1. Material Property Prediction
AI predicts the properties of new composite materials based on their molecular structure and composition, enabling researchers to design materials with targeted characteristics before physical testing.
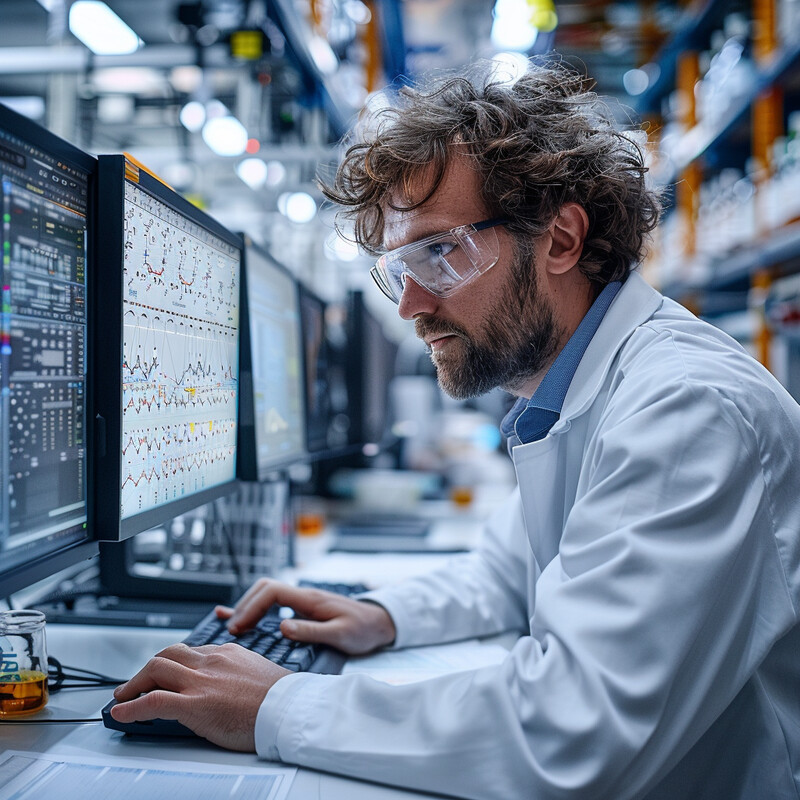
AI uses machine learning models to predict the physical, chemical, and mechanical properties of composite materials based on their molecular structures and compositions. By training these models on historical data, researchers can anticipate how new composites will perform, enabling the targeted design of materials with specific characteristics without the need for extensive physical trials.
2. Optimization of Material Formulations
AI algorithms optimize composite formulations by simulating different combinations of materials and processing conditions to achieve the best performance metrics.
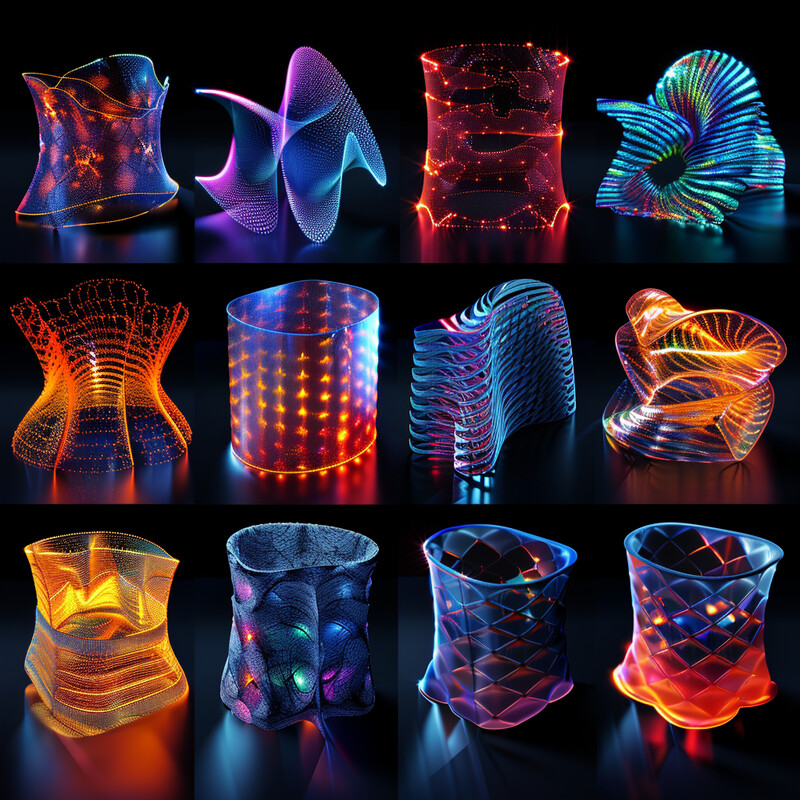
AI algorithms analyze vast amounts of data to optimize composite formulations, simulating different combinations of fibers, resins, and other additives under varied processing conditions. This allows for the fine-tuning of composites to achieve optimal strength, durability, and other desired properties, significantly speeding up the development process.
3. Quality Control
AI is used to monitor and control the quality of composite materials during production, using real-time data analysis to detect defects or deviations from standards.
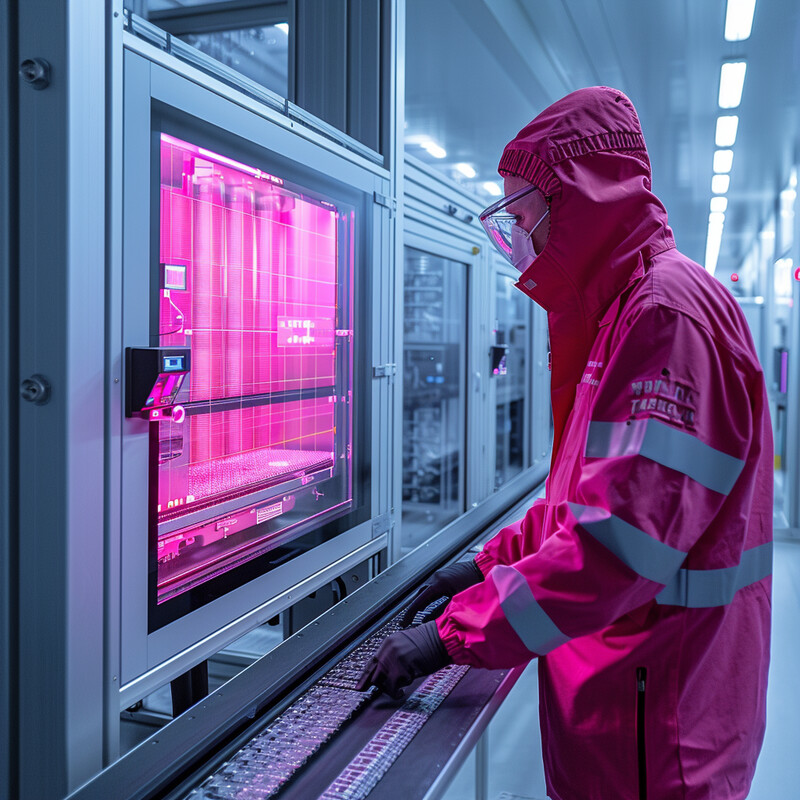
AI enhances quality control in the production of composite materials by monitoring the manufacturing process in real-time. Using sensors and data analytics, AI systems detect anomalies or defects in materials, such as inconsistencies in fiber distribution or resin composition, ensuring that only products meeting high standards proceed to the market.
4. Predictive Maintenance in Manufacturing
AI predicts when equipment used in the manufacturing of composite materials might fail or require maintenance, minimizing downtime and maintaining consistent production quality.
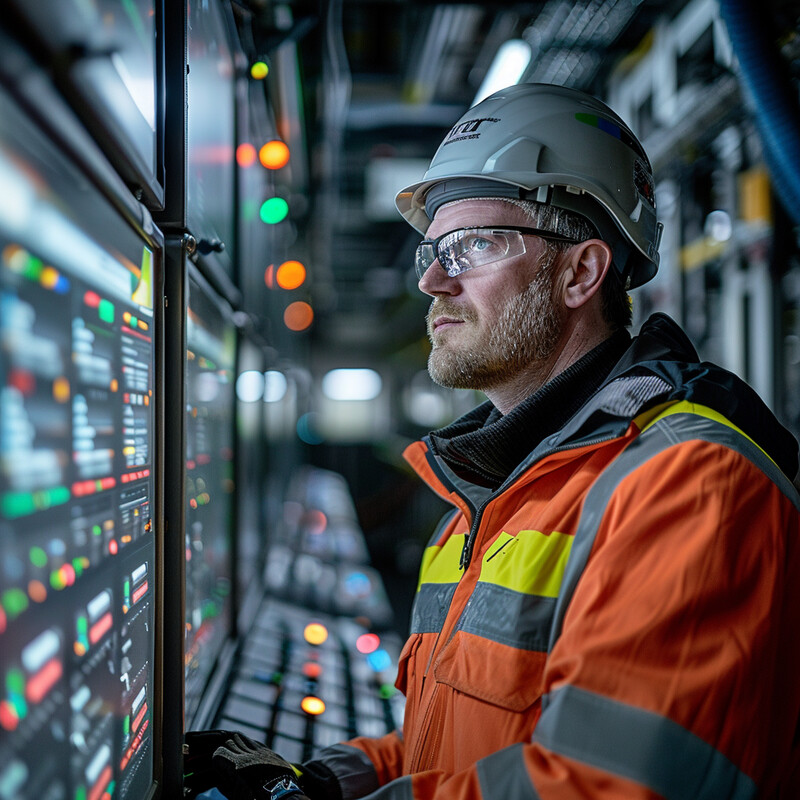
In the manufacturing of composite materials, AI predicts maintenance needs of machinery using data from sensors monitoring operational conditions. This predictive maintenance helps avoid unexpected equipment failures, reducing downtime and maintaining consistent quality and output in production lines.
5. Supply Chain Optimization
AI optimizes the supply chain for raw materials needed in composite manufacturing by predicting demand, managing inventory, and suggesting optimal procurement strategies.
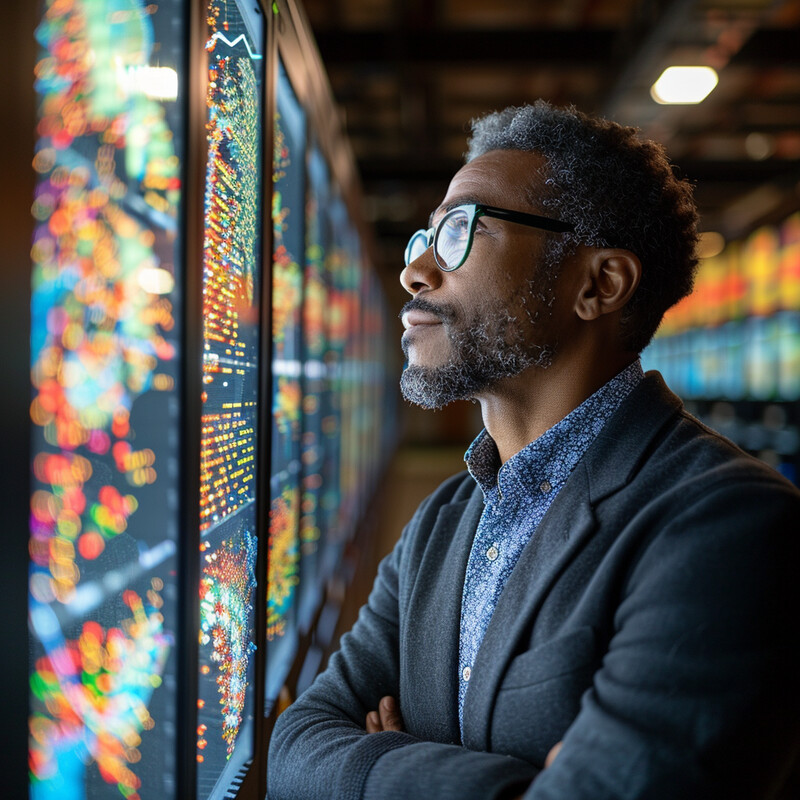
AI optimizes the supply chain management of raw materials needed for composite manufacturing by analyzing patterns in supply and demand, inventory levels, and logistics data. This results in more efficient inventory management, reduced costs, and timely availability of materials, ensuring smooth production processes.
6. Failure Analysis
AI analyzes failure points in composite materials used in various applications, identifying patterns that can inform future improvements in material design and application.
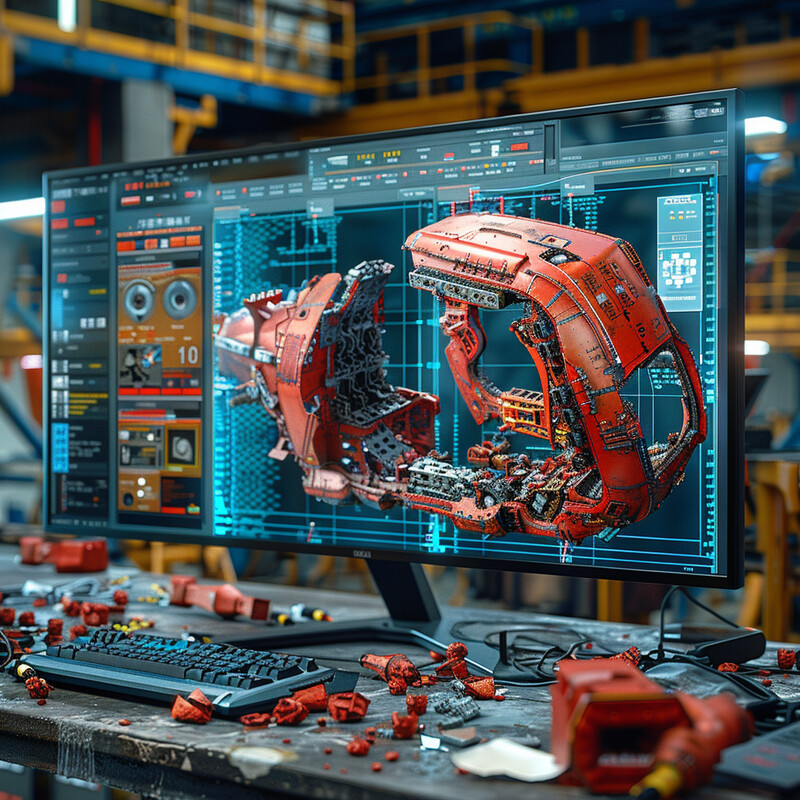
AI analyzes data from failed composite materials to identify common failure points and conditions. This analysis helps engineers understand why materials fail under certain conditions and guides the improvement of material formulations and designs to enhance performance and safety.
7. Customization for Specific Applications
AI aids in customizing composite materials for specific applications, such as aerospace, automotive, or sports equipment, ensuring that the materials meet precise industry standards and performance requirements.
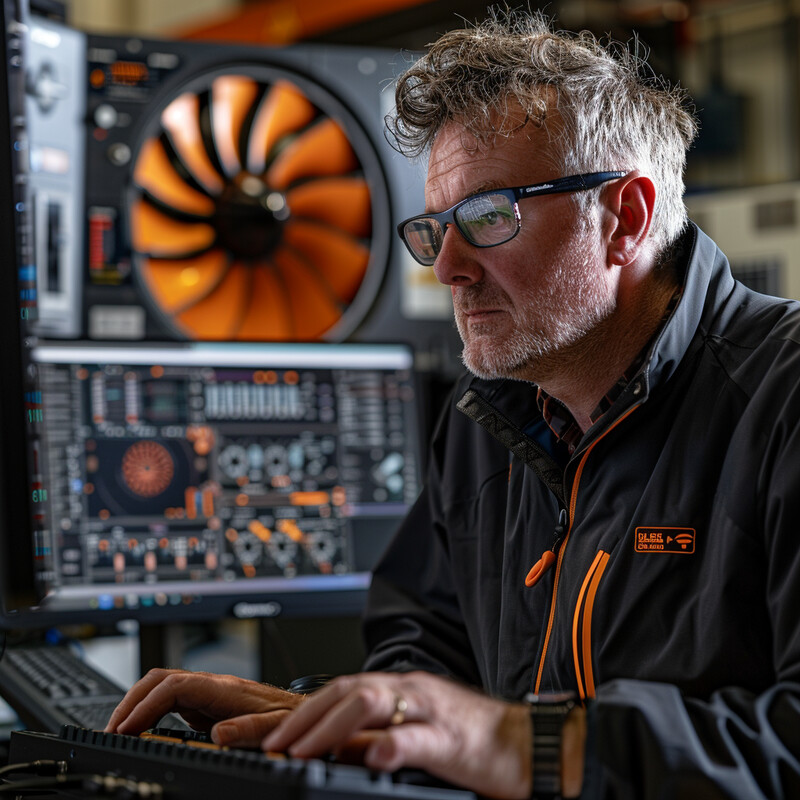
AI facilitates the customization of composite materials for specific industrial applications by simulating performance under conditions unique to each sector, such as high temperatures in aerospace or impact resistance in automotive. This ensures that composites meet the exact requirements of different industries, enhancing functionality and compliance with stringent standards.
8. Recycling and Sustainability
AI models optimize the recycling processes for composite materials, identifying the best methods for breaking down and reusing materials in an environmentally friendly manner.
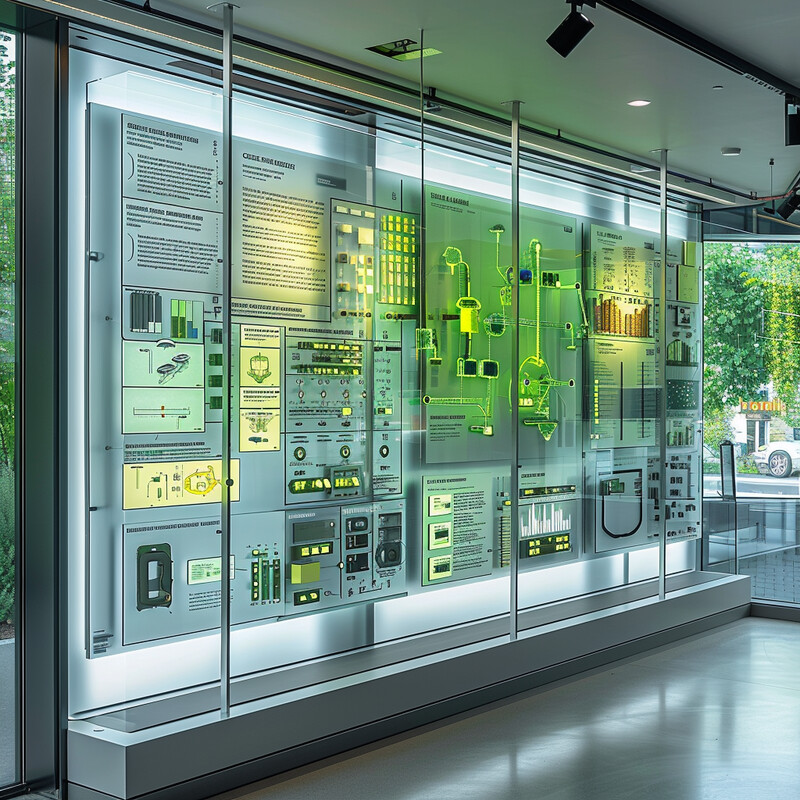
AI models are employed to improve the recycling processes for composite materials. These models identify the most efficient methods for material breakdown and recovery, aiming to enhance the sustainability of composites by minimizing waste and promoting the reuse of materials in new products.
9. Integration with Robotic Systems
AI integrates with robotic systems to automate the complex processes involved in laying up composite materials, ensuring precision and efficiency in manufacturing.
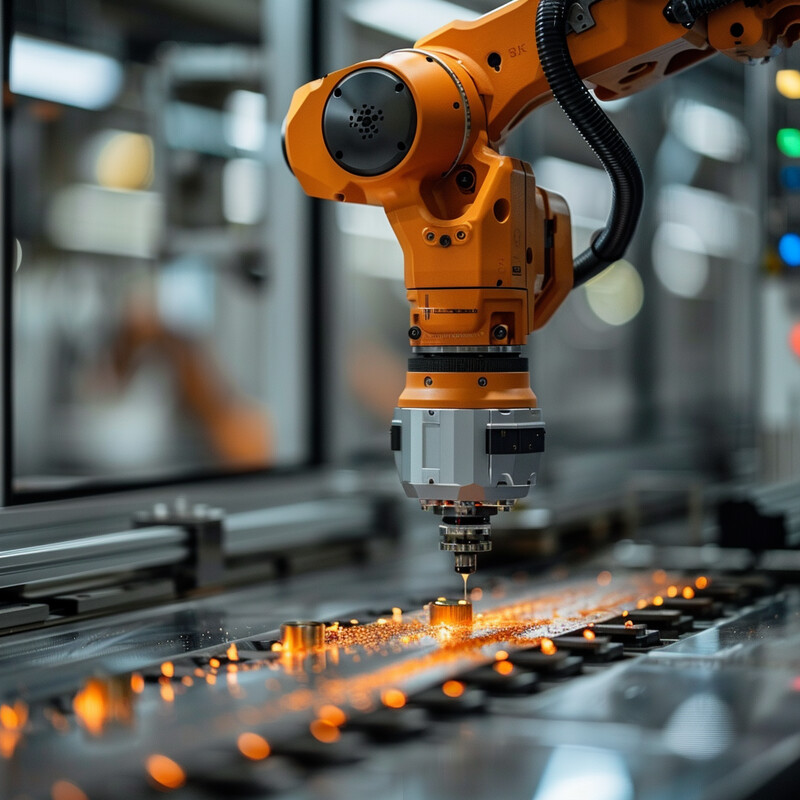
AI-driven robotic systems automate the precise and repetitive tasks involved in laying up composite materials, such as arranging fibers and applying resins in specific patterns. This automation not only speeds up the manufacturing process but also increases the repeatability and accuracy of composite structures.
10. Enhanced Simulation and Modeling
AI improves the simulation and modeling of composite materials under various stress conditions and environments, providing valuable insights into how materials will perform over their lifecycle.
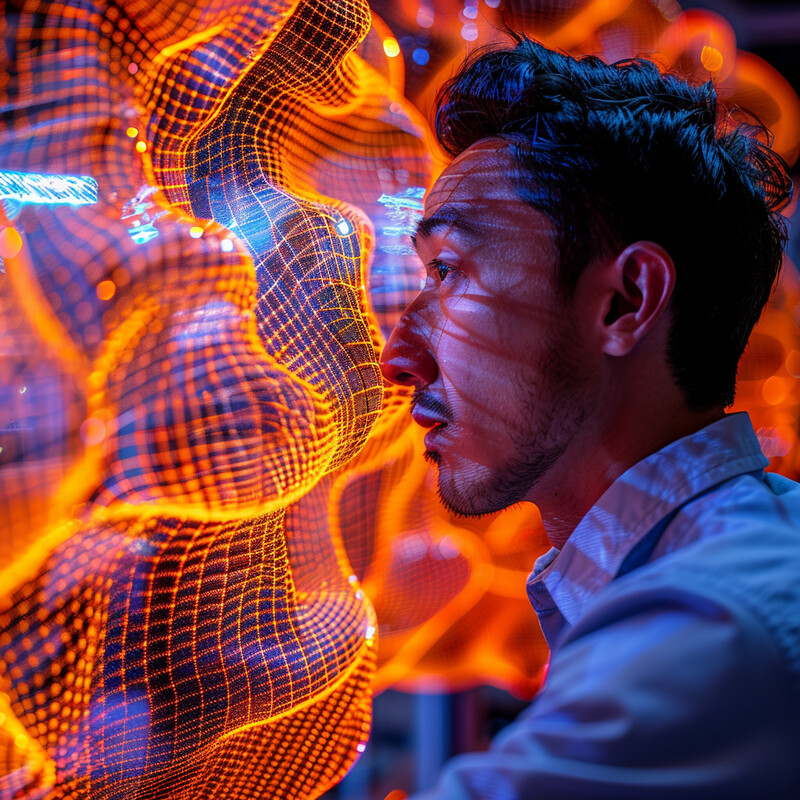
AI significantly improves the simulation and modeling of composite materials, allowing researchers to predict how these materials will behave under different stress scenarios and environmental conditions. Advanced simulations help in understanding the long-term durability and performance of composites, guiding better designs and more reliable application in real-world conditions.