1. Enhanced Perception Systems
AI algorithms process data from cameras, lidar, and radar to accurately perceive surroundings, including other vehicles, pedestrians, obstacles, and road signs, allowing for safer navigation.
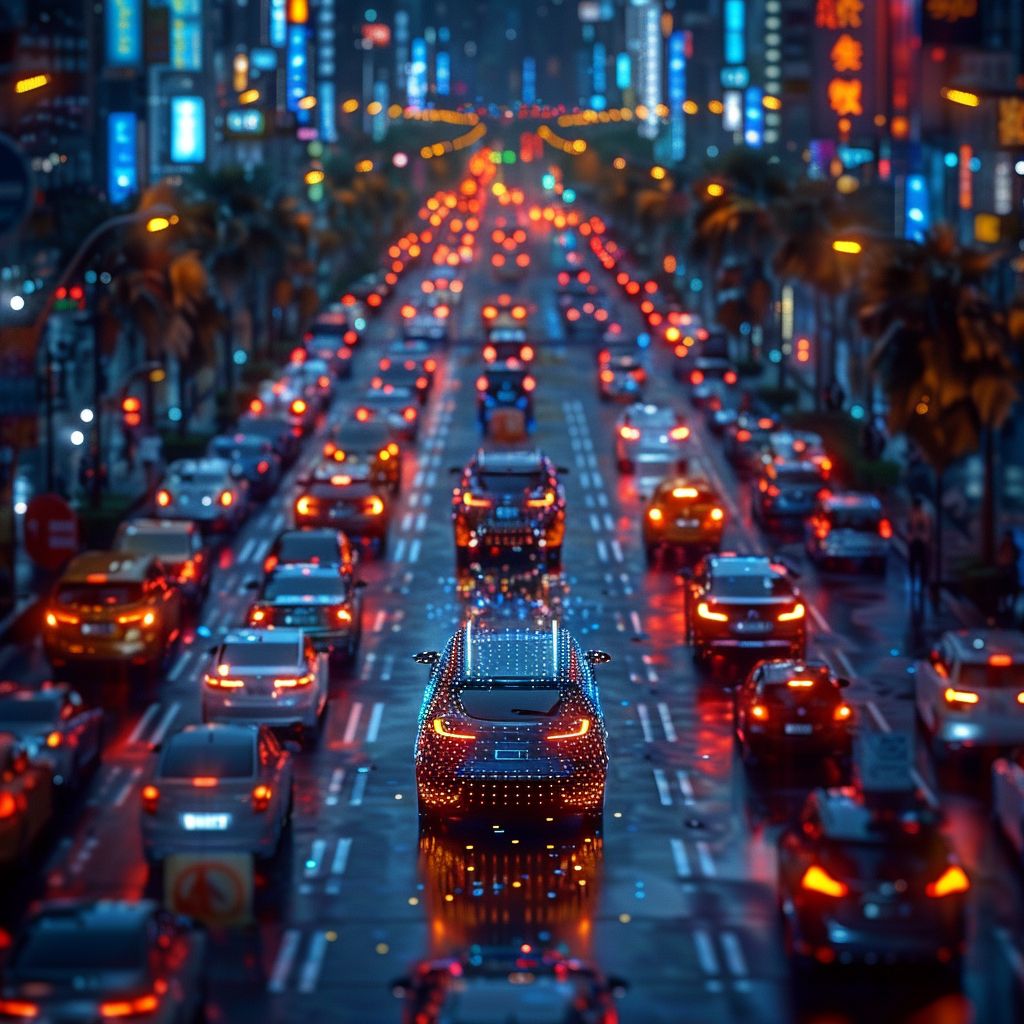
AI-driven perception systems in autonomous vehicles utilize a combination of sensors like cameras, lidar (light detection and ranging), and radar to create a detailed understanding of the vehicle's surroundings. These systems process vast amounts of data to identify objects, track their movements, and predict future positions. This allows the vehicle to navigate complex environments safely, recognizing everything from other vehicles and pedestrians to road signs and lane markings. AI algorithms continuously learn from new data, improving their accuracy and reliability over time.
2. Improved Decision Making
AI enables vehicles to make complex decisions in real-time, such as when to merge into traffic, how to navigate intersections, or how to react to sudden changes like a car abruptly stopping ahead.
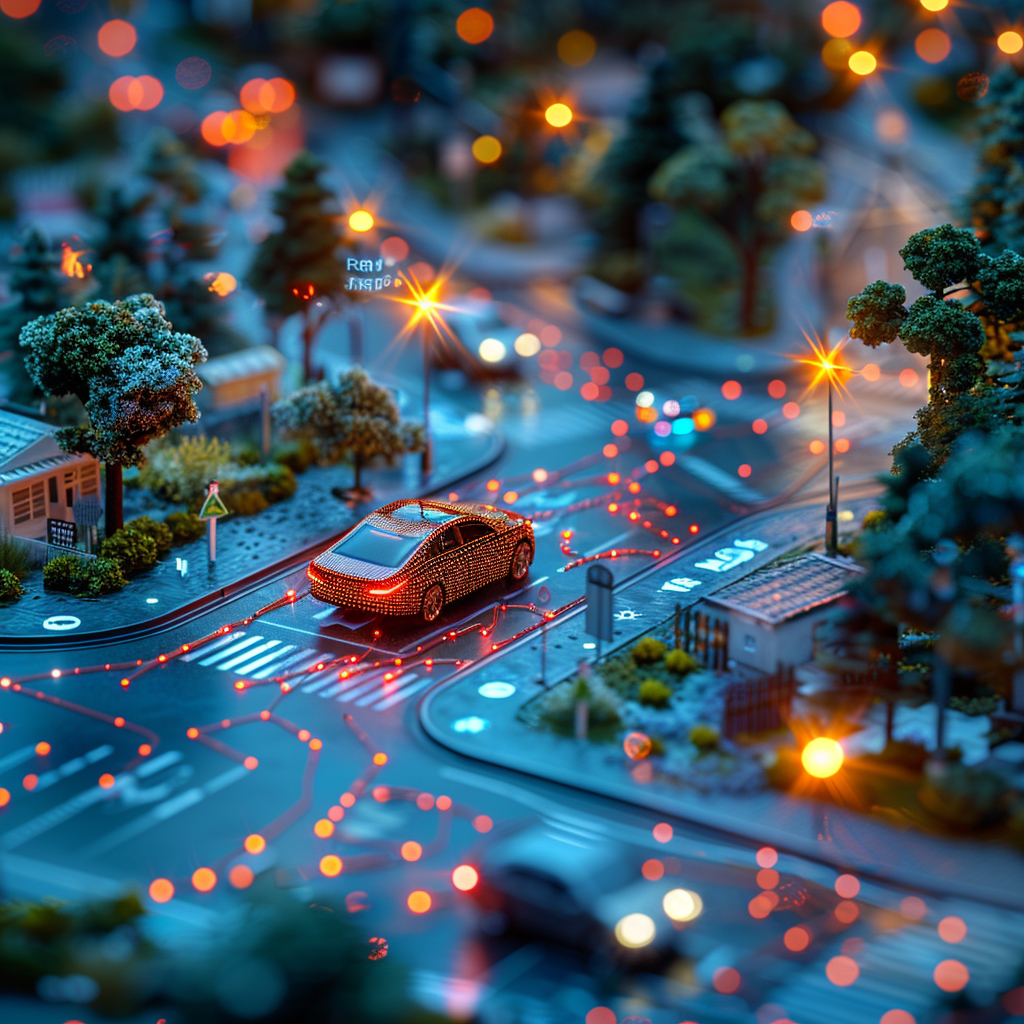
Autonomous vehicles rely on AI for decision-making processes that mimic a human driver's reasoning but with greater speed and precision. AI evaluates multiple possible actions and predicts their outcomes, choosing the safest and most efficient course of action. This capability is crucial in dynamic environments, such as urban settings where sudden events, like a car stopping unexpectedly or a pedestrian stepping onto the road, require immediate and smart decision-making to avoid accidents.
3. Predictive Capabilities
Through machine learning, autonomous vehicles can predict the actions of other road users (like whether a pedestrian will cross the road or a car will change lanes) and adjust their own actions proactively.
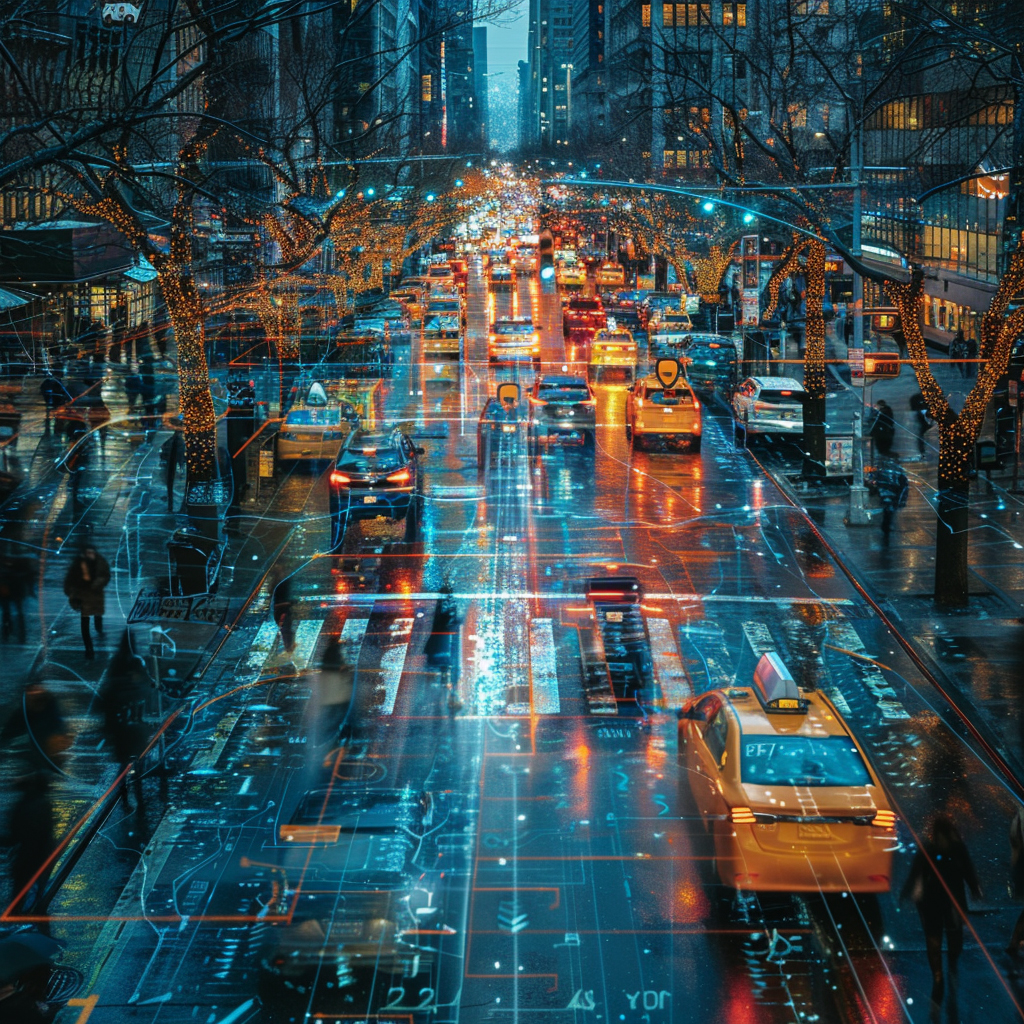
AI enhances the predictive capabilities of autonomous vehicles, enabling them to anticipate the actions of other road users. By analyzing historical data and real-time inputs from the vehicle's sensors, AI models can predict whether a nearby vehicle is likely to change lanes or if a pedestrian might cross the street. This foresight allows the autonomous vehicle to make preemptive adjustments to its speed or trajectory, enhancing safety and smoothness in travel.
4. Optimal Route Planning
AI integrates real-time data such as traffic conditions, road closures, and weather information to determine the most efficient routes, reducing travel time and improving fuel efficiency.
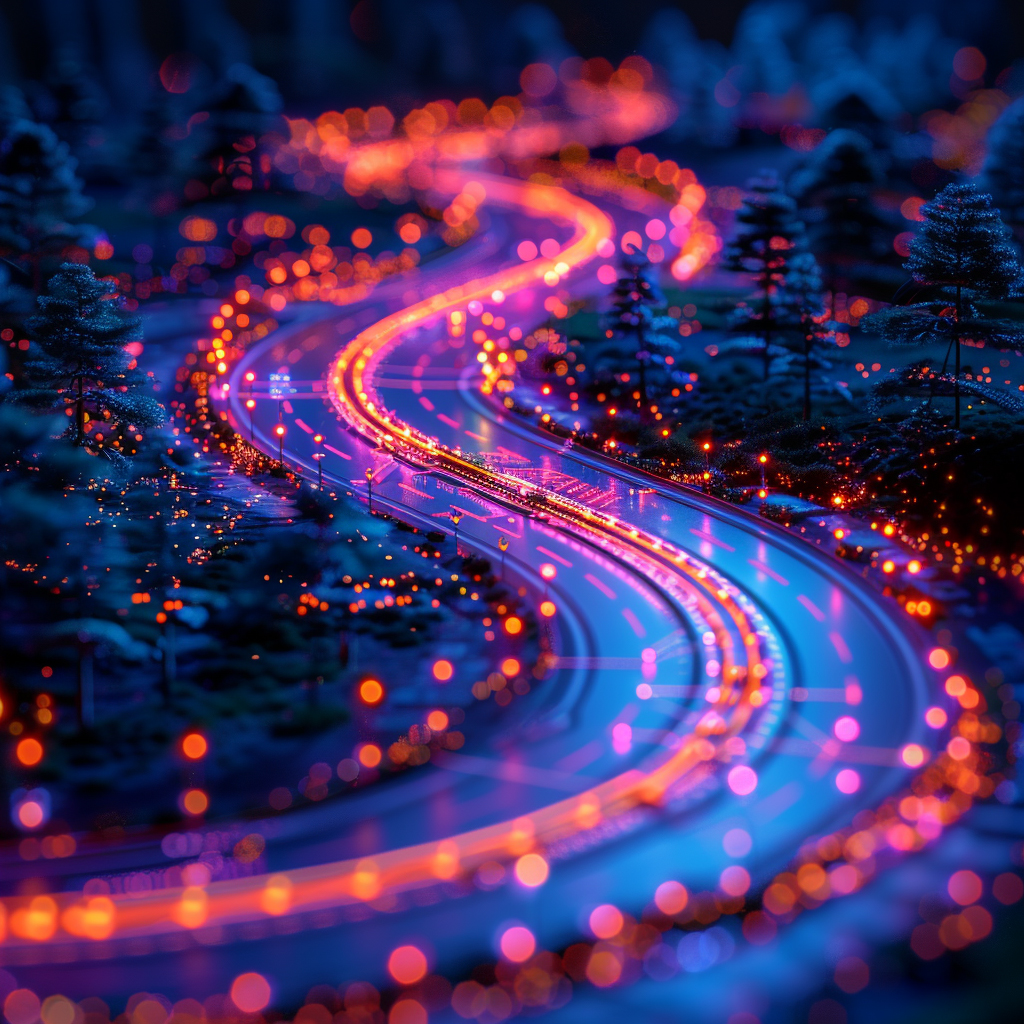
AI optimizes route planning by processing data from various sources, including traffic updates, weather conditions, and known roadworks. It calculates the fastest or most energy-efficient route to a destination, considering current and predicted conditions. This not only saves time but also reduces wear and tear on the vehicle and lowers fuel consumption or battery use, which is especially important for electric vehicles.
5. Adaptive Cruise Control
This AI-driven feature adjusts the vehicle's speed in response to the behavior of cars ahead, maintaining a safe distance and adapting to the flow of traffic without driver input.
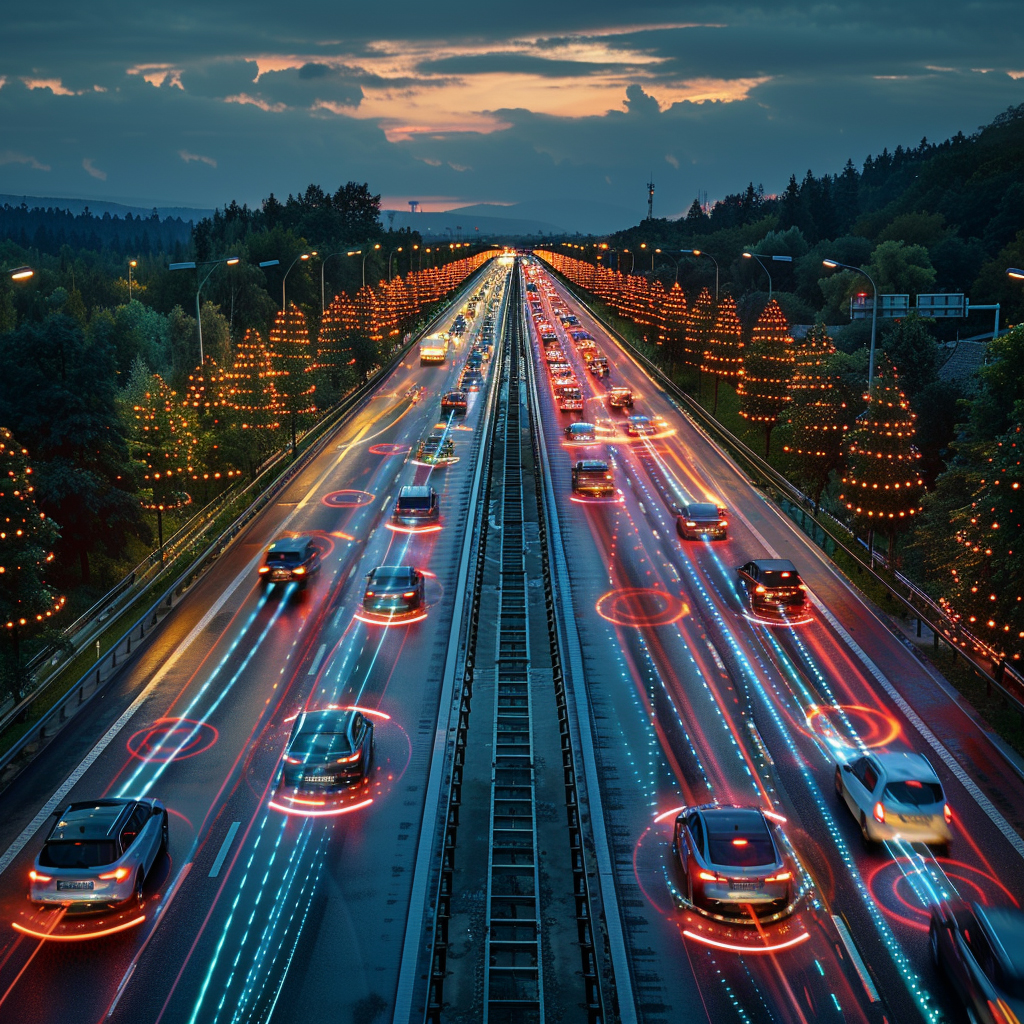
Adaptive Cruise Control (ACC) is an AI-enhanced system that autonomously adjusts the vehicle’s speed to maintain a safe following distance from the car ahead. It uses sensor inputs to monitor the speed and distance of vehicles in front and can accelerate or decelerate accordingly. This system is particularly useful on highways, where it can adapt to changing traffic speeds, enhancing both comfort and safety.
6. Lane Keeping Assistance
AI systems analyze road markings and the vehicle’s position to assist in keeping the vehicle centered in its lane, providing corrections if the vehicle begins to drift.
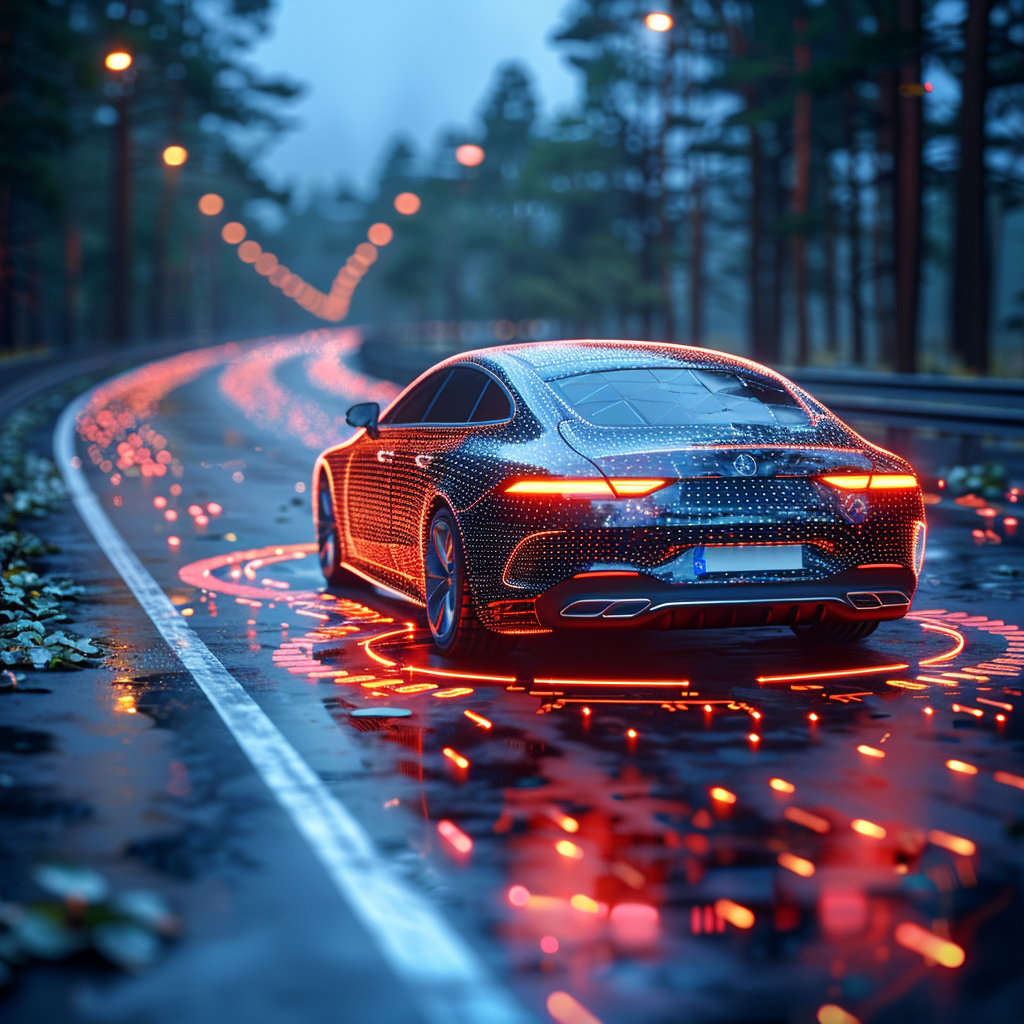
Lane Keeping Assistance (LKA) systems use AI to analyze visual data from cameras to detect lane markings and the vehicle's position relative to them. If the system detects that the vehicle is drifting without signaling, it will automatically make minor steering adjustments to help maintain the vehicle’s lane position. This feature is a critical safety mechanism that prevents accidents caused by drifting due to driver inattention or fatigue.
7. Traffic Sign Recognition
AI can recognize and interpret traffic signs, allowing the vehicle to adjust its speed according to speed limits and comply with other road regulations automatically.
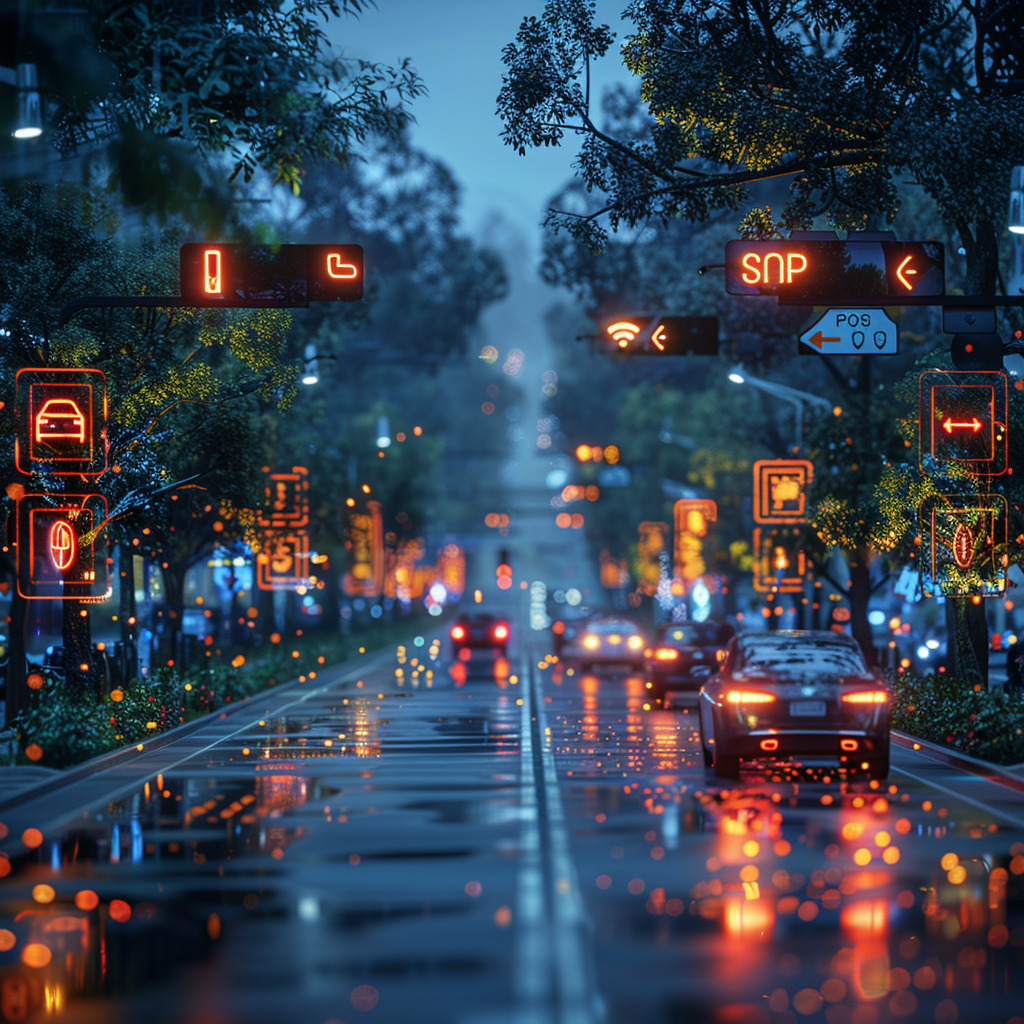
AI in autonomous vehicles can recognize and understand traffic signs using image recognition technologies. This capability enables the vehicle to comply with speed limits, yield signs, stop signs, and other traffic directives automatically. By adhering to these rules, the vehicle ensures compliance with legal standards and maintains safety for all road users.
8. Condition Monitoring
AI monitors the vehicle’s operational status in real-time, predicting maintenance needs and potential system failures before they become problematic, thus ensuring the vehicle’s reliability and safety.
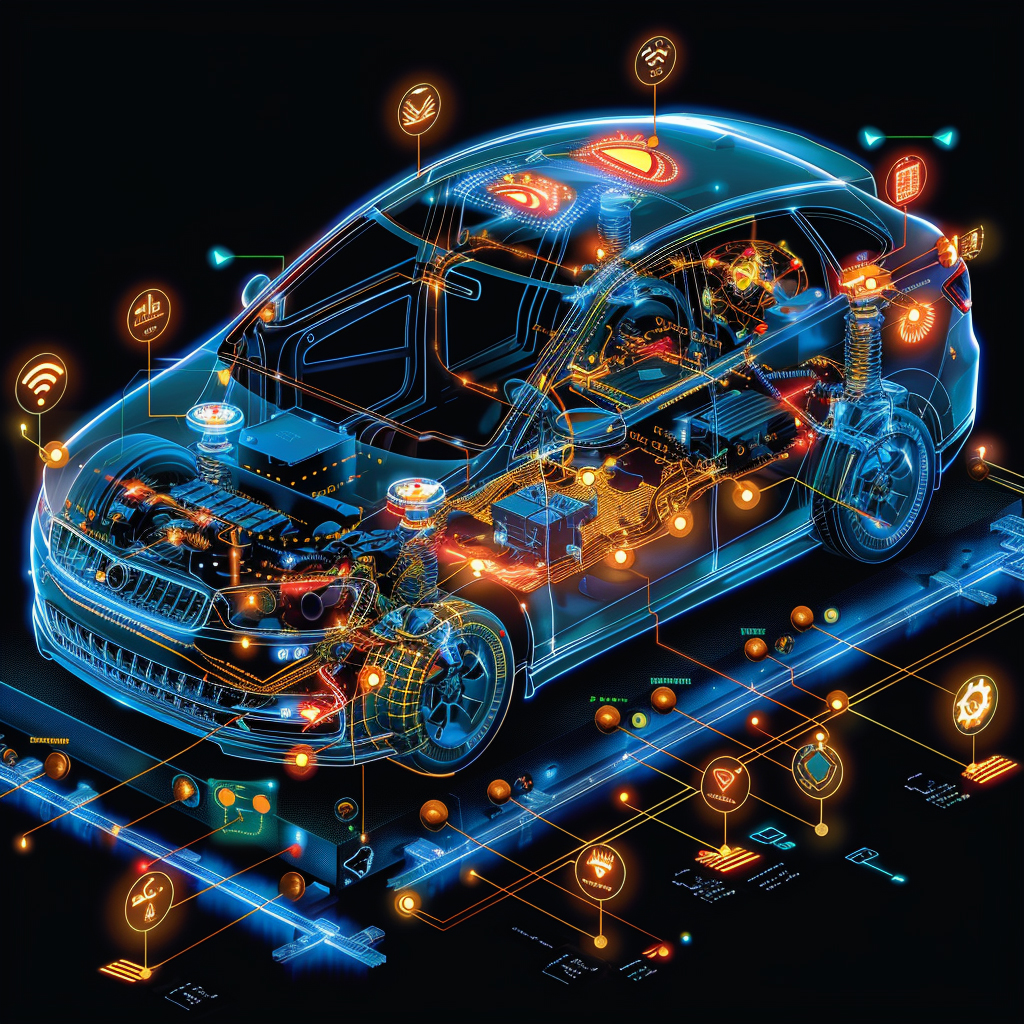
Condition monitoring through AI involves the continuous analysis of the vehicle's mechanical and electronic systems to predict and diagnose issues before they lead to failure. This predictive maintenance can significantly reduce downtime and repair costs. AI algorithms can detect anomalies in behavior that might indicate a problem, allowing for timely interventions that can extend the vehicle's life and improve safety.
9. Enhanced Security Features
AI algorithms help in detecting and responding to security threats, such as unauthorized access or hacking attempts, which are critical for the safety of autonomous vehicles.
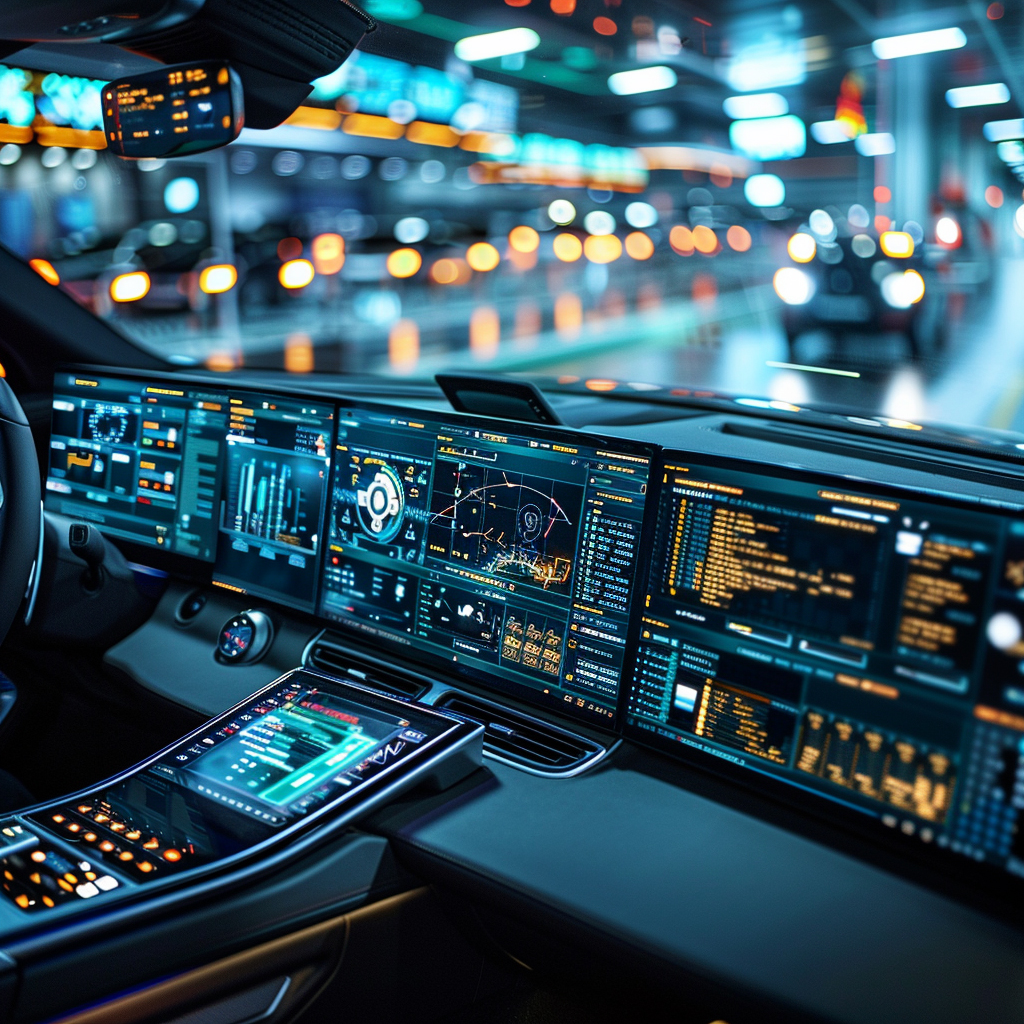
Security is paramount in autonomous vehicles to protect against hacking and other unauthorized interventions. AI algorithms can detect patterns indicative of cyber attacks and can initiate defensive protocols automatically. This proactive security helps ensure the integrity of the vehicle’s operational systems, safeguarding both the vehicle and its passengers.
10. Driver Monitoring
In semi-autonomous vehicles, AI can monitor driver attentiveness and readiness to take control of the vehicle if needed, ensuring a safe handover process between the vehicle and human driver.
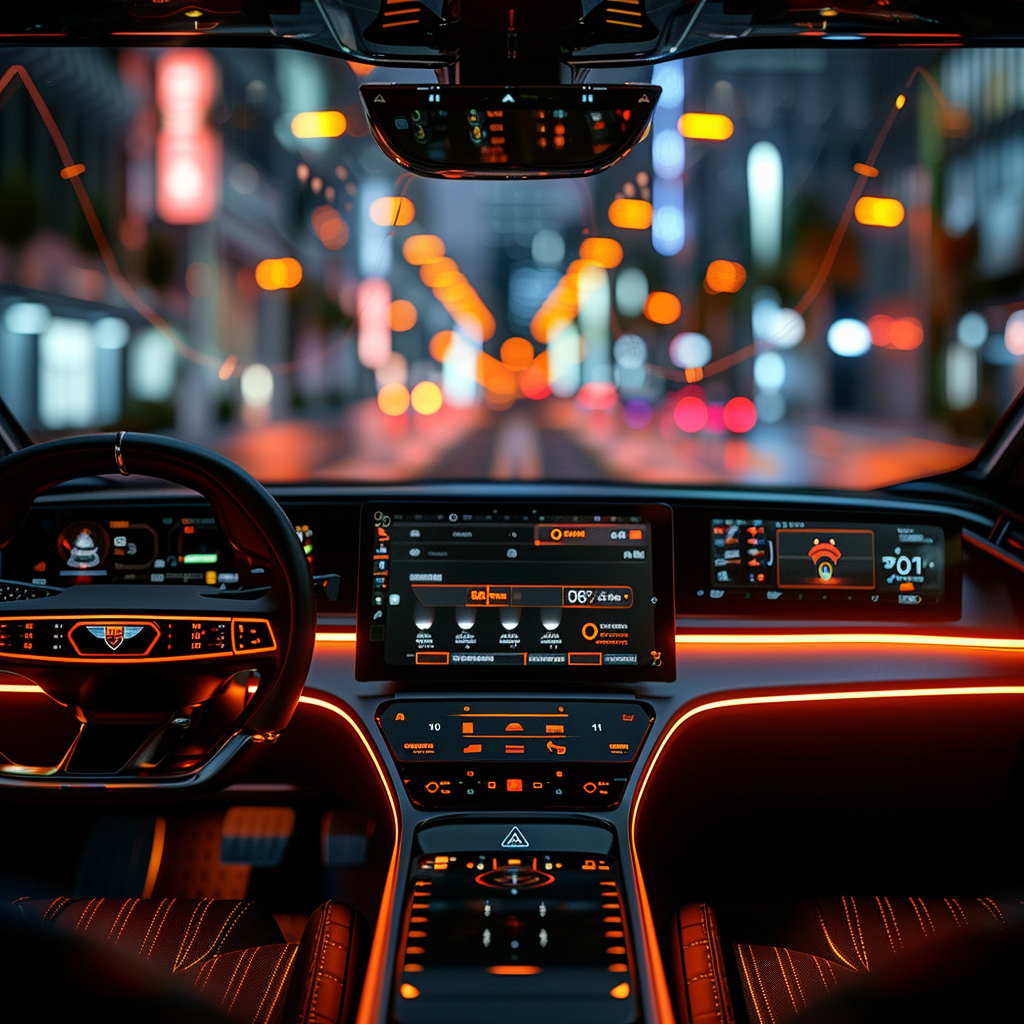
In scenarios where human drivers may need to take control from the autonomous system, AI-driven driver monitoring systems become essential. These systems use sensors and cameras to assess the driver's alertness and readiness to engage. If the driver appears distracted or sleepy, the system can alert them or take precautionary measures to maintain safety. This blending of AI and human input ensures a safer transition and operation in semi-autonomous vehicles.