1. Automated Filtering
AI automatically filters out inappropriate or harmful content based on predefined criteria such as explicit language, hate speech, or violent imagery.
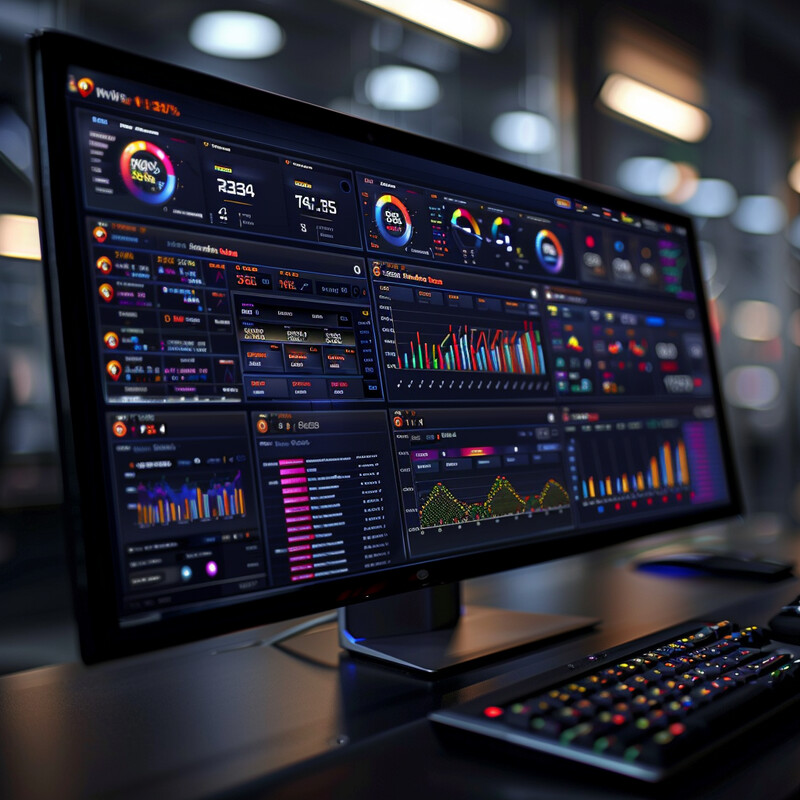
AI-powered automated filtering systems are designed to quickly identify and remove content that violates specific guidelines, such as profanity, hate speech, or explicit material. These systems use pattern recognition and natural language processing to scan text, images, and videos, ensuring that inappropriate content is flagged and, if necessary, removed before it reaches a broader audience.
2. Image and Video Analysis
Advanced AI algorithms analyze images and videos to detect nudity, violence, or other objectionable content that violates platform guidelines.
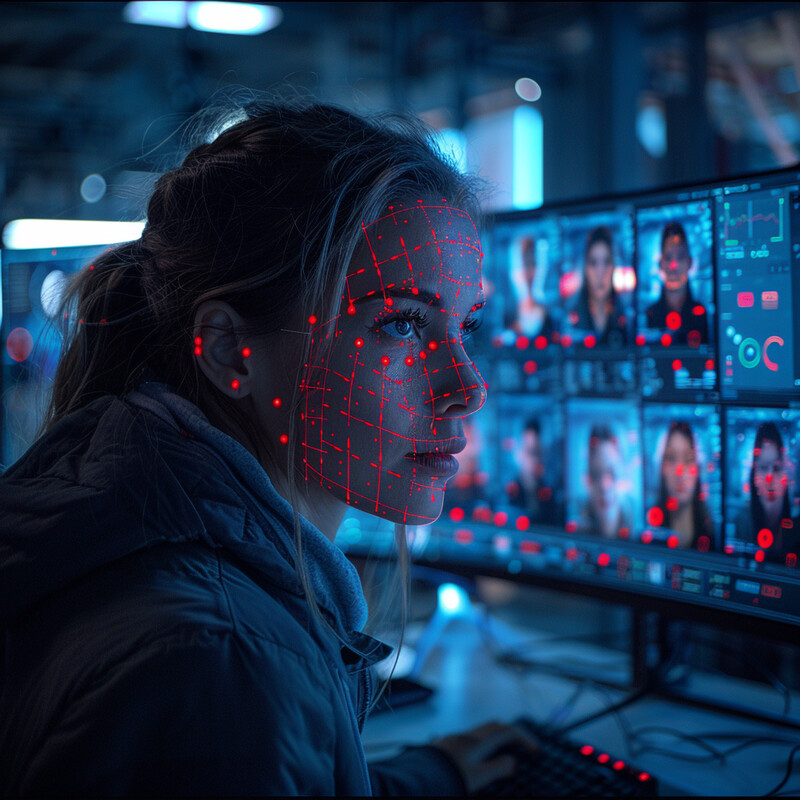
AI excels in analyzing visual content, using computer vision technologies to detect elements that may not be suitable for all viewers, such as nudity, graphic violence, or disturbing imagery. These tools are crucial for platforms that host large volumes of user-generated videos and images, providing a first line of defense against content that could harm community standards.
3. Real-time Moderation
AI enables real-time content moderation, instantly reviewing and acting on content as it is posted, which helps in maintaining the integrity of online communities.
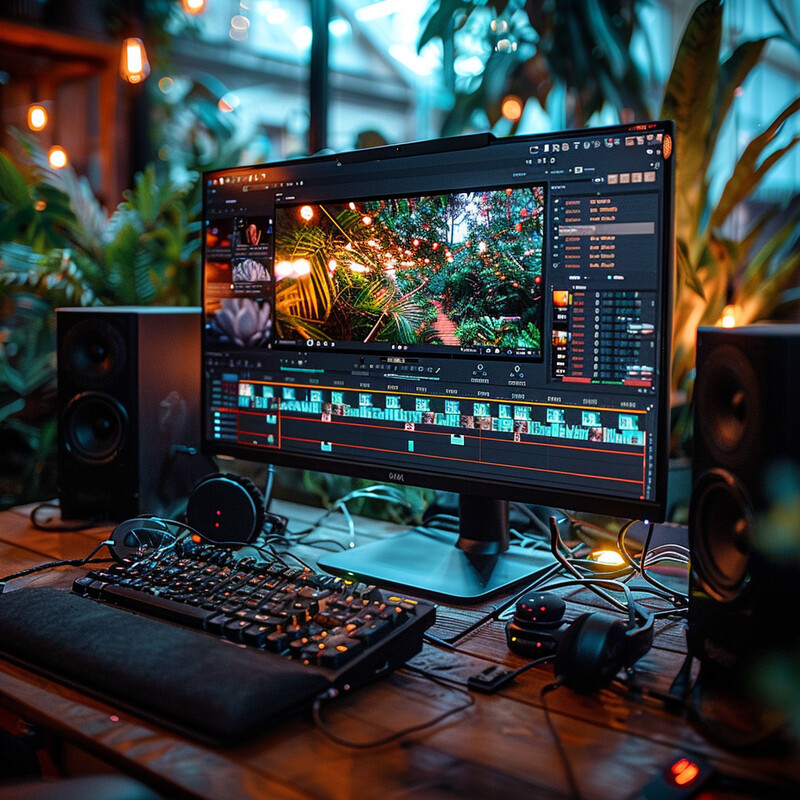
Real-time moderation powered by AI is critical for maintaining the quality and safety of user interactions as they happen. This technology allows platforms to immediately review and moderate content as it is posted, which is essential during live broadcasts or real-time comments, helping to prevent the spread of harmful content.
4. Scalability
AI systems can handle vast volumes of data, scaling up as user content grows, which is essential for large platforms with millions of users.
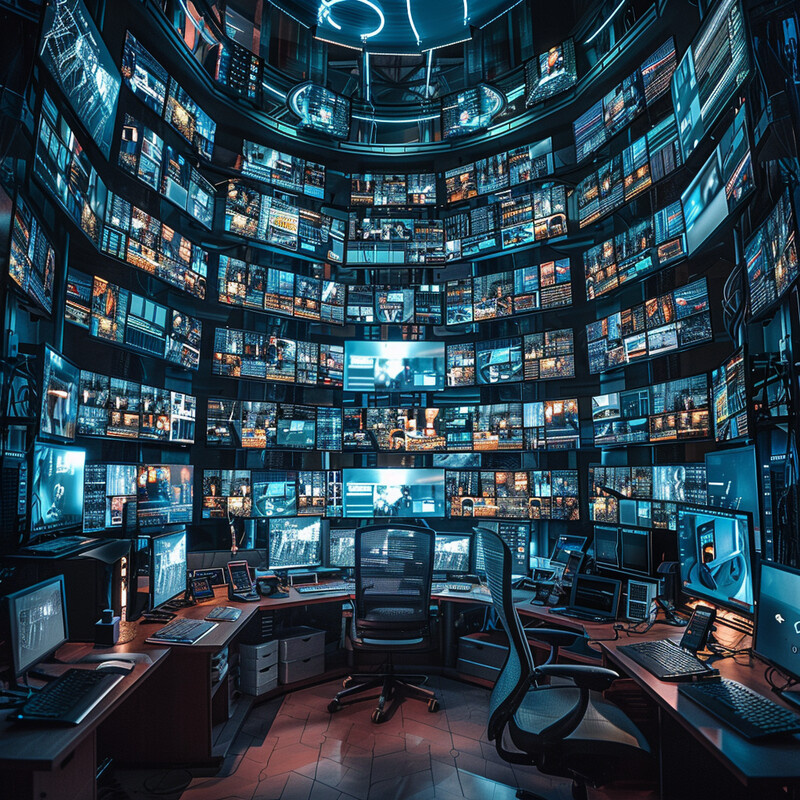
AI systems offer scalability that manual moderation teams cannot match. As the volume of user-generated content continues to grow exponentially, AI tools can scale to handle increased loads without the need for proportional increases in human resources, thereby maintaining consistent moderation standards even as user bases expand.
5. Contextual Understanding
AI has improved in understanding the context of conversations and content, which helps in distinguishing between harmful content and satire, parody, or culturally specific references.
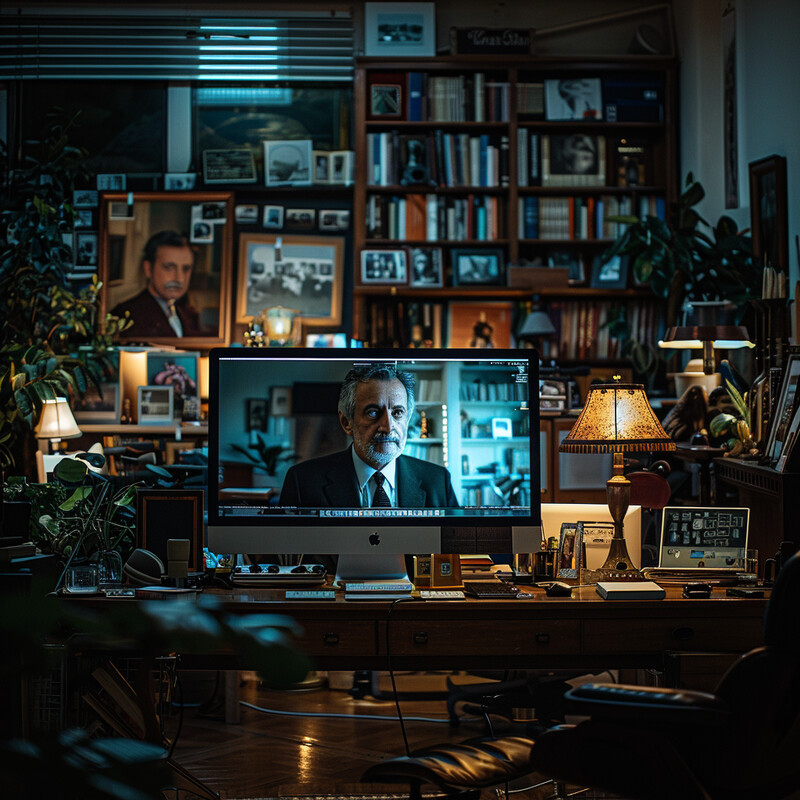
AI has advanced in understanding the context within which content is shared, which helps in distinguishing between what is genuinely harmful and what may be acceptable in certain contexts, such as satire or artistic expression. This nuanced understanding is essential to avoid over-moderation and to respect freedom of expression while keeping online spaces safe.
6. User Behavior Analysis
AI tracks user behavior over time to identify patterns that may indicate malicious activities, such as spamming or coordinated harassment campaigns.
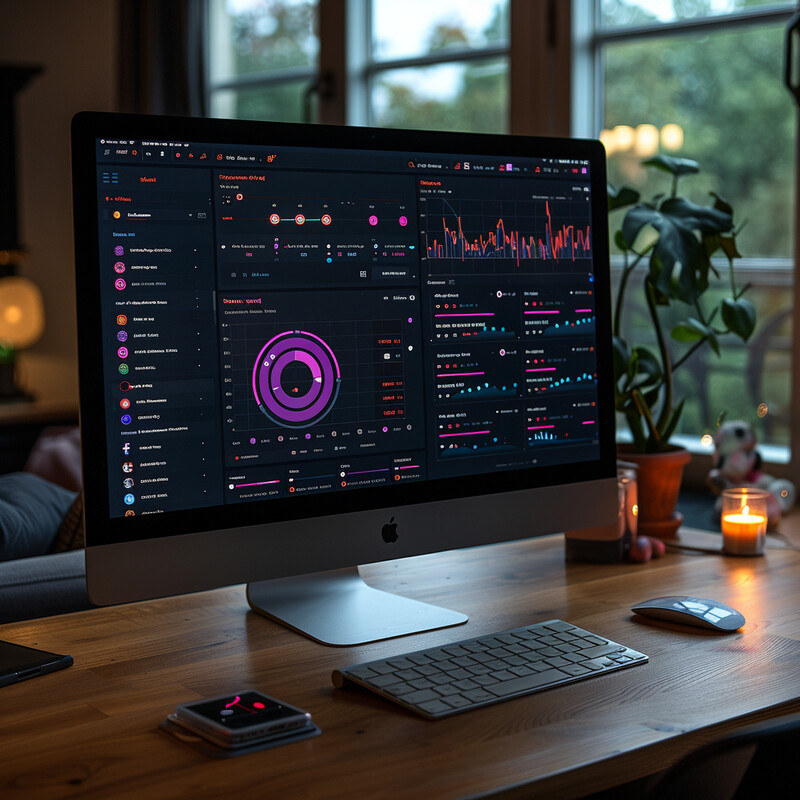
AI monitors and analyzes user behavior patterns to identify potential malicious activities. By understanding normal versus abnormal behaviors, AI can detect coordinated attacks, spamming efforts, or harassment campaigns early, allowing for timely interventions.
7. Reduced Bias
AI models are continually being trained to recognize and reduce biases in content moderation decisions, aiming for fair and consistent enforcement of rules.
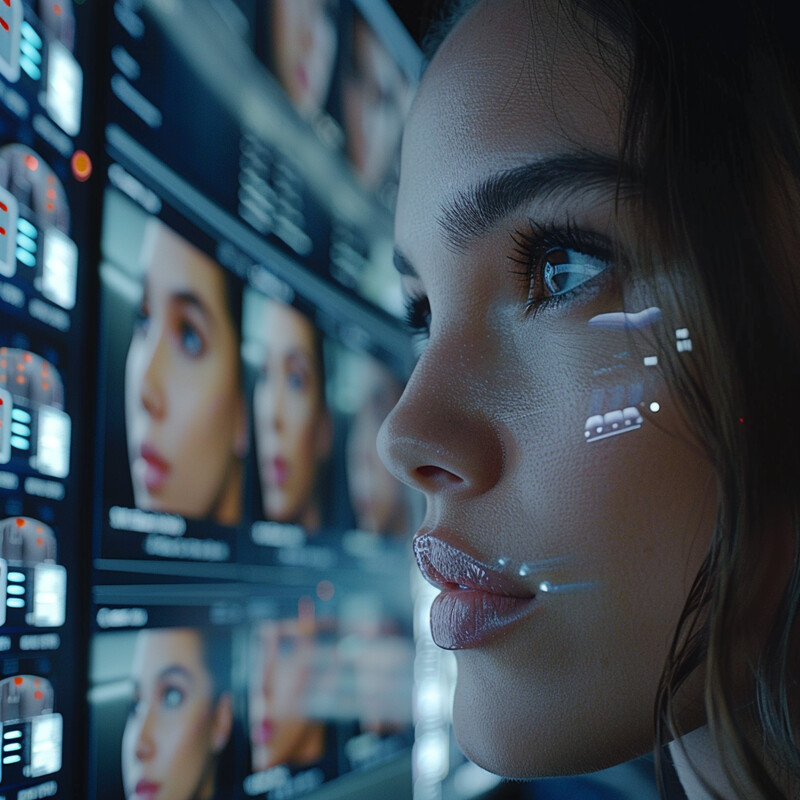
AI models are being developed and refined to reduce human biases that can affect moderation decisions. By training these models on diverse data sets and continually testing and updating them, platforms aim to achieve more objective and equitable moderation outcomes.
8. Language Support
AI-powered tools can moderate content in multiple languages, broadening the scope of moderation across global platforms and diverse user bases.
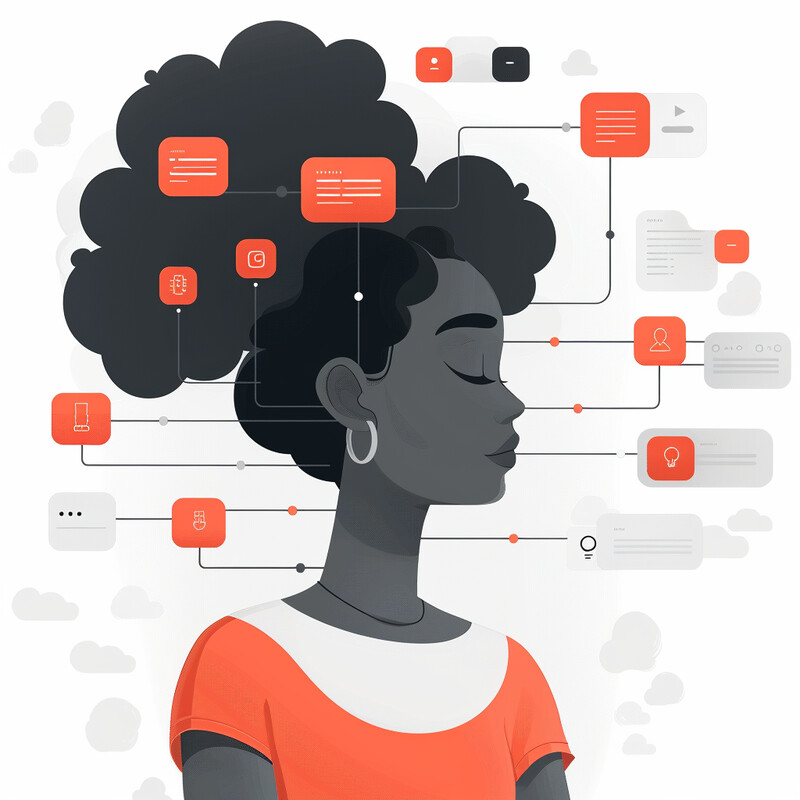
AI-powered moderation tools support multiple languages, which is crucial for global platforms with diverse user populations. These tools use advanced NLP capabilities to understand and moderate content in various languages, ensuring consistent community standards across different linguistic groups.
9. Feedback Loops
AI systems use feedback from moderators and users to learn and improve their accuracy, adapting to new forms of inappropriate content and changing community standards.
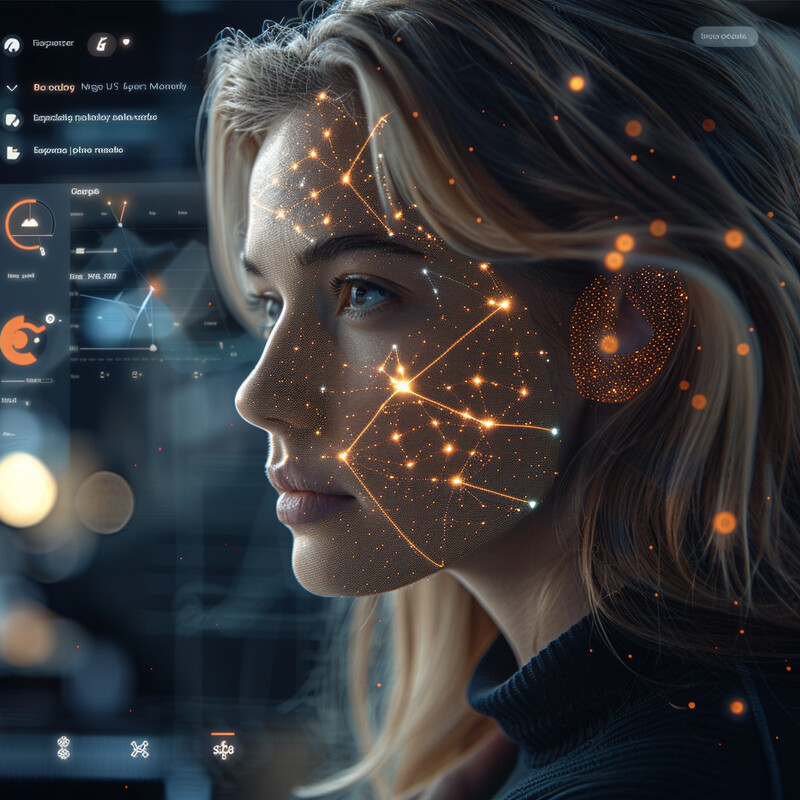
Feedback loops are integral to AI systems, allowing them to learn from moderation outcomes and user reports. This ongoing learning process helps AI tools become more accurate over time and adapt to new forms of inappropriate content or changes in social norms and standards.
10. Predictive Moderation
AI predicts potential violations by analyzing emerging trends and user reports, allowing platforms to proactively address issues before they escalate.
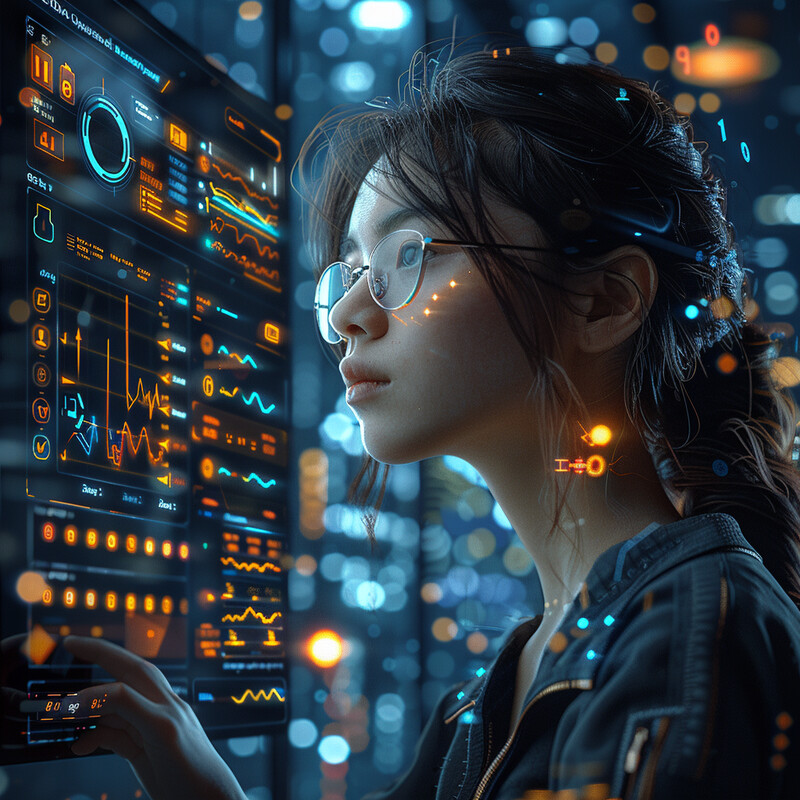
AI uses predictive analytics to foresee potential issues based on emerging trends and user reports. This proactive approach enables platforms to prepare and react before a situation escalates, potentially preventing widespread harm or disruption.