1. Enhanced Facial Recognition Accuracy
AI algorithms are constantly refined to improve the accuracy of facial recognition systems, even under varying lighting conditions, angles, or with partial facial obstructions.
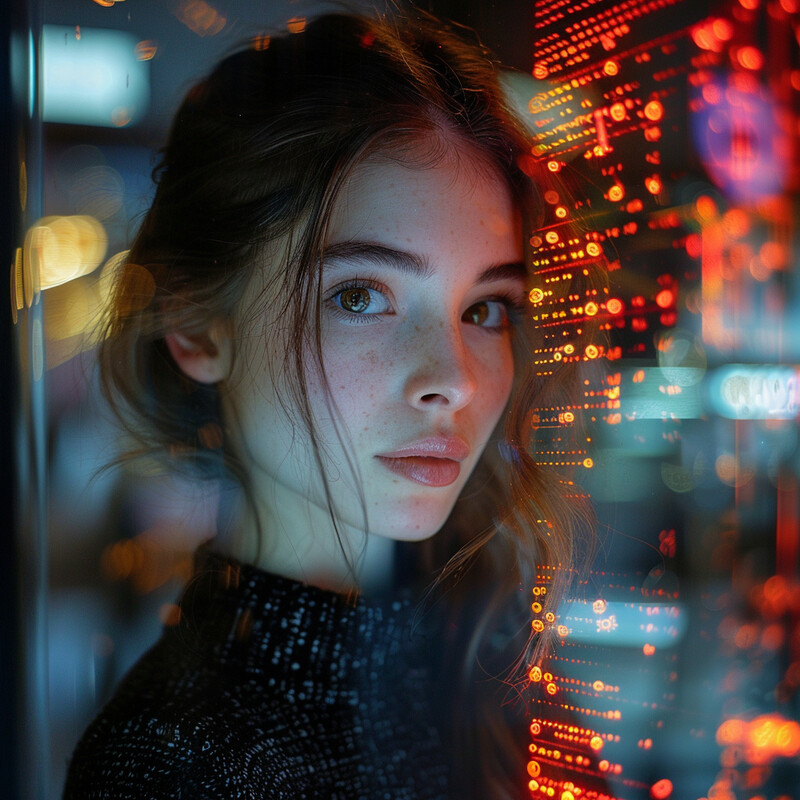
AI significantly enhances the accuracy of facial recognition systems by applying deep learning algorithms that can analyze and recognize faces under varied conditions. These systems are trained on diverse datasets to recognize faces across different lighting, angles, facial hair, cosmetics, or even when partially obscured. This improvement ensures reliable authentication and reduces false negatives, making systems more robust and user-friendly.
2. Adaptive Voice Recognition
AI enhances voice recognition systems by adapting to changes in a user's voice due to factors like age, illness, or background noise, maintaining high accuracy over time.
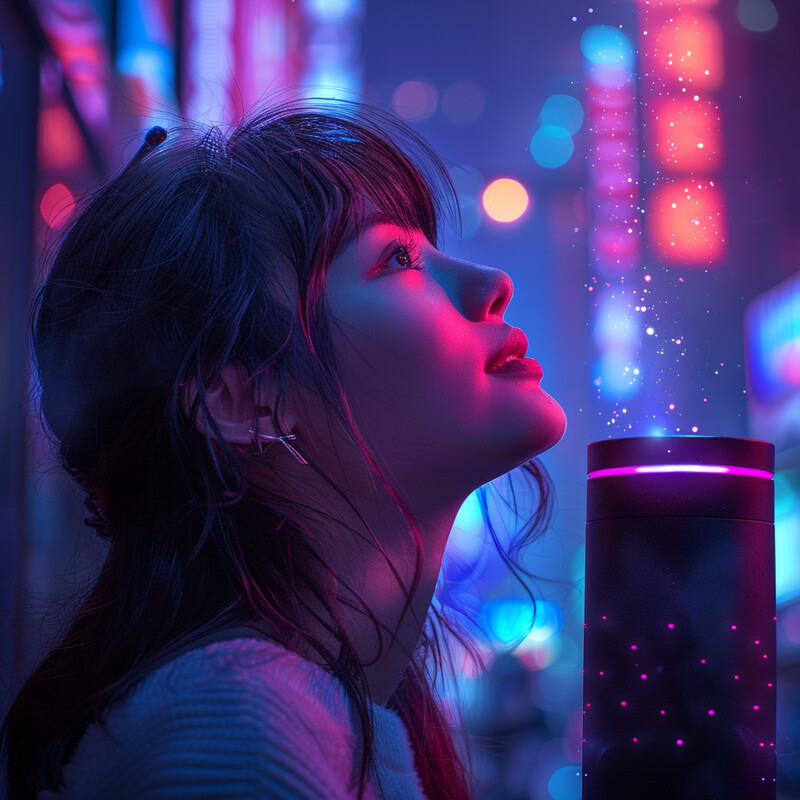
AI improves voice recognition systems by enabling them to adapt to changes in a user's voice, which might occur due to aging, health conditions, or background noise. By continuously learning from new voice data, AI ensures that the voice recognition remains accurate throughout the user’s life, enhancing both security and convenience.
3. Fingerprint Analysis Improvement
AI uses advanced pattern recognition techniques to analyze minutiae in fingerprints more accurately, reducing false rejections and increasing reliability.
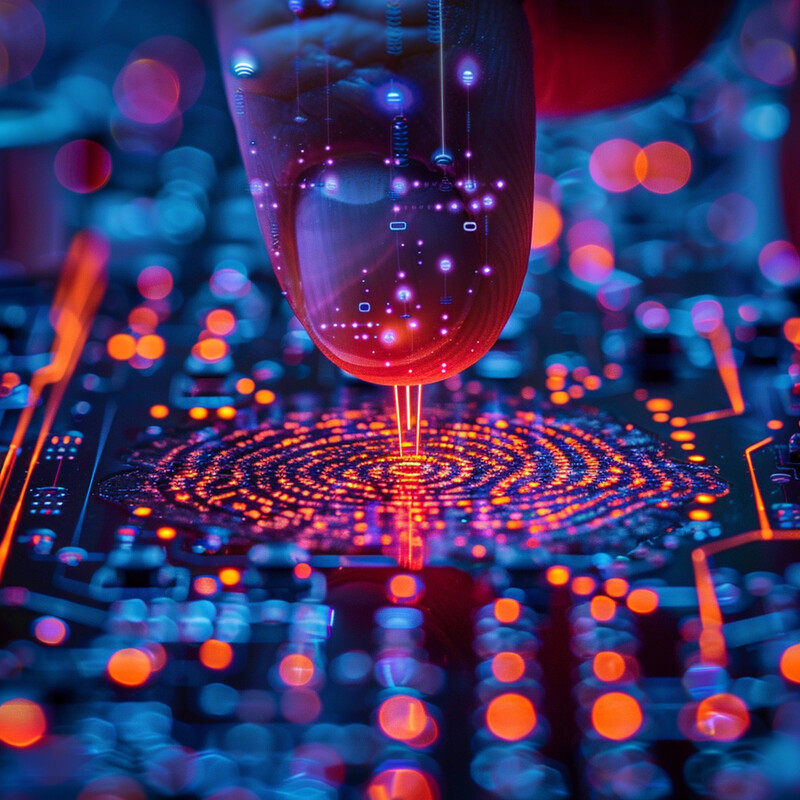
AI refines fingerprint analysis by employing sophisticated pattern recognition algorithms to examine the minutiae points more accurately. These improvements help in reducing the rate of incorrect rejections due to slight variations in finger placement, pressure, or skin condition, thereby increasing the reliability of fingerprint scanners.
4. Iris Recognition Enhancement
AI-powered systems refine iris recognition technologies by better handling occlusions like glasses or contact lenses and adapting to physiological changes over a person’s lifetime.
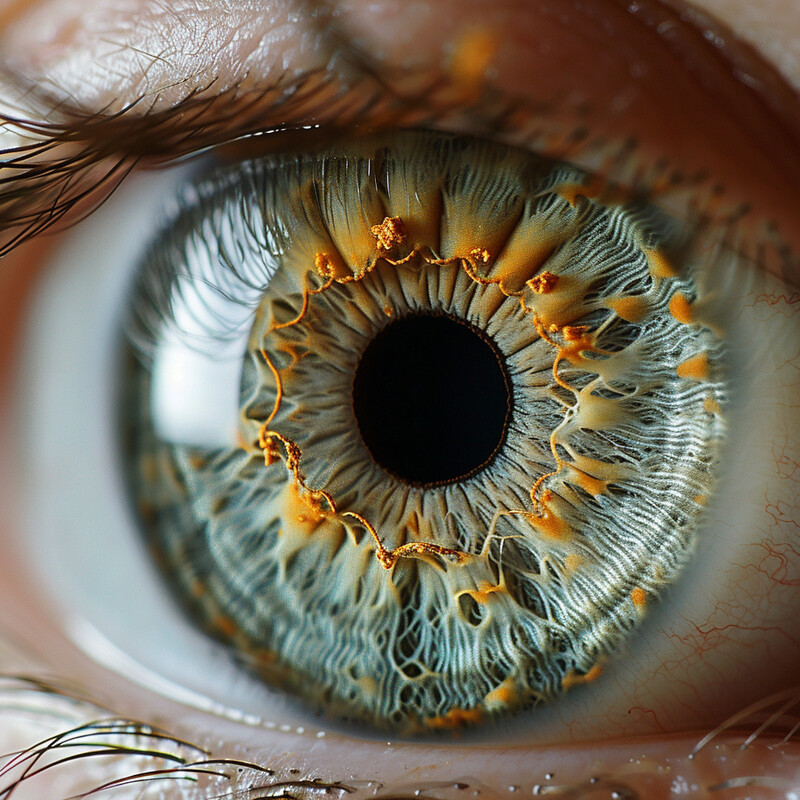
AI-powered enhancements in iris recognition involve sophisticated image recognition capabilities that can accurately identify individuals even when there are occlusions like eyeglasses or contact lenses. AI algorithms adjust for reflections, distortions, and other image artifacts, ensuring high accuracy and reducing false rejections.
5. Behavioral Biometrics
AI analyzes patterns in behavior such as typing rhythms, mouse movements, and walking patterns to create user profiles that enhance security measures without overt user interaction.
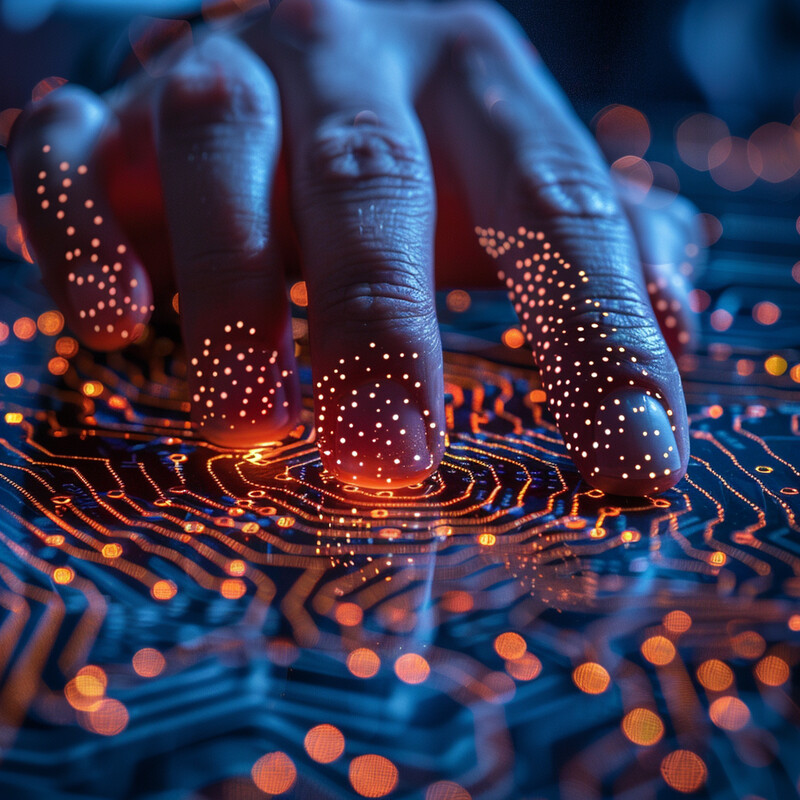
AI leverages patterns in user behavior such as typing rhythm, mouse movements, and gait analysis to authenticate users. By analyzing these behavioral patterns, AI creates a dynamic and continuously updated model of the user's behavior, which can subtly but effectively confirm the user’s identity over time, adding an additional layer of security that is difficult to replicate or forge.
6. Anti-spoofing Measures
AI implements advanced anti-spoofing techniques to distinguish between real biometric traits and artificial replicas or masks, thereby enhancing the security against fraudulent access attempts.
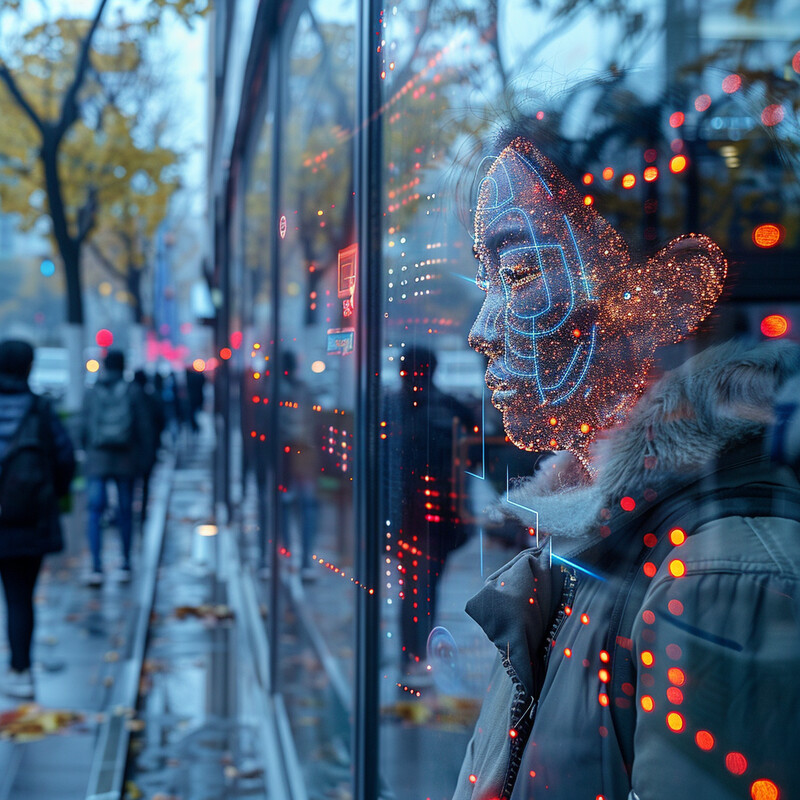
AI implements sophisticated anti-spoofing techniques to differentiate between genuine biometric markers and fake ones, such as masks or synthetic fingerprints. Using detailed analyses of texture, temperature, pulse, or even pore patterns, AI systems can effectively prevent fraudulent access attempts, significantly enhancing security.
7. Real-Time Decision Making
AI processes biometric data in real-time, allowing for instant authentication decisions that improve user convenience and system efficiency.
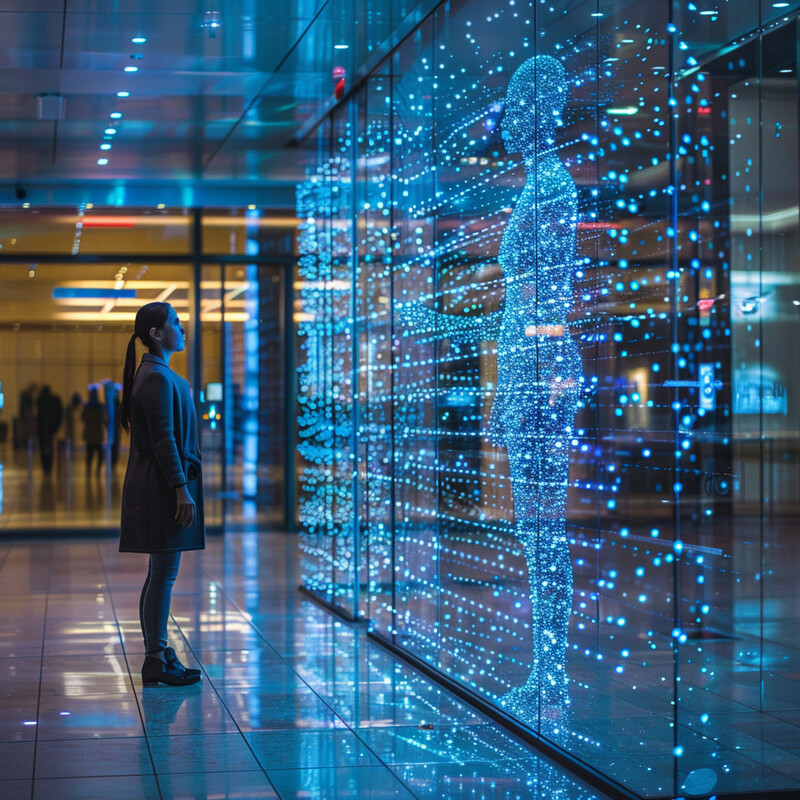
AI processes and authenticates biometric data in real-time, providing immediate feedback and decision-making capabilities. This speed is crucial for user convenience in high-traffic environments like airports or secure access buildings, where quick throughput is necessary without compromising security.
8. Integration with Other Security Measures
AI helps integrate biometric data with other security systems, such as two-factor authentication, to create multi-layered security architectures that are harder to breach.
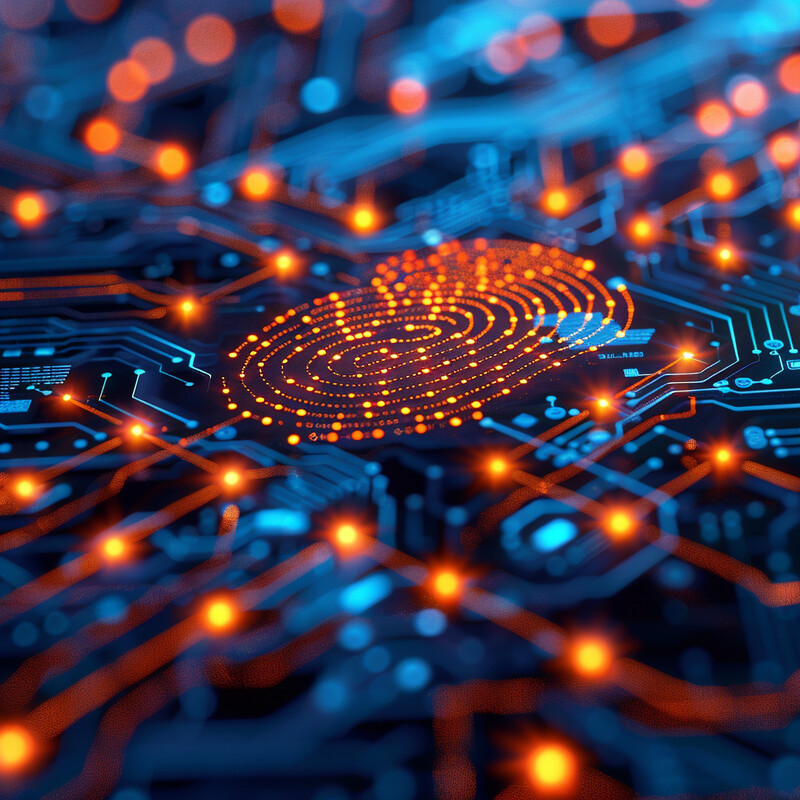
AI facilitates the integration of biometric authentication with other security protocols, such as two-factor authentication systems. For instance, combining a biometric check with a password or security token offers a multi-layered defense that significantly enhances overall system security.
9. Continuous Authentication
AI enables continuous authentication by constantly verifying the user’s identity in the background during sessions, increasing security for sensitive applications.
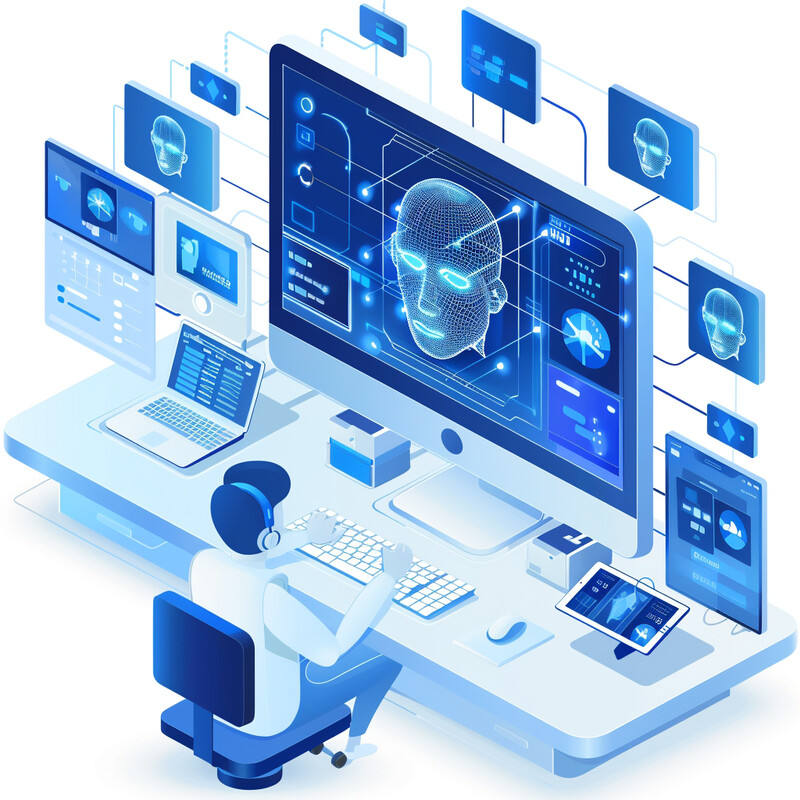
AI enables continuous authentication, where the system doesn't just check identity at the login stage but continuously verifies the identity of the user throughout a session. This method is particularly useful in high-security environments, ensuring that the system remains secure even if the initial authentication factors are compromised.
10. Personalization of User Experience
AI uses biometric data not only for authentication but also to personalize user interfaces and services based on the identified user, enhancing both security and user experience.
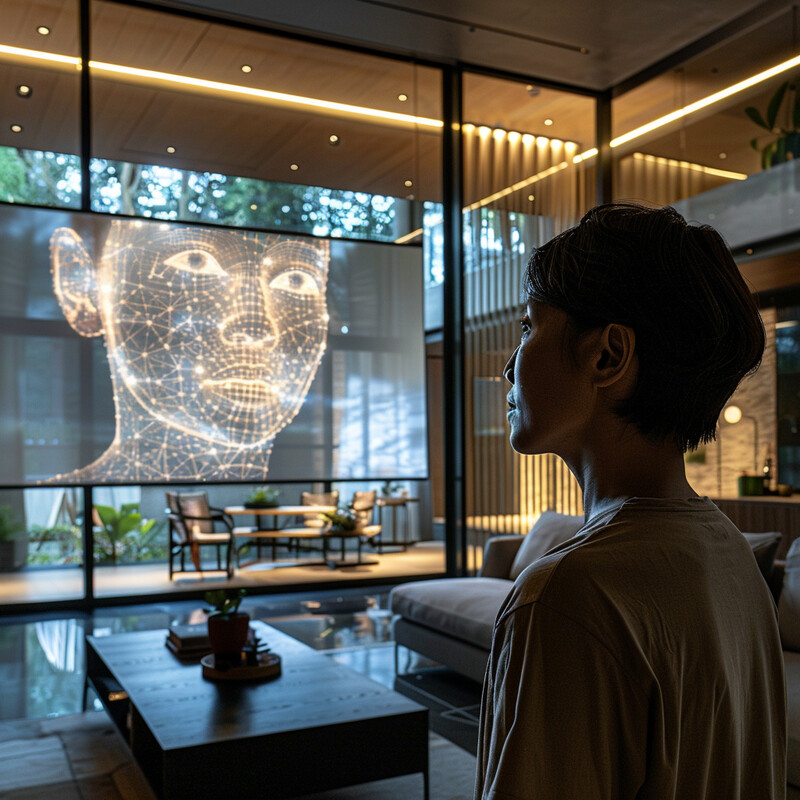
Beyond security, AI uses biometric data to personalize user experiences. Once a user is identified, systems can adjust interfaces, preferences, and functionalities to match the user’s established habits and preferences, enhancing usability and satisfaction.