1. Diagnostic Assistance
AI algorithms analyze medical data, such as imaging and lab results, to assist in diagnosing diseases more accurately and quickly than traditional methods
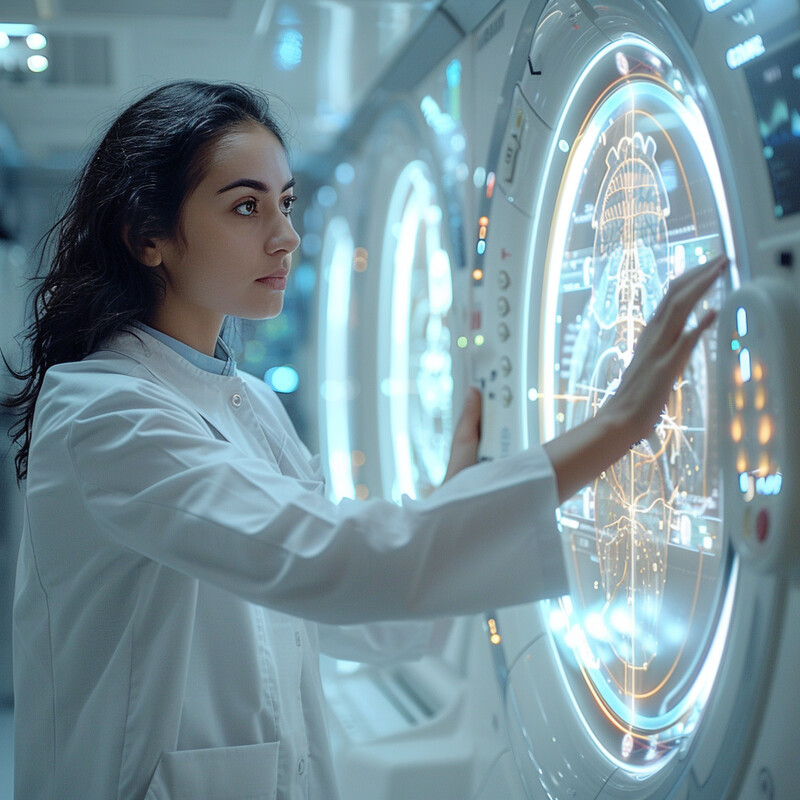
AI algorithms significantly enhance the diagnostic process by analyzing medical images, lab results, and patient data to identify patterns and anomalies that may indicate disease. Tools like deep learning can interpret complex imaging data, such as CT scans or MRIs, to detect conditions like tumors or fractures more quickly and accurately than traditional methods, reducing diagnostic errors and improving patient outcomes.
2. Treatment Recommendations
AI provides personalized treatment recommendations by analyzing patient data alongside vast medical knowledge bases and previous outcomes.
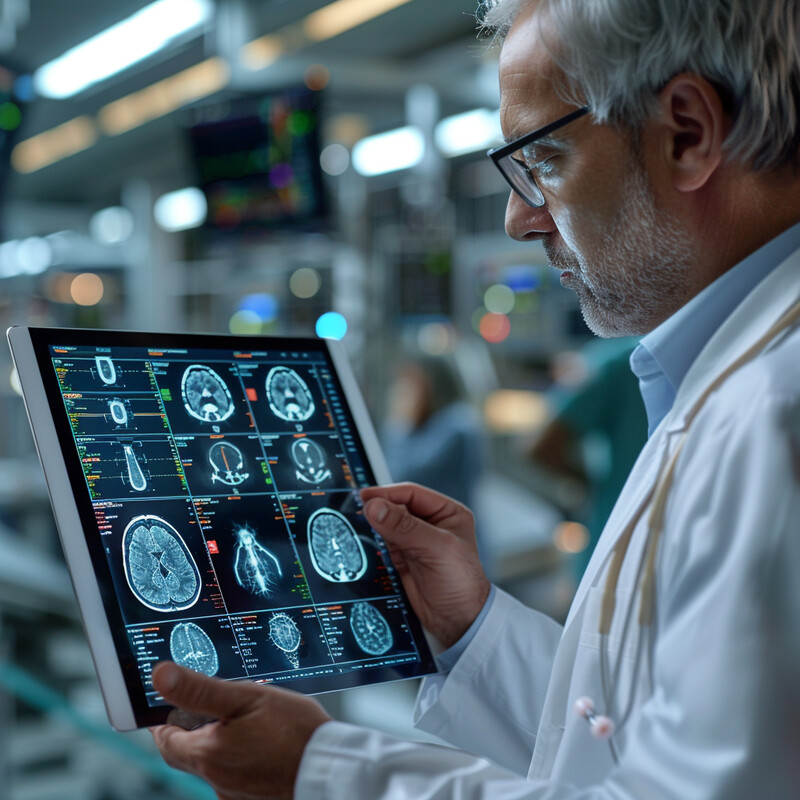
AI leverages vast databases of clinical research and patient histories to generate personalized treatment recommendations. By comparing a patient's detailed health profile against outcomes from similar cases, AI can suggest the most effective therapeutic approaches, tailored to each patient's specific condition and medical history, ensuring a higher precision in treatment planning.
3. Drug Interaction Alerts
AI systems monitor patient prescriptions and alert healthcare providers to potential drug interactions, enhancing patient safety.
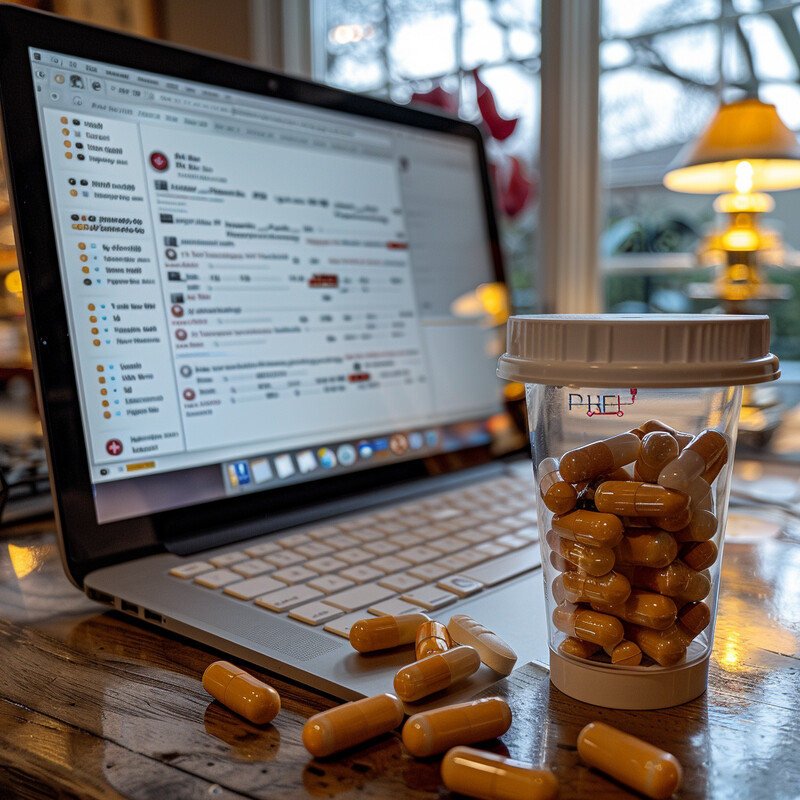
AI systems integrate with electronic health records (EHRs) to monitor patients' medication lists for potential adverse drug interactions. These systems use complex algorithms to analyze prescribed medications and alert healthcare providers if combinations pose a risk, thereby enhancing patient safety and preventing harmful side effects.
4. Predictive Analytics for Patient Risk
AI uses historical data and real-time inputs to predict patients at high risk for certain conditions, allowing for early intervention and tailored care plans.
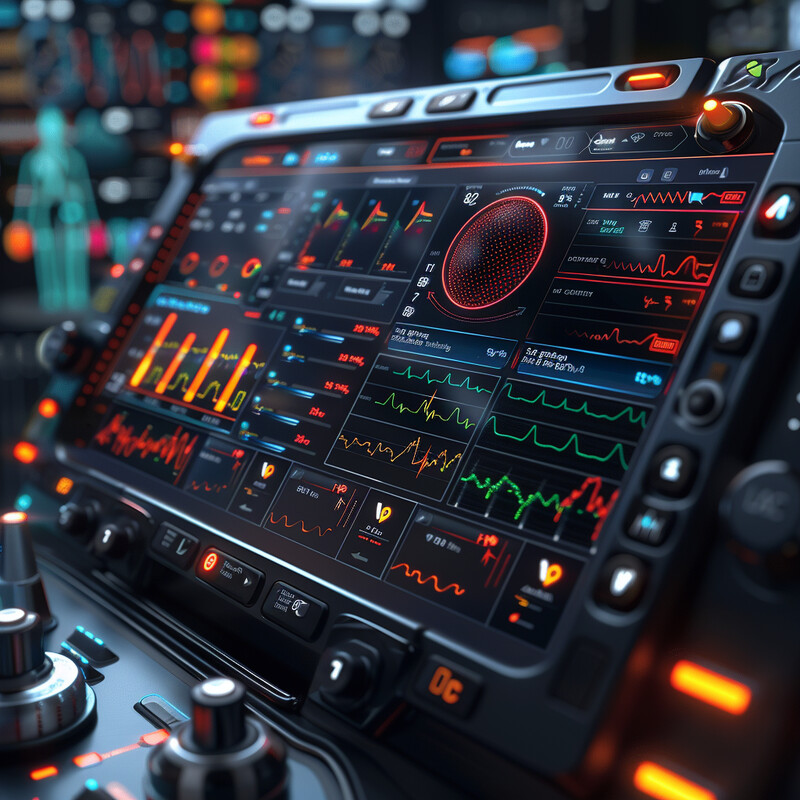
AI employs predictive analytics to identify patients at risk of developing certain conditions, such as sepsis or chronic illnesses, based on real-time data analysis and historical health data. This foresight allows healthcare providers to implement preventative measures or more closely monitor at-risk individuals, potentially saving lives by preempting critical health issues.
5. Patient Monitoring and Alerts
AI continuously monitors patient vitals and other health data in real-time, providing alerts to healthcare providers if abnormal patterns are detected.
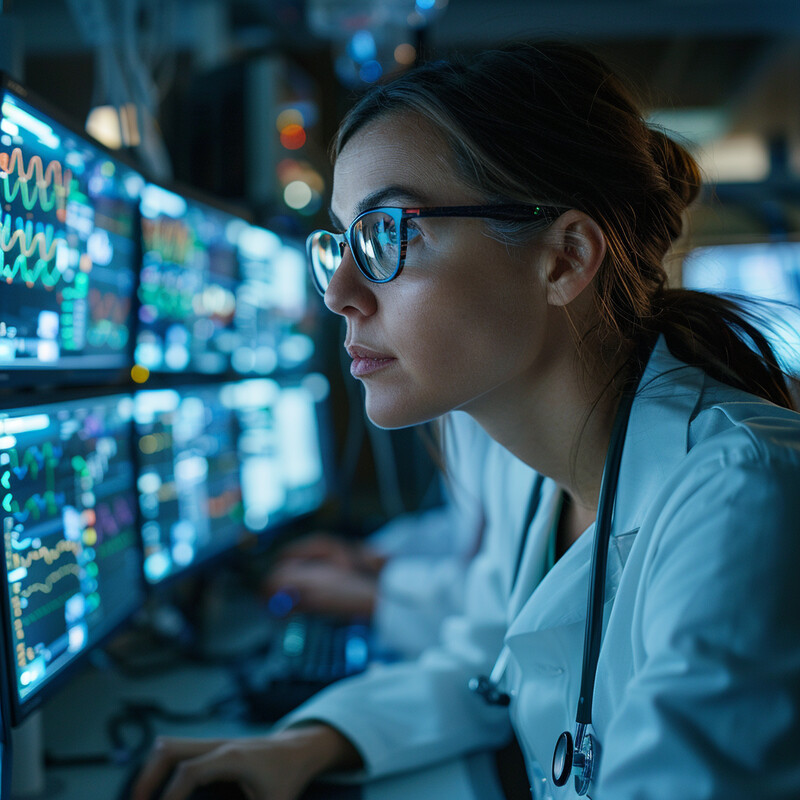
In environments like intensive care units, AI systems continuously analyze patient vitals and other health metrics, providing real-time alerts to healthcare professionals when abnormal patterns are detected. This immediate responsiveness can be critical in emergency situations, facilitating swift medical intervention to address potential complications.
6. Streamlining Workflow
AI automates routine tasks such as data entry and appointment scheduling, freeing up healthcare professionals to focus more on patient care.
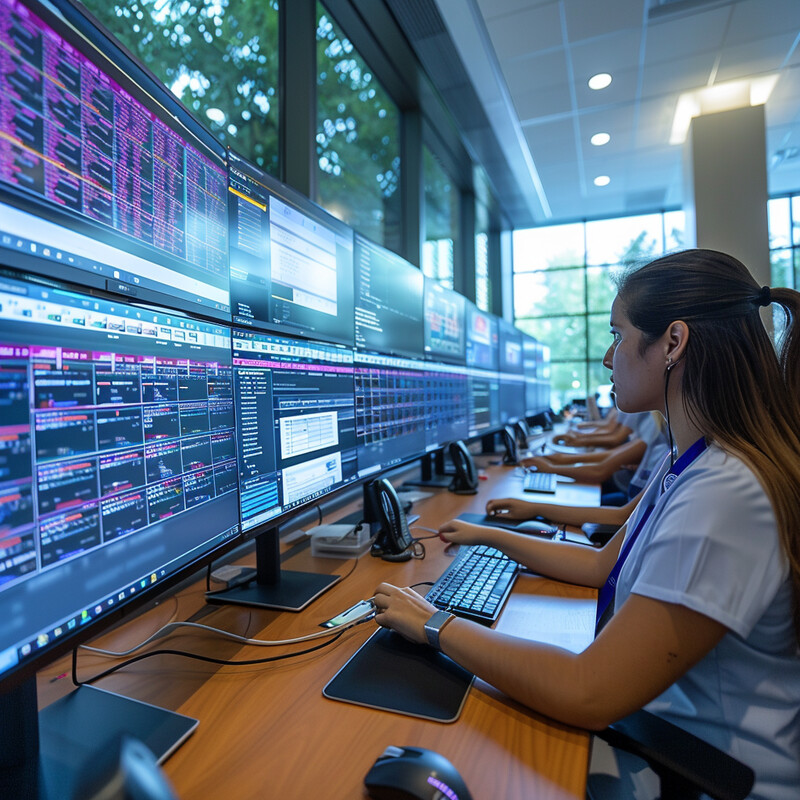
AI automates administrative tasks within clinical settings, such as data entry, appointment scheduling, and patient triage. This automation streamlines healthcare workflows, reducing the administrative burden on staff and allowing them to devote more time and resources to direct patient care.
7. Evidence-Based Treatment Protocols
AI analyzes the latest research and clinical data to suggest evidence-based treatment protocols, ensuring that patients receive the most effective care.
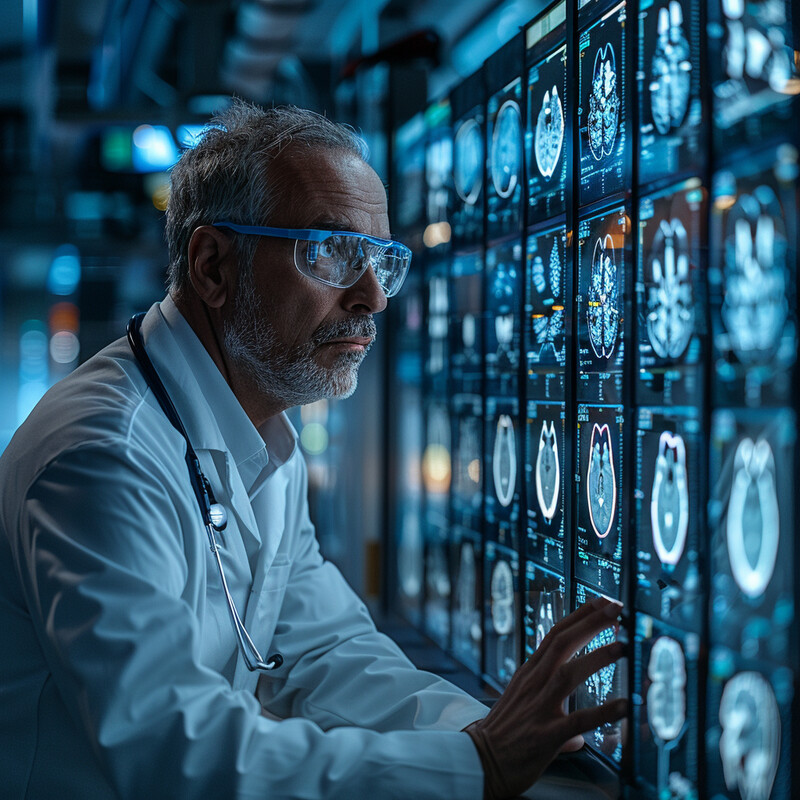
AI systems are continually updated with information from the latest clinical studies and proven treatment protocols. By accessing current, evidence-based guidelines, these systems help healthcare providers make informed decisions that align with the most recent medical research and regulatory approvals.
8. Natural Language Processing (NLP)
AI uses NLP to interpret unstructured data, such as doctor's notes and clinical journals, making this information accessible and actionable for treatment planning.
-1.jpg)
AI utilizes NLP to interpret and organize unstructured data from clinical notes, discharge summaries, and medical journals. This capability allows healthcare providers to quickly extract and use relevant information for patient care, improving efficiency and accuracy in handling patient records.
9. Outcome Prediction
AI models predict the outcomes of different treatment plans based on similar patient profiles and historical data, helping clinicians make more informed decisions.
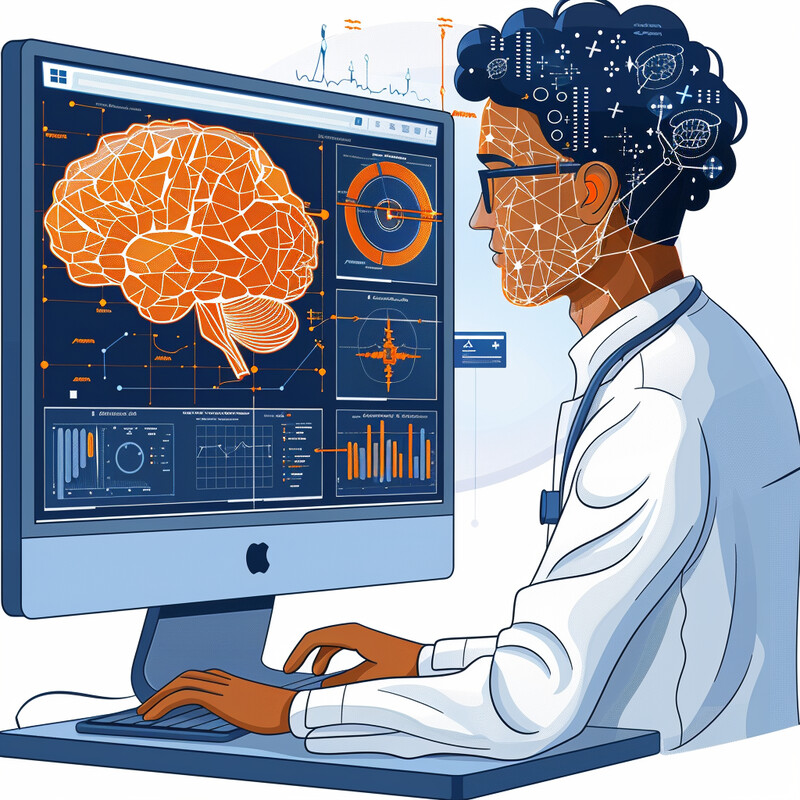
AI models analyze patient data and predict the likely outcomes of various treatment options. These predictions are based on analyses of similar historical cases and can help clinicians choose the most effective treatment strategies, minimizing trial and error and enhancing patient care planning.
10. Personalized Medicine
AI integrates genetic information with clinical data to tailor treatments to the individual genetic profile of each patient, promoting more effective and personalized care.
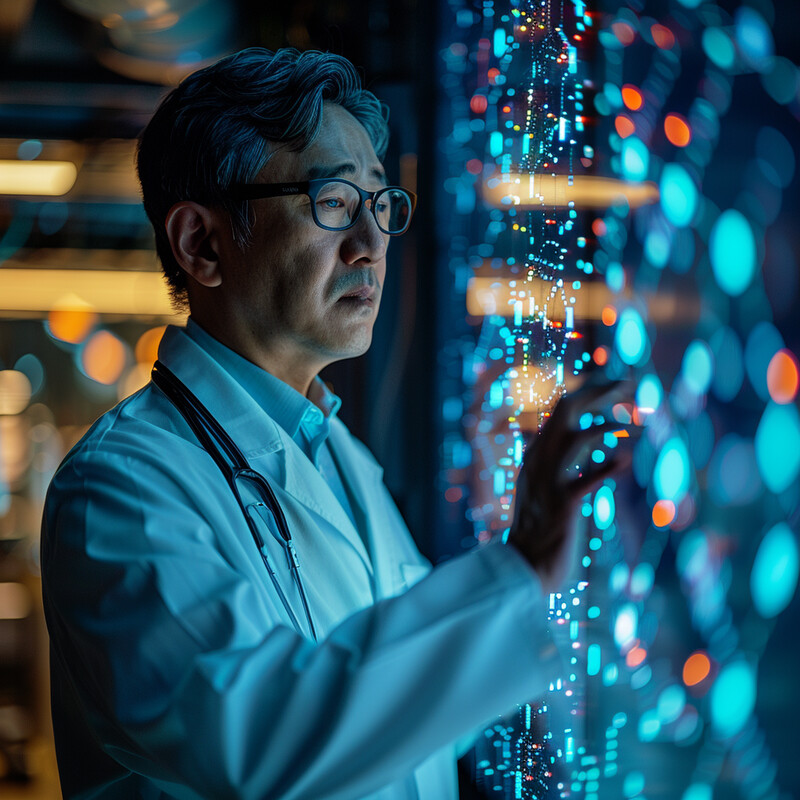
Incorporating genetic data into clinical decision-making, AI tailors treatments to the genetic profiles of individual patients. This approach is particularly effective in fields like oncology, where AI can suggest specific medications or therapy plans that are more likely to be effective based on the genetic markers of a patient's tumor.