Recommendations
Personalized product recommendation engines use AI algorithms to suggest items tailored to each shopper’s preferences and behavior. By analyzing browsing history, past purchases, and similar users’ data, these systems present relevant products that a customer is more likely to buy. This individualized approach enhances the user experience and can significantly boost engagement, conversion rates, and sales in e-commerce. Most major online retailers (from Amazon to streaming services) rely on recommendation models to drive a large share of their revenue. As personalization becomes standard, businesses focus on fine-tuning algorithms and data quality to maintain effectiveness while respecting customer privacy.
AI analyzes individual browsing and purchase history to tailor product suggestions to each customer's preferences, increasing the likelihood of purchases.
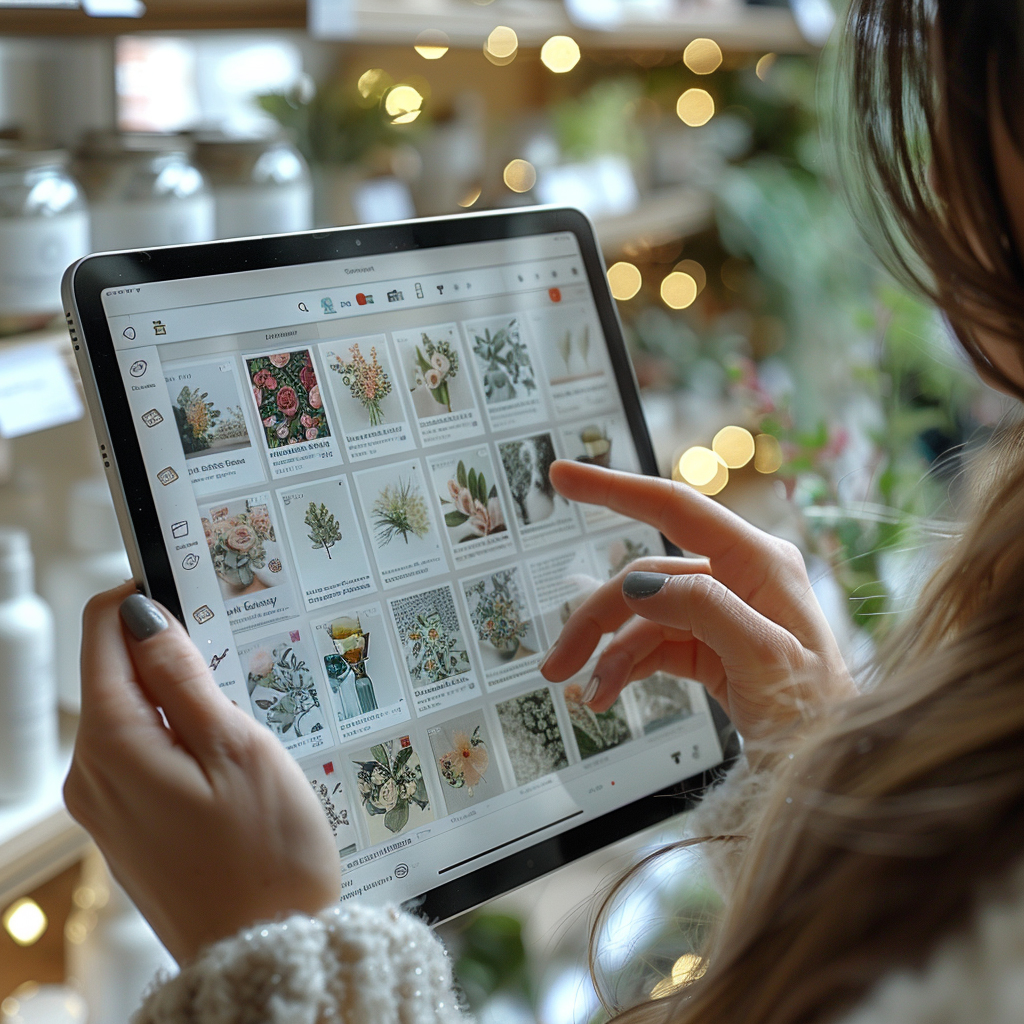
Personalized product recommendations can account for roughly one-third of an e-commerce site’s revenue – a 2023 study found they contribute an average of 31% of online retail revenues.
AI-driven algorithms analyze past browsing and purchasing behaviors to tailor product recommendations for each user. By understanding individual preferences and habits, AI can suggest items that the customer is more likely to be interested in, thus enhancing the shopping experience and increasing sales. This personalization is not just limited to past purchases but also includes items viewed, search history, and even abandoned cart contents.
2. Predictive Analytics
Predictive analytics involves using historical and real-time data along with machine learning to forecast future outcomes in e-commerce. Retailers employ predictive models for demand forecasting, trend anticipation, and customer behavior predictions. This proactive approach helps optimize inventory (stocking the right products in the right quantities), personalize marketing (predicting what a customer might buy next), and improve operational efficiency. In recent years, many companies have increased investments in predictive analytics to gain a competitive edge, as better forecasts can lead to higher sales and lower costs. By transitioning from reactive to data-driven proactive strategies, e-commerce businesses can make informed decisions that improve profitability and customer satisfaction.
AI uses predictive analytics to forecast trends and user behavior, allowing businesses to proactively offer products and deals that are more likely to interest specific customers.
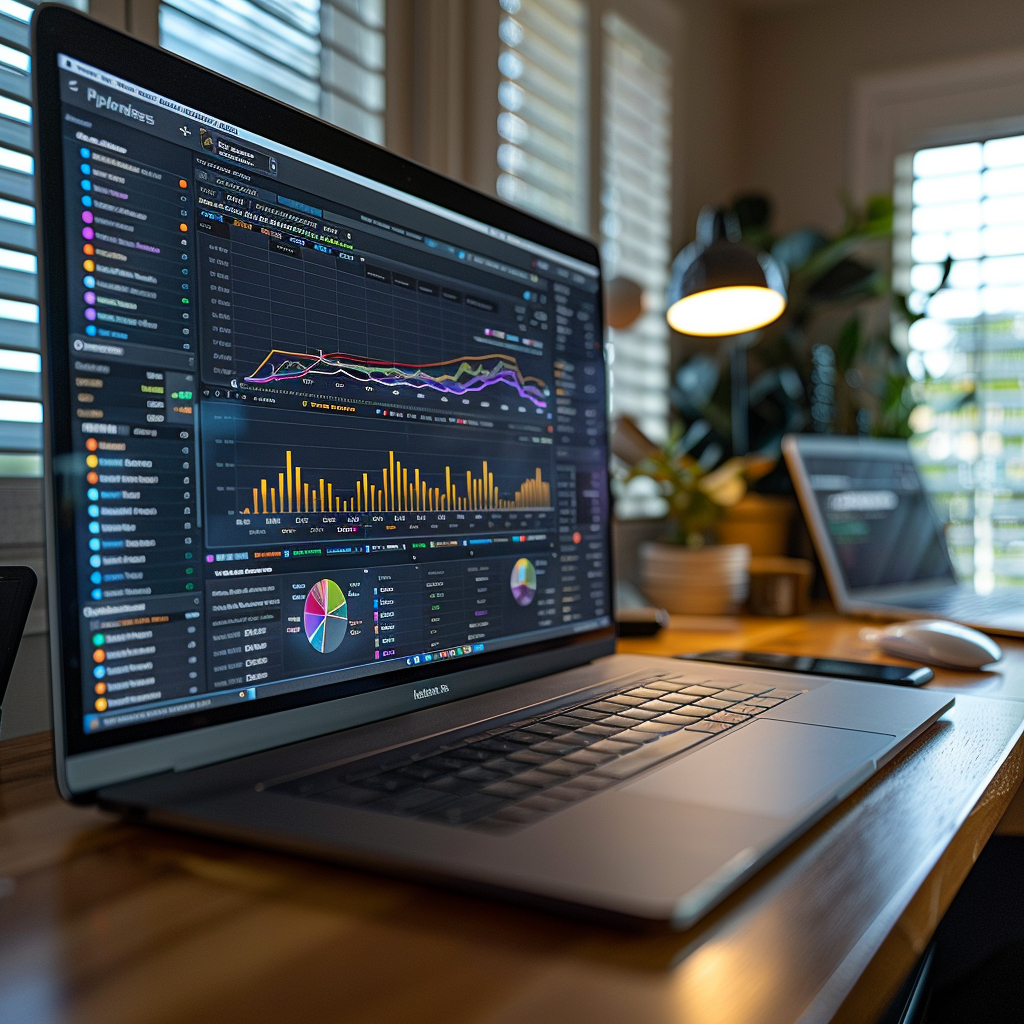
By late 2023, over 60% of retail executives reported plans to increase spending on predictive analytics and AI, a jump from about 40% in 2021.
Predictive analytics powered by AI forecasts future consumer purchasing trends and behaviors based on accumulated data. This allows e-commerce platforms to anticipate what products customers might want next, enabling businesses to market these products proactively. It also helps in planning marketing campaigns and inventory for upcoming trends.
3. Dynamic Pricing
Dynamic pricing uses AI to adjust product prices in real time based on demand, supply, customer profiles, and market conditions. In e-commerce, this means prices are not fixed but can fluctuate – for example, rising for high-demand items or dropping for excess stock. Retailers leveraging dynamic pricing algorithms can optimize revenue by charging the ideal price at any given moment, much like airlines and hotels have long done. AI-driven pricing models sift through large datasets (competitor prices, browsing behavior, time of day, etc.) and can update prices frequently to maximize sales or profit margins. While this strategy can significantly increase profitability and sell-through rates, it requires careful implementation to avoid alienating customers, who expect fair and consistent pricing.
AI enables dynamic pricing strategies where prices are adjusted in real-time based on demand, competition, and user interest, maximizing profit and market competitiveness.
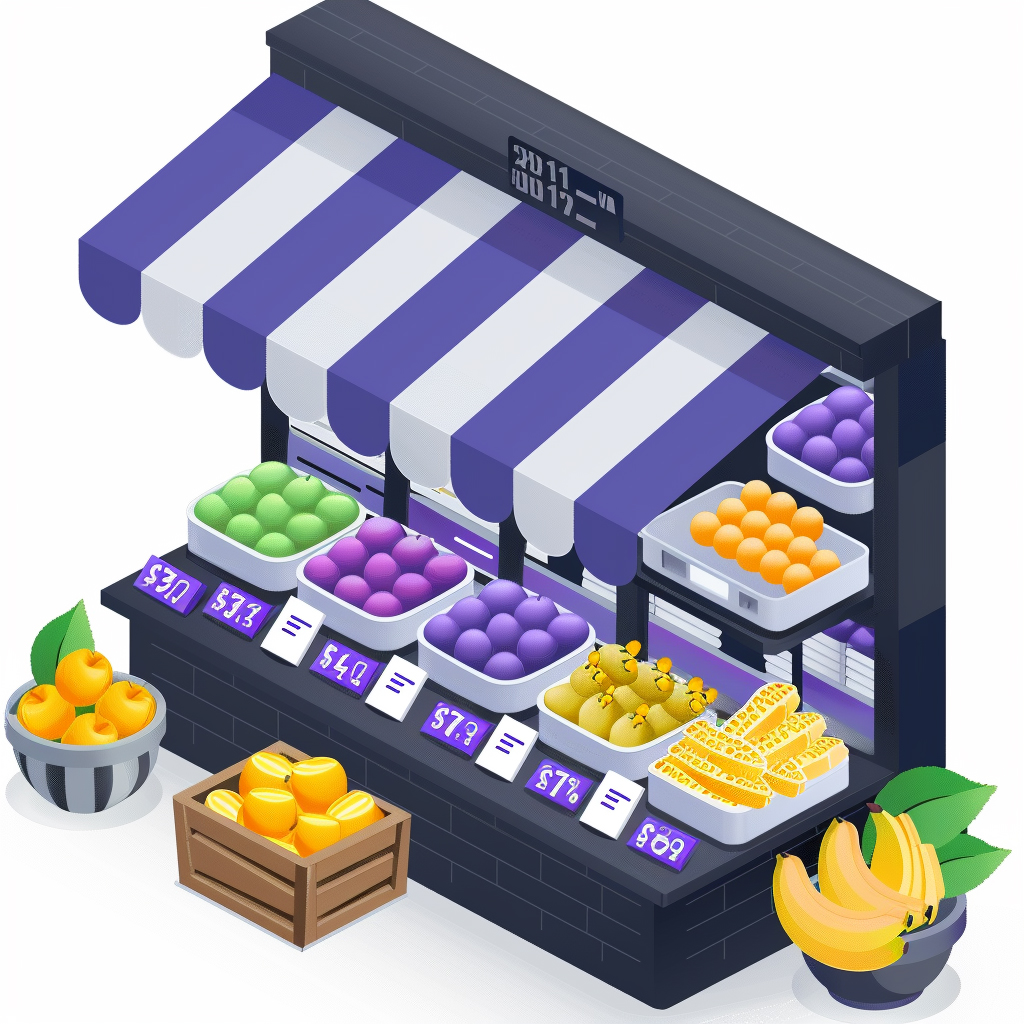
Implementing AI-powered dynamic pricing has been shown to substantially lift profitability – a recent McKinsey study indicates that using dynamic price optimization can increase retailers’ profits by about 10–20%.
AI implements dynamic pricing strategies where product prices are automatically adjusted based on various factors such as demand, customer behavior, inventory levels, and competitor pricing. This ensures that the pricing strategy remains optimal for both sales volume and revenue maximization, helping businesses stay competitive in fluctuating markets.
4. Customer Segmentation
Customer segmentation in e-commerce involves categorizing shoppers into distinct groups so that marketing and recommendations can be better tailored to each segment. AI has made this process far more granular and dynamic. Traditional segmentation might target broad categories (e.g. age or location), but AI-driven segmentation analyzes purchasing patterns, website interactions, and demographics to find micro-segments or “clusters” of customers with similar behaviors or needs. By understanding these nuanced segments (for example, bargain hunters vs. brand loyalists), companies can deliver more relevant product recommendations, personalized content, and targeted promotions to each group. This leads to more effective marketing campaigns, as messages resonate better with their intended audience, ultimately driving higher engagement and conversion rates.
AI segments customers into distinct groups based on their behavior and preferences, enabling more targeted and effective marketing strategies.
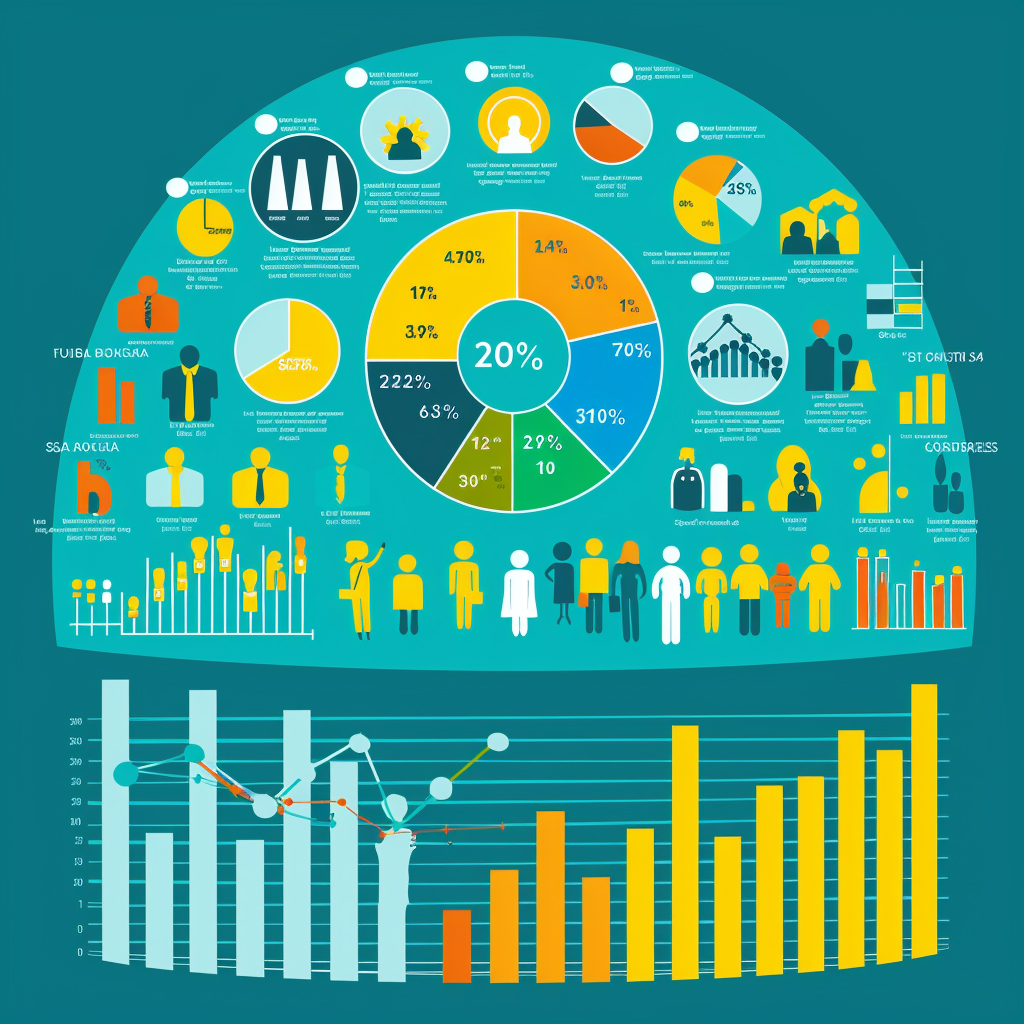
Retailers using AI for customer segmentation have seen significant gains in marketing effectiveness – AI-driven segmentation can yield up to a 20% increase in marketing ROI by enabling more accurate targeting and highly personalized campaigns.
AI classifies customers into precise segments based on their shopping patterns, demographic data, and preferences. This segmentation allows for more targeted marketing and personalized recommendations, ensuring that customers receive offers and promotions that are relevant to their interests and are more likely to engage them.
5. Visual Search and Recognition
Visual search allows customers to search for products using images rather than text. With AI image recognition, an e-commerce app or website can take a photo (or camera scan) of an item and find similar products in its catalog. This technology is especially popular in fashion and home décor – for example, a shopper can upload a picture of a dress or a chair they like, and the system will recommend similar items for sale. Tech giants and retailers have heavily invested in visual search and recognition, as consumers increasingly seek more intuitive, visually driven ways to discover products. The growth of smartphone cameras and apps like Pinterest Lens and Google Lens has accelerated this trend. As visual search improves, it complements traditional keyword search and can improve product discovery, engagement, and sales for retailers.
AI-powered visual search technology allows customers to upload images to find similar products, enhancing the shopping experience and facilitating easier product discovery.
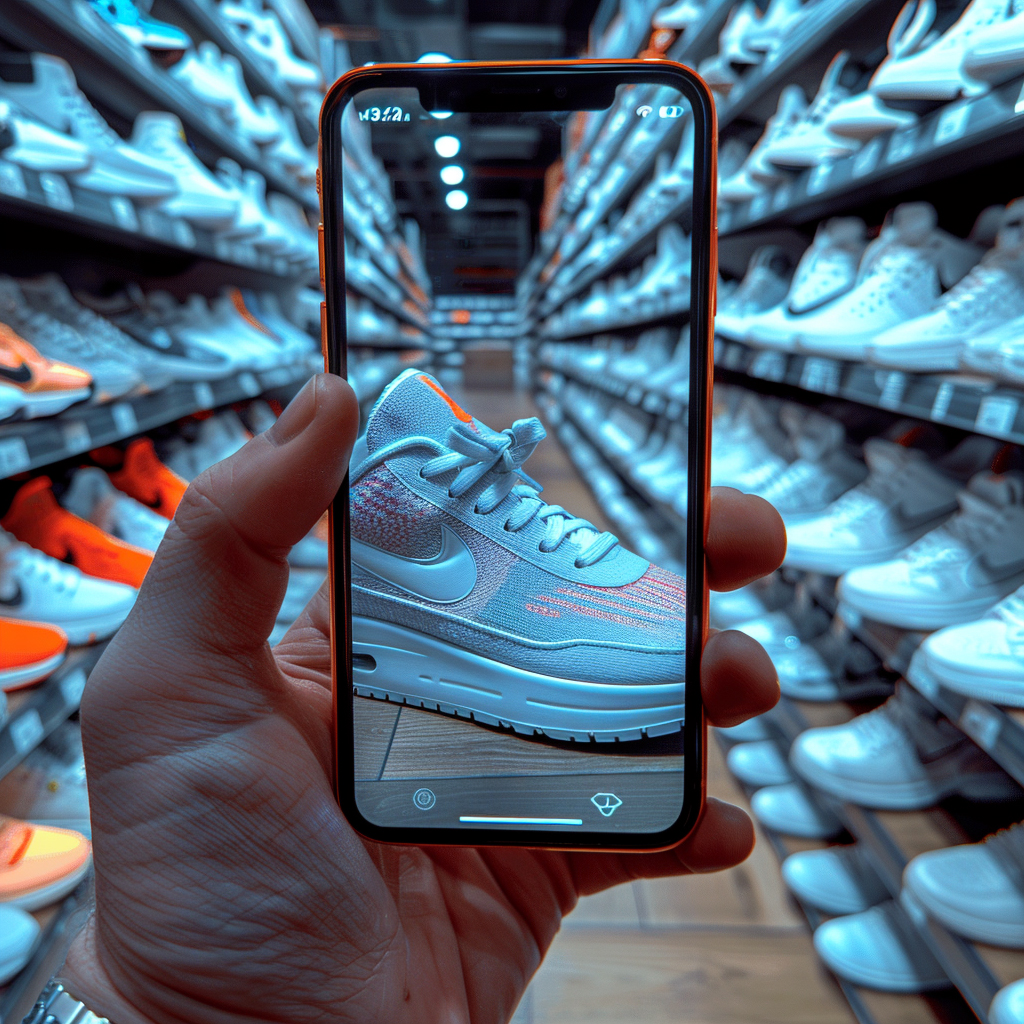
The use of visual search is surging – Google’s Lens tool now handles about 20 billion image searches per month, with roughly 4 billion of those searches related specifically to shopping.
Through AI-powered visual search capabilities, customers can upload images to search for similar products or styles. This technology analyzes the images to understand attributes like shape, color, and brand, making it easier for shoppers to find and buy products that match their interests without knowing the exact terms to describe them.
6. Optimized Inventory Management
AI-driven inventory management helps e-commerce businesses maintain optimal stock levels by accurately forecasting demand and automating replenishment decisions. Machine learning models analyze sales history, seasonality, current trends, and even external factors (like weather or social media buzz) to predict how much of each product will be needed and when. By anticipating demand, retailers can reduce stockouts (running out of an item) and overstock (excess unsold inventory). This optimization leads to cost savings (less capital tied up in inventory and lower storage costs) and increased sales (products are in stock when customers want them). In practice, companies using AI for inventory management see smoother operations – fewer lost sales due to missing items and less waste from over-ordering – ultimately improving both customer satisfaction and the bottom line.
AI predicts demand for products, helping businesses optimize their inventory levels, reduce overstock, and avoid stockouts.
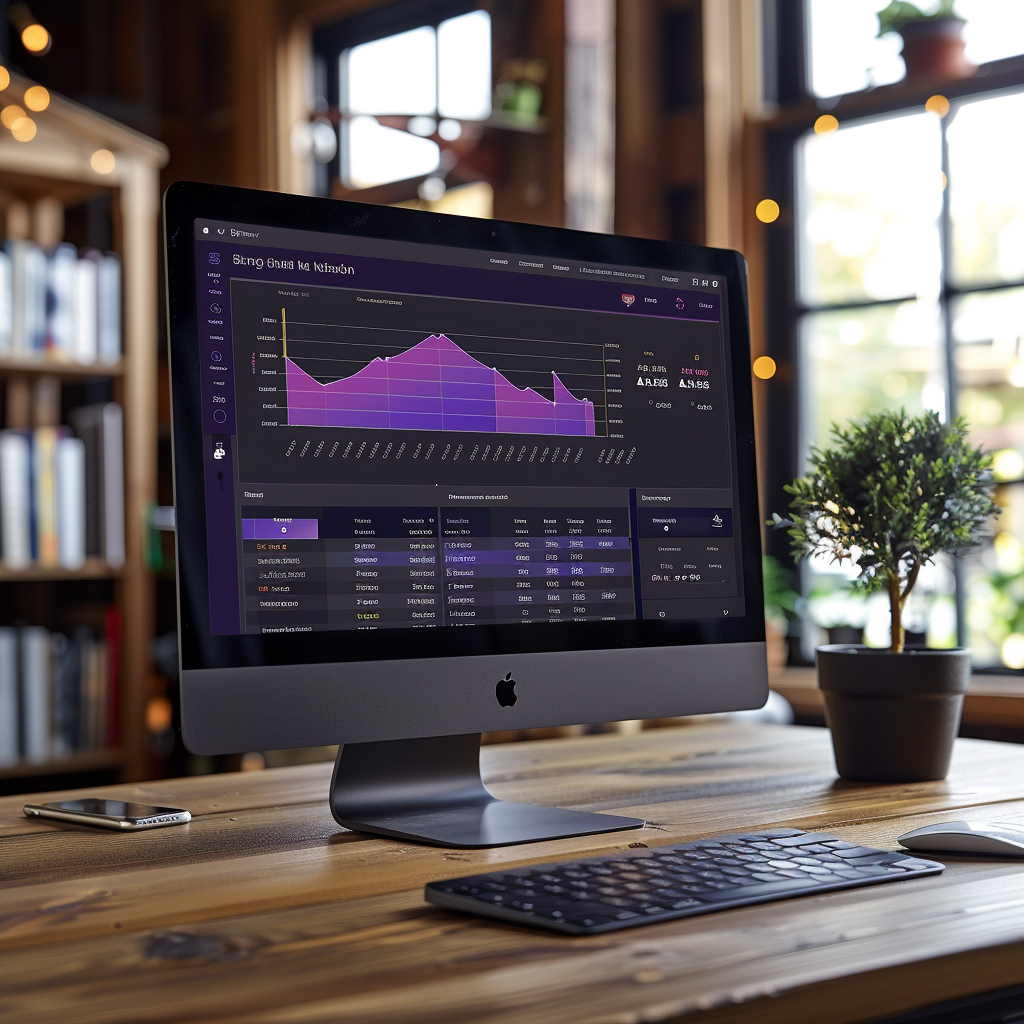
Retailers that implemented advanced AI forecasting methods report markedly better stock availability – in fact, some saw up to a 25% improvement in in-stock rates during peak demand periods after using AI-driven inventory predictions.
AI forecasts product demand using historical sales data, seasonal trends, and current market analysis to help businesses manage their inventory more efficiently. This optimization reduces the risk of overstocking or understocking, ensuring that capital is not tied up unnecessarily and that popular items are always in stock.
7. Enhanced Search Functionality
Enhanced search functionality refers to AI-powered improvements in an e-commerce site’s search bar and results. Traditional search might only match keywords, but AI allows the search engine to understand natural language queries (e.g., “comfortable black running shoes under $100”), correct spelling errors, and even learn from past user interactions to deliver more relevant results. These intelligent search systems can also personalize results based on a user’s behavior – for example, prioritizing brands or categories the user has shown interest in. The goal is to help customers find what they’re looking for faster and more accurately. A better search experience reduces frustration (less chance the user sees “no results found”) and can increase conversion rates, since users who find suitable products easily are more likely to buy. Ultimately, investing in enhanced site search leads to a smoother shopping experience and higher sales.
AI improves the search functionality on e-commerce sites by understanding natural language queries and providing more accurate search results.
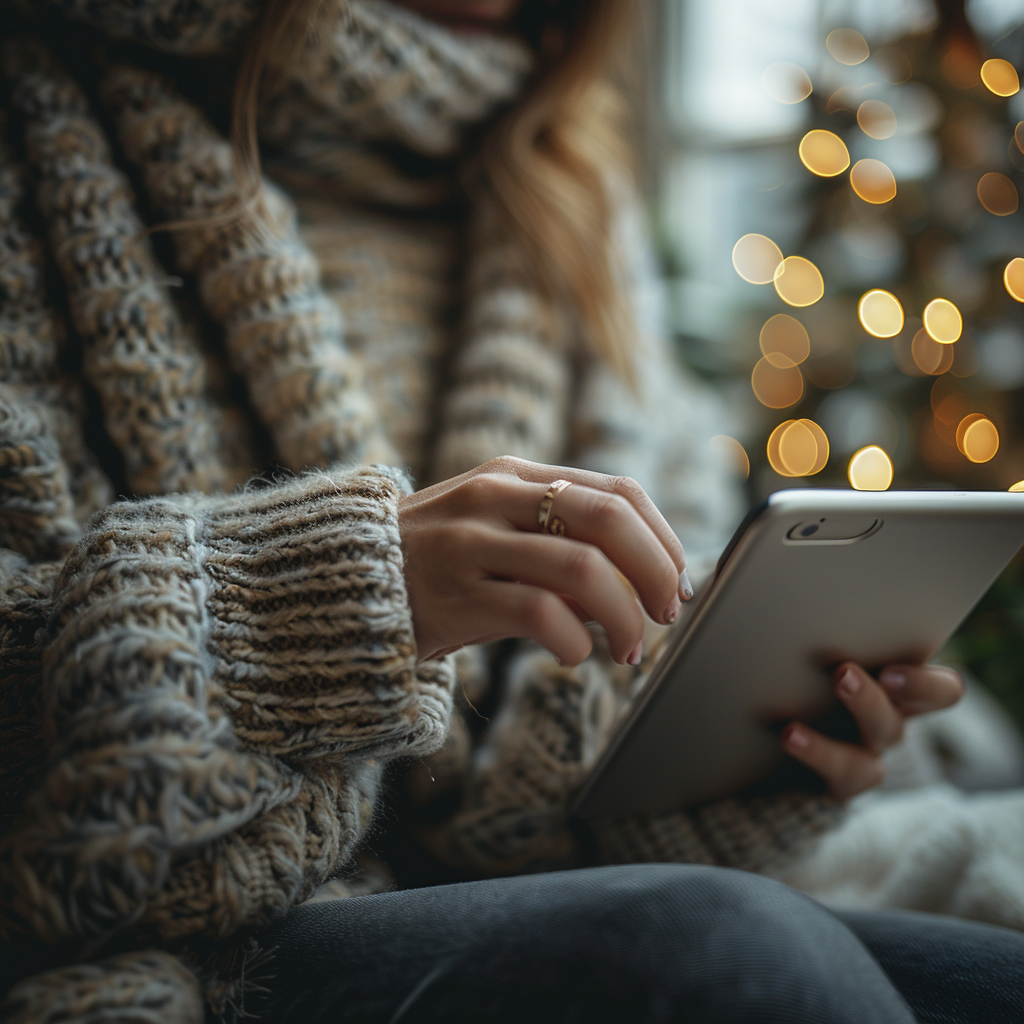
Optimizing an e-commerce site’s internal search can yield a dramatic payoff – case studies have shown that improving on-site search functionality can increase conversion rates by as much as 43%.
AI enhances the search functionality of e-commerce platforms by interpreting natural language inputs and delivering more relevant search results. This capability allows consumers to find products more easily using conversational language or detailed descriptions, significantly improving the user experience.
8. Customer Feedback Analysis
Customer feedback analysis uses AI to process and interpret large volumes of feedback, such as product reviews, customer support interactions, and social media comments. Instead of manually reading thousands of comments, companies deploy natural language processing algorithms to determine overall sentiment (how positive or negative customers feel) and to extract common themes or complaints. In e-commerce, this means a retailer can quickly discover if many customers are unhappy with, say, the quality of a new product or the checkout experience, or if a particular feature is delighting users. By quantifying sentiments and identifying trends in real time, businesses can prioritize improvements that matter most to customers. This AI-driven analysis also helps measure customer satisfaction more continuously (beyond occasional surveys) and can alert teams to emerging issues before they escalate, thereby improving service and reducing churn.
AI automates the analysis of customer reviews and feedback, extracting insights to inform product recommendations and business decisions.
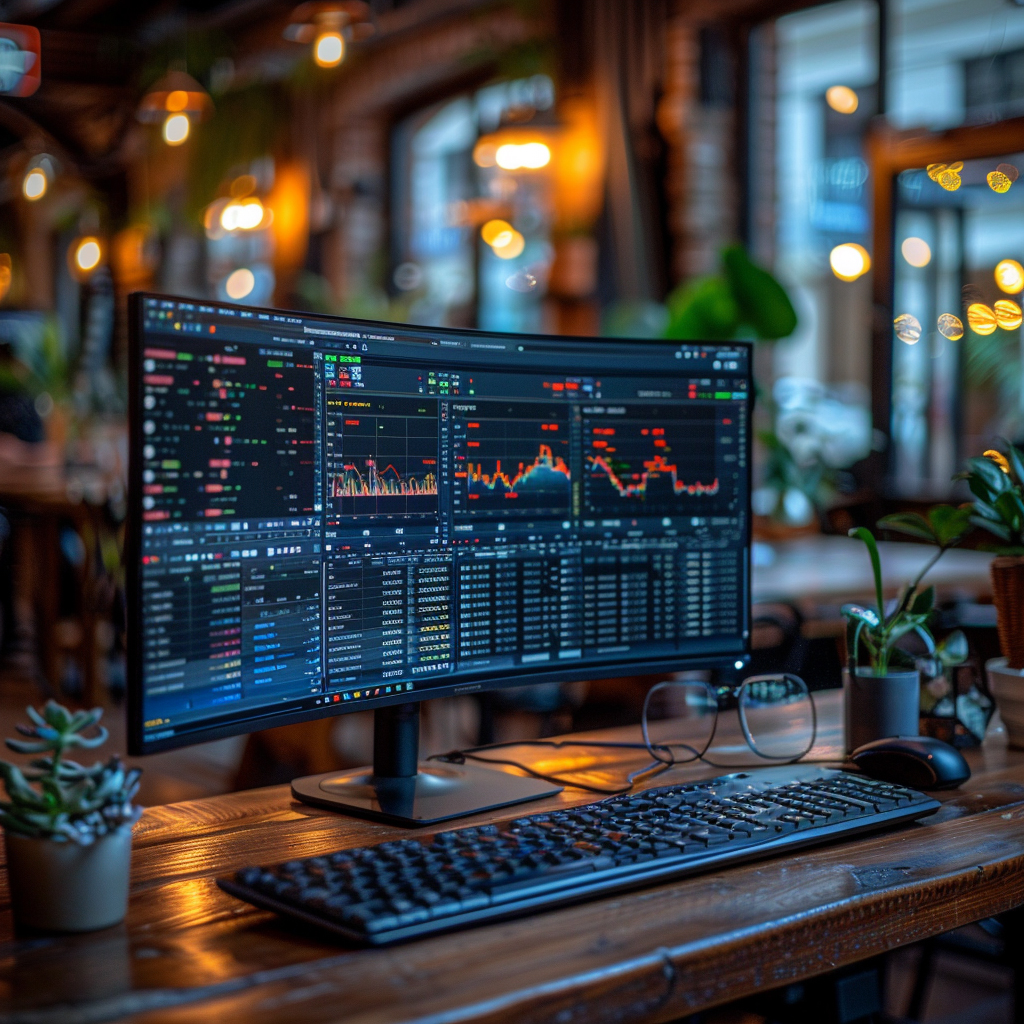
Businesses are rapidly adopting AI sentiment analysis tools as part of their customer service strategy – by the end of 2023, approximately 72% of customer service leaders had plans to pilot or implement sentiment analysis solutions to better track and act on customer feedback.
AI tools automate the processing and analysis of customer reviews and feedback. By extracting key sentiments and common themes from text data, AI provides valuable insights into customer satisfaction and product preferences, which can inform further improvements in product recommendations and service adjustments.
9. Cross-Selling and Upselling
Cross-selling and upselling are techniques to increase a customer’s value by recommending additional or higher-end products – and AI has made these tactics smarter and more personalized. Cross-selling suggests complementary items (for example, recommending a camera case and memory card to someone buying a camera), while upselling suggests a more premium version of the item or an add-on (for example, suggesting an upgraded model or warranty). AI analyzes a customer’s current selections, past purchases, and what similar customers bought to make effective recommendations in real time. In e-commerce, these suggestions often appear as “You might also like” or “Frequently bought together” sections. When done correctly, AI-driven cross-sells and upsells provide value to the customer (by highlighting useful extras or better-suited products) and boost the retailer’s average order value and revenue. It’s a balancing act – the recommendations must be relevant and timed well so they enhance the shopping experience rather than seem pushy.
AI identifies patterns in customer purchases to recommend complementary or higher-value alternatives, increasing average order value.
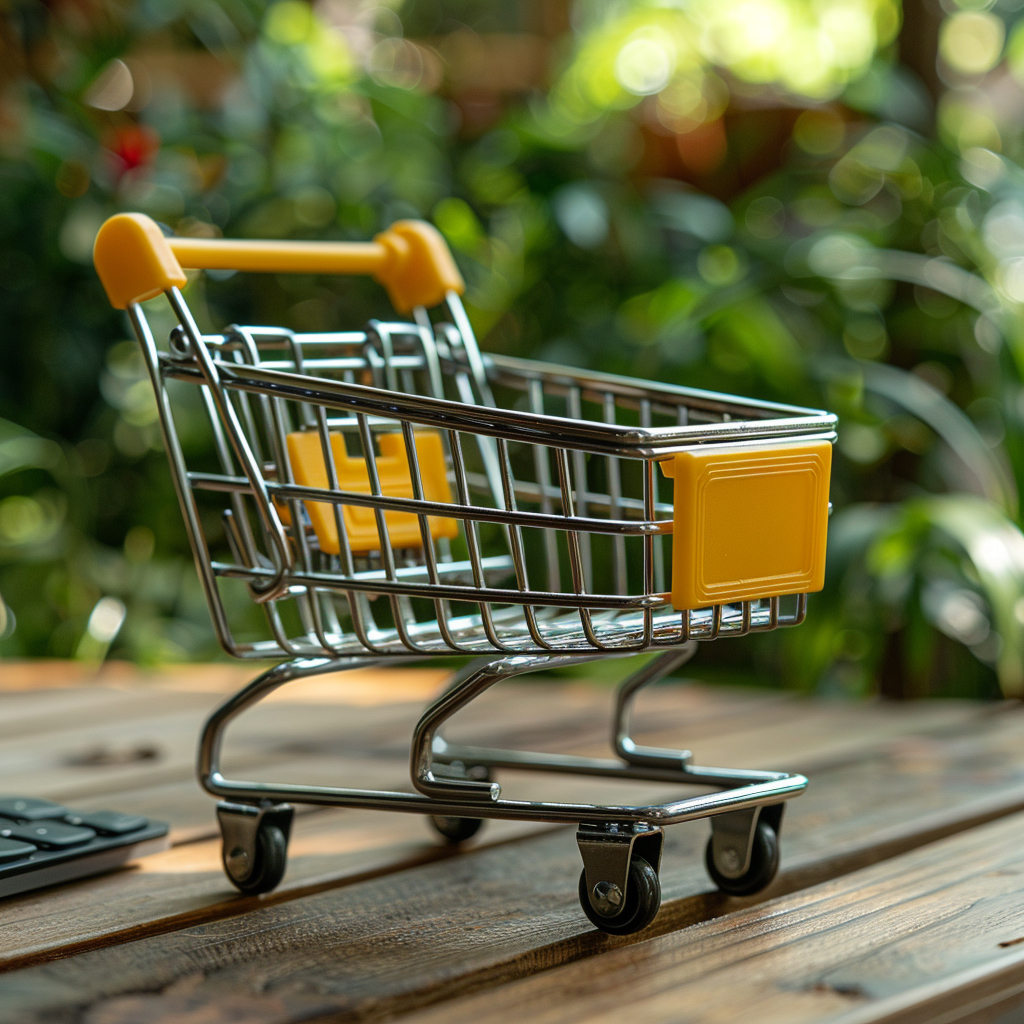
Companies that deploy AI-powered cross-selling and upselling strategies see a notable lift in revenue – on average roughly a 15% increase in sales revenue has been attributed to these personalized AI-driven recommendation tactics.
AI analyzes customer purchase histories to identify opportunities for cross-selling and upselling relevant products. By recommending complementary or premium products at the right moments, AI can increase the average order value and enhance customer satisfaction through relevant suggestions.
10. Churn Prediction
Churn prediction uses AI to identify customers who are likely to stop using a service or shopping with a business. In e-commerce and subscription services, retaining customers (reducing churn) is crucial for sustained revenue. AI models evaluate a wide range of data – purchase frequency decline, reduced website visits, lower engagement with emails, customer service interactions, etc. – to flag which customers are at risk of leaving. Once identified, companies can intervene with retention strategies, such as targeted discounts, personalized outreach, or improving certain aspects of the experience for those customers. The use of predictive analytics for churn means businesses can be proactive: rather than reactively trying to win back lost customers, they attempt to prevent the loss in the first place. Effective churn prediction and prevention improves customer lifetime value and lowers the costs associated with acquiring new customers to replace those who leave.
AI identifies signs that a customer may stop shopping and provides targeted incentives to retain them, reducing customer churn.
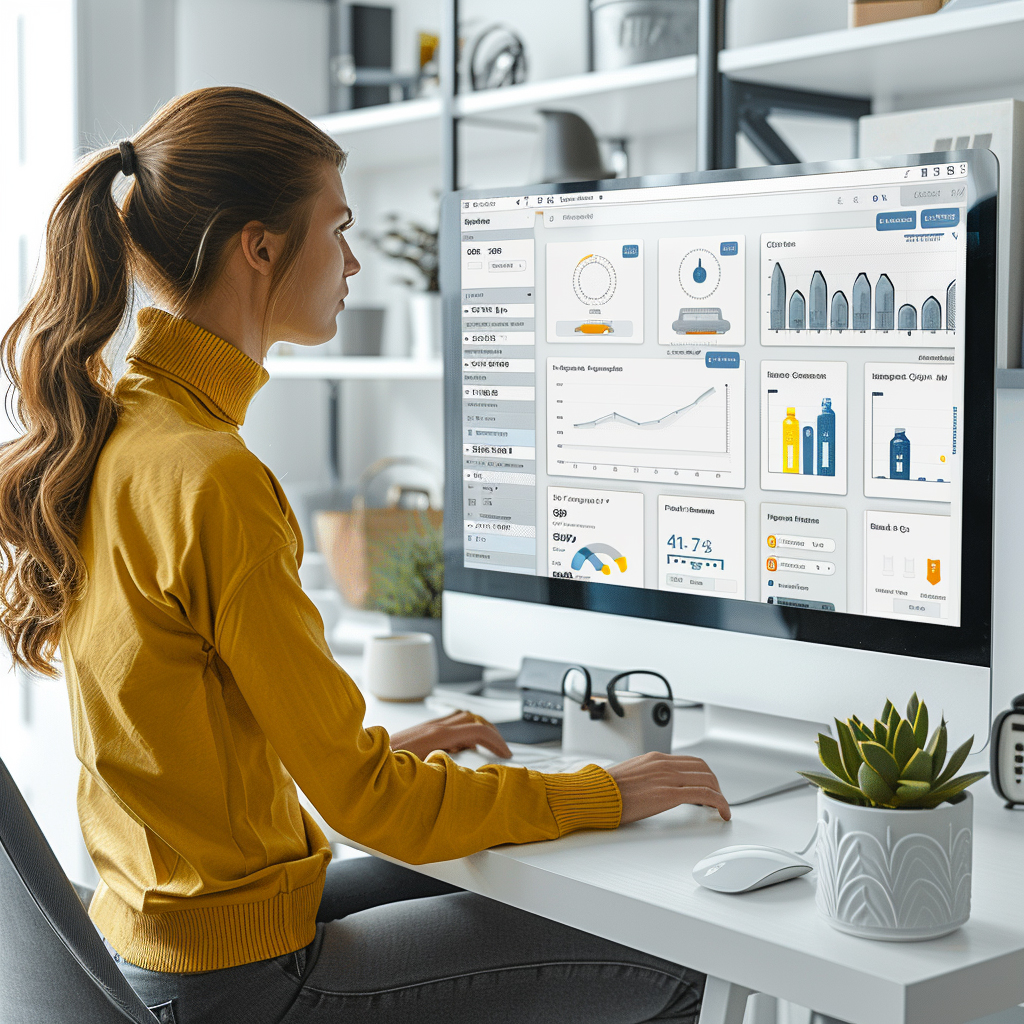
The financial stakes of preventing churn are extremely high – research by Bain & Company famously showed that increasing customer retention by just 5% can lead to profit increases of up to 75%. This underscores why churn prediction (and subsequent retention efforts) is a top priority for businesses.
AI identifies patterns and signals that a customer may be at risk of stopping their purchases. By recognizing these signs early, e-commerce platforms can proactively offer personalized incentives, discounts, or engaging content to retain these customers, effectively reducing churn rates.