1. Personalized Content Recommendations
AI-driven personalization is now core to content platforms and e-commerce, tailoring what each user sees based on their behavior and preferences. Services like streaming sites and online retailers use algorithms to recommend movies, articles, or products that an individual is likely to enjoy. These systems improve user engagement by keeping audiences on-platform longer and increasing click-through rates. The technology leverages browsing history, past interactions, and even demographic data to predict what content will resonate. By delivering more relevant choices, AI personalization enhances user satisfaction and loyalty in real-world applications (for example, many users find much of their Netflix or Amazon content via AI recommendations rather than manual search).
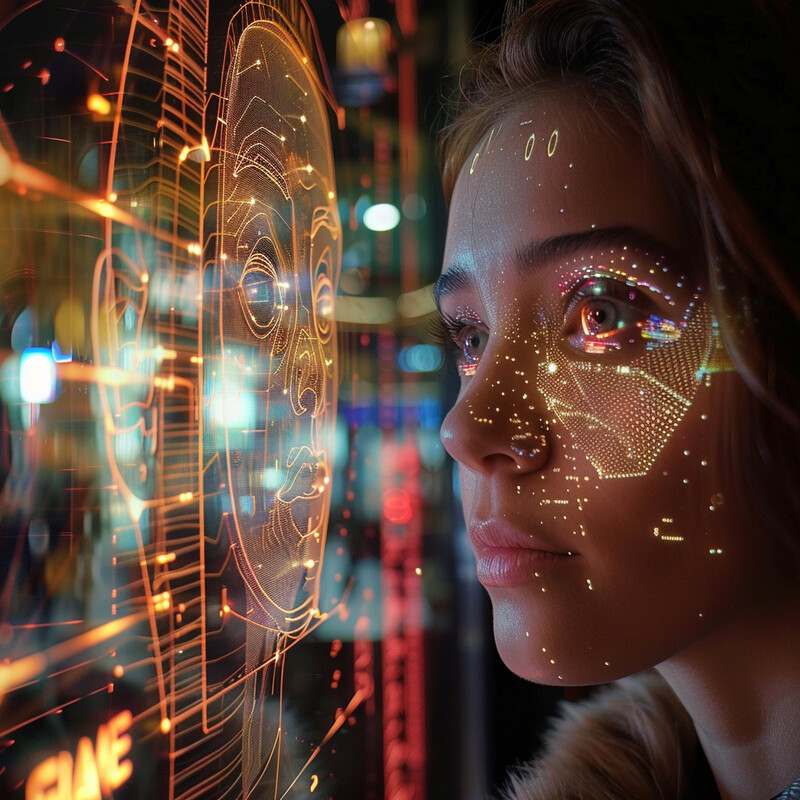
Major companies report substantial portions of their engagement and revenue coming from AI-driven recommendations. Amazon, for instance, attributes an estimated 35% of its e-commerce sales to its recommendation engine, which suggests products based on user behavior. In the media realm, Netflix’s personalized recommendation system is so effective it saves the company roughly $1 billion each year, by keeping viewers watching content they enjoy and reducing subscriber churn. These figures underscore how AI-curated content significantly drives user activity. More broadly, a recent McKinsey study noted that 71% of consumers now expect companies to deliver personalized interactions, and 76% get frustrated when this doesn’t happen. When personalization is done right, companies see measurable lifts – McKinsey found 10–15% higher conversion rates from more personalized customer experiences. In short, tailoring content through AI has moved the needle on engagement and sales across industries.
2. Automated Customer Support
AI-powered customer support—such as chatbots and virtual agents—is increasingly handling routine inquiries on websites and messaging channels. These systems can instantly answer frequently asked questions, help users navigate services, or even process simple transactions. By providing 24/7 assistance with zero wait time, AI support tools keep customers engaged and resolve issues faster than traditional queues. Importantly, modern AI chatbots use natural language processing to understand a wide range of user requests (often in conversational language) and continuously improve from interactions. This has allowed businesses to scale up support during peak times without overwhelming human staff. Overall, automated support enhances the user experience by being always available and immediately responsive, which in turn boosts customer satisfaction and frees human agents to tackle more complex problems.
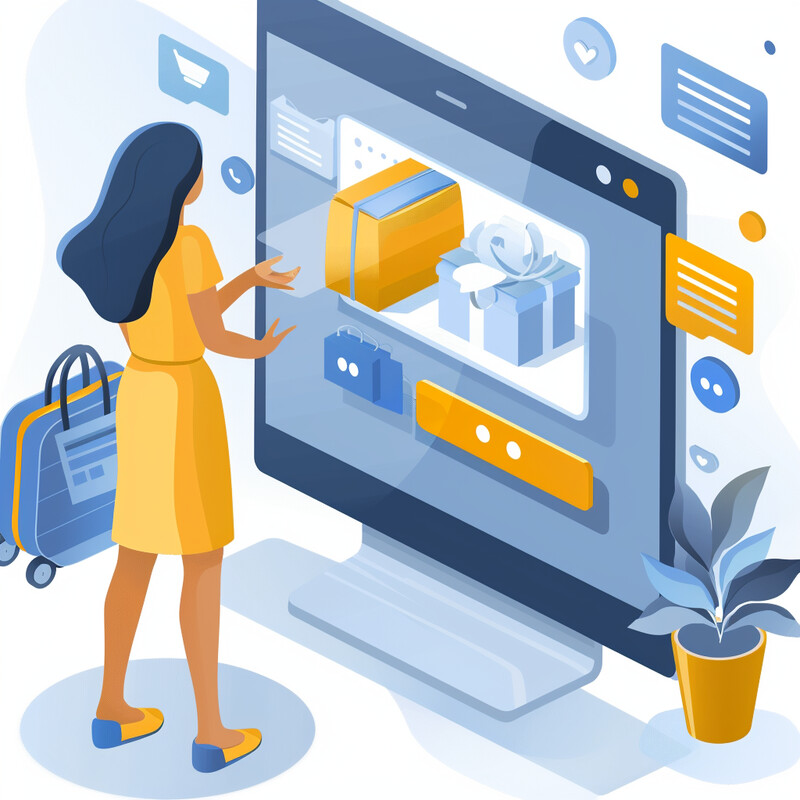
Adoption of AI chat support has become mainstream for both consumers and businesses. In the United States, roughly 49% of adults have interacted with an AI-powered chatbot for customer service within the past year. Companies report positive impacts as well: nearly 75% of U.S. business owners in 2023 said that AI chatbots (e.g. via instant messaging) improved their customer experience by providing timely, helpful responses. Efficiency gains are notable – industry analyses find that companies using AI chat agents can reduce customer service costs by up to 30% while improving response times. Additionally, surveys show more than half of consumers actually prefer interacting with bots when they want instant service, rather than waiting for a human. These trends highlight how AI-driven customer support is meeting audience expectations: providing quick answers around the clock, resolving common issues immediately, and escalating complex issues to humans when needed. As a result, businesses deploying chatbots have seen higher customer satisfaction scores (up 24% in one study) and faster resolution rates for routine inquiries.
3. Sentiment Analysis
Sentiment analysis tools use AI to analyze customer opinions and emotions across social media, reviews, and feedback in real time. By processing text (and sometimes speech) through natural language understanding, these systems determine whether the sentiment expressed is positive, negative, or neutral. In practice, companies leverage sentiment analysis to gauge how audiences feel about a product launch, a piece of content, or the brand overall. This provides immediate insight into public perception at scale – far beyond what manual monitoring could achieve. Tech professionals incorporate sentiment dashboards to alert them to spikes in negative sentiment (potentially signaling an issue or PR crisis) or to measure the impact of campaigns. Overall, AI sentiment analysis helps businesses stay tuned into audience morale and react quickly: for example, by addressing common complaints, adjusting messaging, or thanking customers for positive responses. This data-driven emotional pulse of the audience informs strategy and leads to more empathetic, audience-centered engagement.
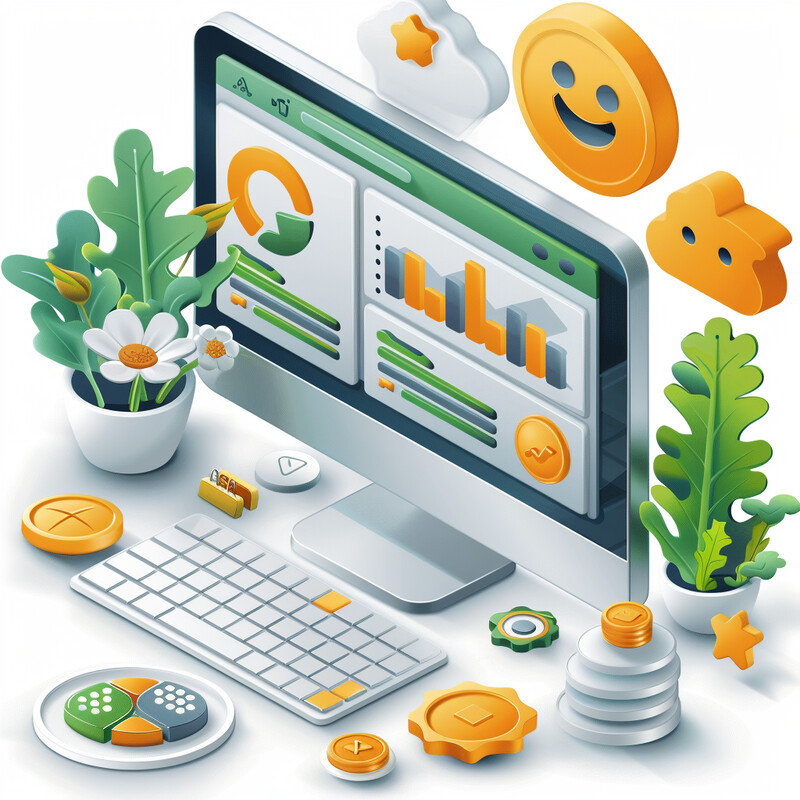
Listening to audience sentiment yields measurable benefits for customer relations. A 2023 study by McKinsey found that companies “excellent” at social listening (including sentiment analysis) had a 17% higher customer satisfaction rate than companies that didn’t focus on these feedback channels. Similarly, responsiveness guided by sentiment data can significantly boost goodwill. According to social media analytics data, businesses that respond to negative comments within one hour see up to a 70% increase in customer satisfaction compared to those that respond later or not at all. These outcomes show why sentiment analysis matters: brands can swiftly identify and resolve pain points voiced by customers, turning around negative experiences. In one survey, 79% of consumers said they appreciate when companies actively monitor and address customer feedback online, as it makes them feel heard. By using AI to parse thousands of social posts and reviews for sentiment, organizations can maintain a positive brand image, respond to emerging issues before they escalate, and ultimately foster stronger loyalty through responsive engagement.
4. Predictive Analytics
Predictive analytics uses AI and machine learning to forecast future user behaviors based on historical data. In audience engagement, this means systems can predict which customers are likely to churn, which users might click on which content, or what products will trend next month. These insights allow companies to be proactive: for example, a streaming service might pre-load recommended videos it predicts a user will watch, or a marketing team might reach out with incentives to a subscriber who is flagged as likely to cancel. Predictive models digest countless signals (past purchases, time on site, past click patterns, etc.) to output probabilities for future actions. Tech professionals implement these models to drive decision-making – allocating resources toward likely high-impact opportunities and personalizing outreach on an individual level. Overall, AI-powered predictive analytics turns raw data into actionable foresight, helping businesses optimize engagement strategies (like targeted upsell offers or content placement) before user behavior happens, rather than reacting afterward.
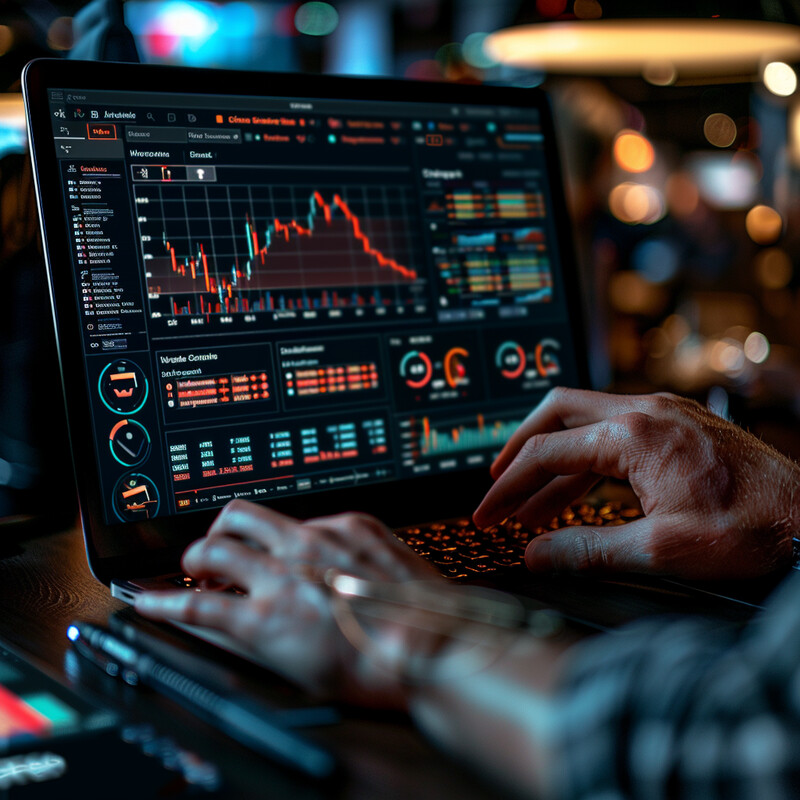
Organizations have rapidly embraced predictive analytics in marketing and customer engagement, and they’re seeing clear benefits. Over 60% of marketing professionals report using predictive analytics to enhance customer targeting and personalization efforts as of 2024. This data-driven foresight is linked to better retention and sales. Notably, Gartner analysis indicates that companies using predictive models to identify high-risk customers have improved those customers’ retention rates by roughly 10–15% through timely, targeted interventions. In one case study, a telecom firm used AI predictive scores to proactively offer promotions to subscribers about to lapse, reducing churn significantly versus a control group. Predictive analytics also boosts marketing ROI: researchers have found that businesses leveraging advanced analytics and AI for personalization achieve 5–8% higher marketing ROI than competitors. As a result, investment in predictive analytics is growing – surveys show about 78% of marketers plan to increase spending on AI analytics tools, recognizing that anticipating customer needs leads directly to better engagement and conversion.
5. Real-time Interaction Tracking
Real-time interaction tracking refers to AI systems continuously monitoring user actions as they happen – clicks, swipes, views, comments, etc. – and providing instant analytics or adjustments. In audience engagement tools, this means a dashboard can show, for example, how many people are reading an article right now and where they drop off, or a video platform can highlight that a certain segment of viewers is rewinding a particular part of a live stream. Tech teams use this instantaneous feedback to optimize content on the fly. If a live metric shows users are abandoning a webpage after a certain section, editors can tweak the content or layout immediately. Real-time A/B testing is another application: an AI might dynamically promote a trending topic on a homepage as it detects rising interest. The key advantage is agility – content creators and marketers aren’t waiting days or weeks for reports; they can respond to audience behavior in the moment. By iterating in real time, engagement tools ensure that what the audience is seeing is always as relevant and effective as possible, keeping users interested and reducing the chance of losing them to boredom or frustration.
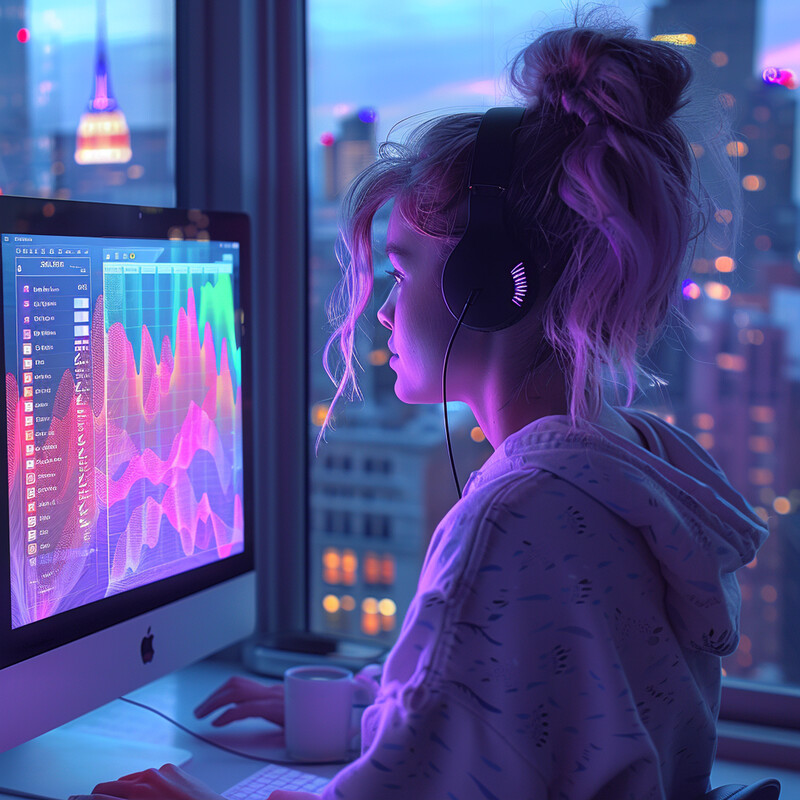
Operating on real-time analytics delivers proven business benefits. According to an IDC study, 79% of organizations that embraced real-time data analytics reported improved business outcomes, and on average saw a 15% increase in revenue within one year of implementation. This bottom-line impact comes from engaging customers more effectively in the moment. A striking example is Starbucks: by analyzing over 4 billion transactions in real time to personalize offers (such as recommending a drink based on current weather and past purchases), the company achieved a 25% increase in the use of its loyalty rewards program. In general, companies using real-time engagement data can react instantly – one online retailer, for instance, noted that when they began tweaking site content hour-by-hour based on live interaction data, their bounce rate dropped and time-on-site rose by a significant margin. Real-time tracking also helps pinpoint issues immediately. An e-commerce site could see a spike in drop-offs at checkout in real time and quickly investigate (and fix) a potential payment glitch, saving that session’s sales. Industry surveys confirm that organizations feel more agile: over 85% of businesses say real-time analytics has made them better equipped to make quick decisions that enhance customer experience on the fly.
6. Enhanced Social Media Management
AI is elevating how marketers manage social media by automating and optimizing content strategy. Modern social media management tools use AI to analyze engagement patterns (e.g. when followers are most active, which posts perform best) and recommend the optimal times and formats to post. They can also auto-generate social copy or hashtags based on trends, and even curate relevant content. These capabilities let social media teams maintain a consistent, high-quality presence with less manual effort. For instance, instead of manually checking analytics from each platform, an AI assistant might surface a daily brief: “Your video post is trending on Twitter – consider boosting it now.” AI can also monitor comments or brand mentions in real time, alerting managers to positive interactions to amplify or negative feedback to address. By handling everything from smart scheduling to sentiment-driven moderation suggestions, AI-assisted social media tools help brands be more responsive, data-driven, and efficient in their social engagement, which typically translates to broader reach and higher user interaction.
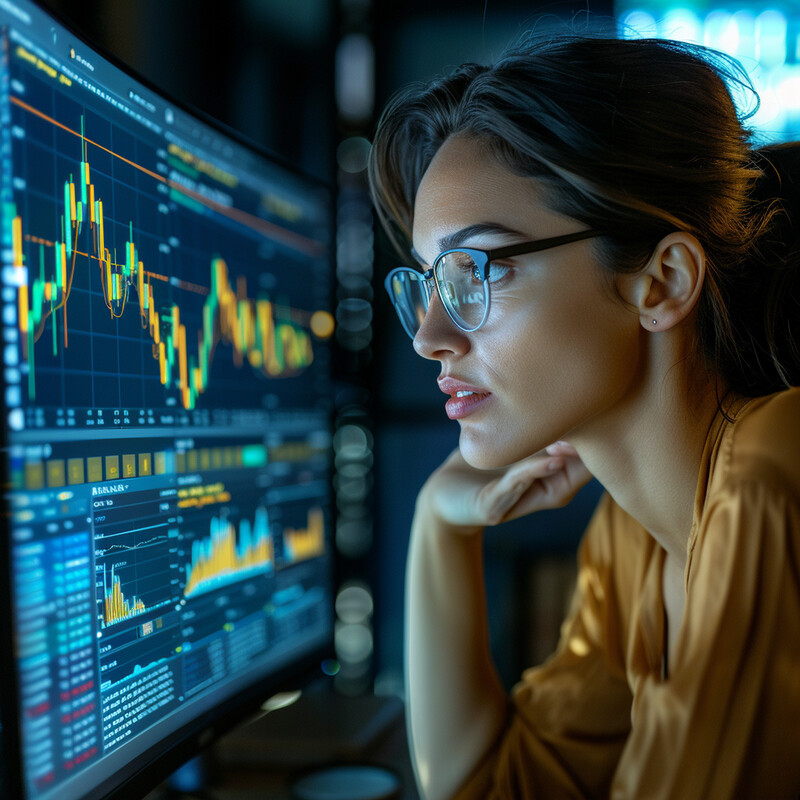
The use of AI in social media marketing has grown rapidly in the past couple of years. By 2024, approximately 40% of marketers and social media managers reported using AI tools in their social media workflows, whether for content scheduling, social listening, or competitor research. These practitioners cite efficiency and performance gains as a result. In one industry survey, 38% of marketers said that increased efficiency was the primary benefit of using AI in social media marketing. Concretely, brands leveraging AI-driven scheduling and optimization have seen engagement upticks – for example, an internal analysis by LinkedIn found that posts made at AI-recommended times yielded significantly higher impressions and interactions than those posted at random times. Moreover, AI can analyze large volumes of social data to inform strategy: a social media AI tool might determine that video posts outperform text posts for a particular audience, leading the team to shift its content mix and subsequently seeing higher engagement rates. In practice, companies using AI for social media have been able to increase posting frequency by 2-3x without added staff, while maintaining or improving engagement metrics, because the AI handles much of the heavy lifting (content suggestions, timing, and cross-platform optimizations).
7. Dynamic Email Campaigns
AI is making email marketing more dynamic by personalizing emails at the individual recipient level and optimizing campaigns in real time. Traditionally, email campaigns were one-size-fits-all or segmented into a few broad groups. Now, AI can tailor nearly every element of an email: subject lines are A/B tested and refined for each user, product recommendations within the email are chosen based on the recipient’s browsing history or past purchases, and even send times are optimized (AI might send an email to Jane at 7:30 AM because it learned she tends to open emails in the morning, whereas John’s goes out in the evening). These dynamic adjustments significantly improve the relevance of marketing emails. AI can also automate the trigger of emails based on user behavior – for example, sending a follow-up with a discount if someone browsed a product but didn’t purchase. All of this leads to emails that feel more like a one-on-one communication than a mass blast. For tech professionals, AI-driven email platforms mean less manual segmentation and more trust in the system to “learn” what content and timing works best for each subscriber, ultimately driving higher open and click rates.
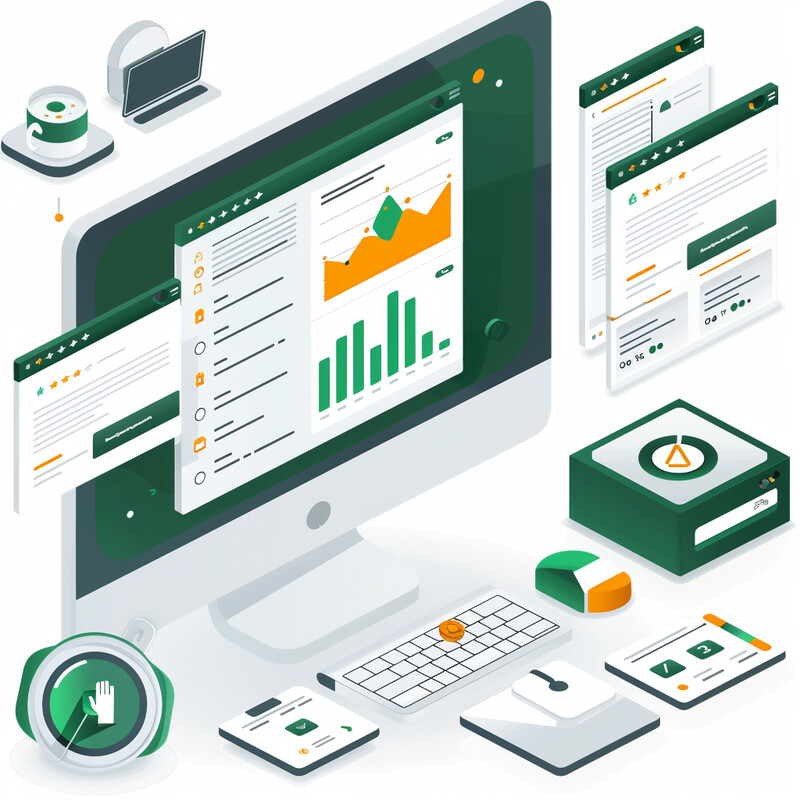
Email marketers are quickly adopting AI to boost engagement metrics. A recent industry survey found that nearly 49% of email marketers now use AI to assist with email content creation and personalization. The impact on performance is significant. In controlled tests, AI-driven email campaigns have achieved open rates up to 41% higher than similar campaigns without AI personalization. Even more modest AI interventions show clear gains – for example, marketing studies show that emails with AI-generated subject lines see about a 5–10% higher open rate compared to human-written subject lines alone. These improvements are attributed to AI’s ability to craft more compelling, personalized messaging and send it at the optimal time for each user. Click-through rates benefit as well: one analysis by G2 found that using AI for email personalization led to roughly a 13% increase in email click-through rates on average. Companies also report efficiency gains, with AI handling what used to be manual tasks like list segmentation or A/B testing – in turn, marketing teams can run more frequent campaigns (or more variants) without additional workload. Overall, the data demonstrates that AI-optimized email campaigns both engage more readers (getting more people to open and read) and drive more action from those readers, compared to traditional static email strategies.
8. Behavioral Targeting in Advertising
Behavioral targeting in advertising refers to using AI to serve ads to the users most likely to be interested, based on their past online behavior. Instead of showing the same ad to millions of people, AI algorithms analyze things like browsing history, purchase patterns, search queries, and demographic data to decide who should see which ad. This makes advertising far more relevant to individuals – for example, if someone has been reading articles about electric cars, an AI system might place an EV advertisement in their social media feed, whereas their neighbor who was shopping for pet food might see a pet supplies ad instead. For tech professionals, implementing behavioral targeting involves using programmatic ad platforms that automatically bid on and place ads in real time when a target user is detected (within milliseconds of a webpage loading). The net effect is a more efficient use of ad spend, as impressions are concentrated on audiences with higher propensity to engage or convert. Users also benefit by seeing ads that align more closely with their interests. Behavioral targeting is a pillar of modern digital advertising, and it’s almost entirely powered by AI models that learn which behaviors predict interest in which products.
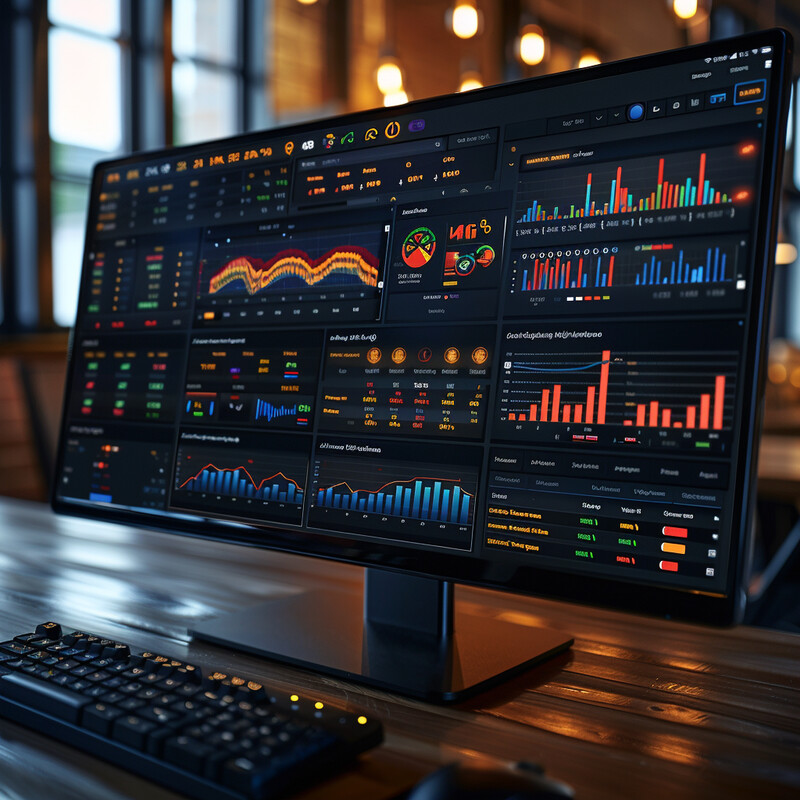
AI-driven behavioral targeting has essentially become the norm in digital advertising due to its effectiveness. In the United States, over 91% of all digital display ad spending in 2024 is flowing through programmatic channels that utilize AI algorithms to target ads to specific audience segments. This AI-centric approach vastly outperforms the old “spray and pray” method of broad advertising. Research by IDC found that conversion rates can increase by up to 60% when campaigns are hyper-personalized using AI-driven behavioral data, compared to traditional non-targeted campaigns. In other words, advertisements get significantly more people to take action (like clicking or purchasing) when AI ensures those ads are shown to receptive users. Another example: a case study in the travel industry showed that an AI-targeted ad campaign achieved a 2.5x higher click-through rate than the same creative sent without behavioral targeting, because the AI honed in on users already expressing travel intent. The efficiency gains are also clear – by reducing wasted impressions on disinterested viewers, companies have reported improving their return on ad spend (ROAS) by 20–30% after implementing machine-learning-based ad targeting. As these statistics illustrate, AI-fueled behavioral targeting makes advertising more effective for advertisers and more relevant for consumers, which is why it now dominates digital ad delivery.
9. Interactive and Gamified Content
Interactive and gamified content transforms passive audience experiences into active ones – the user is not just reading or watching, but participating. This can take the form of quizzes (e.g., “Which product is right for you?” questionnaires), polls, interactive infographics, or even mini-games embedded in a webpage. By engaging users in a two-way interaction, this content naturally holds attention longer and often provides a sense of fun or competition. Brands use gamification elements like points, badges, or challenges to motivate continued participation (for instance, a news site might have a trivia game that rewards the user with a badge for a perfect score). For tech builders, adding interactivity can be as simple as including a poll in a blog post or as complex as developing a custom game. The goal is to make the audience feel involved and in control, turning engagement from passive consumption to an immersive experience. This strategy has been shown to increase retention of information and the likelihood of content being shared, as users often like to show off their quiz results or challenge friends, amplifying reach.
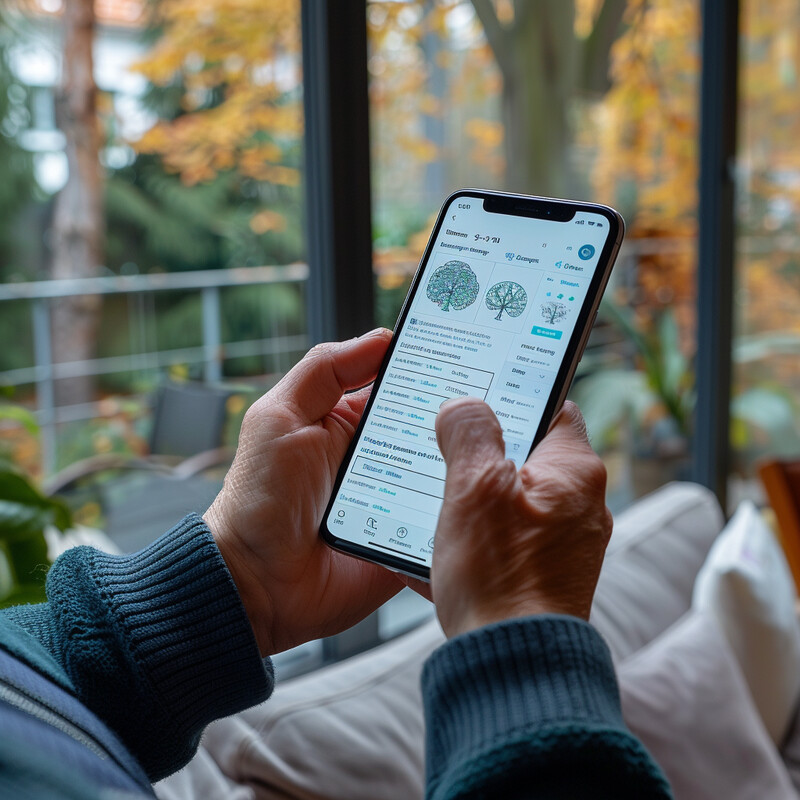
Interactive content delivers markedly better engagement metrics than static content. In one analysis of B2B marketing assets, interactive content achieved 52.6% more engagement on average than static content covering the same topics. Users also spent far longer interacting: the study found that people spent about 13 minutes with interactive content versus 8.5 minutes with static content, a roughly 53% increase in dwell time. These numbers came from a large sample (content engagement data via Mediafly) and demonstrate how adding quizzes, polls, or other interactive elements can captivate an audience. Marketers echo these findings – in a Content Marketing Institute survey, 81% of marketers agreed that interactive content (like polls, quizzes, surveys) is more effective at grabbing audience attention than static material. Moreover, interactive content often yields higher conversion rates: one case study noted that embedding a simple personality quiz on an e-commerce site generated x3 more leads than a standard sign-up form, because users were enticed to complete the fun quiz and then willingly provided their email to get results. Whether the goal is education, lead generation, or brand awareness, making content interactive clearly leads to deeper engagement and a more memorable user experience.
10. Accessibility Improvements
AI is dramatically improving digital accessibility, ensuring that people with disabilities can engage with content on equal footing and even enhancing the experience for all users. Key examples include automatic captioning and subtitling of videos (benefiting those who are deaf or hard of hearing, as well as anyone watching without sound), AI-generated image descriptions (so blind or visually impaired users using screen readers can know what an image contains), and real-time translations or sign language avatars. For tech professionals, implementing AI accessibility might involve integrating an API that transcribes audio to text on the fly or uses computer vision to describe images. These features used to require substantial manual effort (e.g., a human transcriber for captions or separate descriptive text for every image), but AI can perform them instantly and at scale. The result is more inclusive content that reaches a wider audience. And importantly, many accessibility improvements double as engagement boosts – for example, captions not only assist those with hearing loss, they also make videos more engaging in sound-off environments (like a user scrolling through social media in a quiet library). In summary, AI is helping make the web and media more inclusive by building assistive features into the content delivery, often automatically.
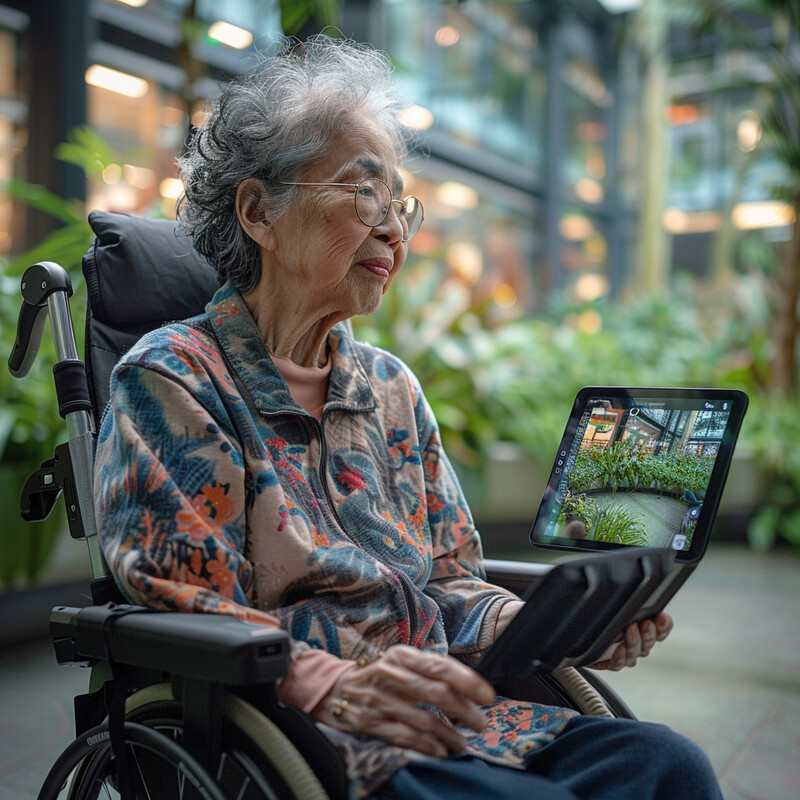
AI-driven accessibility features have measurable positive effects on user engagement. A joint study by Verizon Media and Publicis found that about 80% of viewers are more likely to finish watching a video if it has subtitles or closed captions. This highlights how captions (which AI can auto-generate) keep viewers engaged – by ensuring they can follow along in sound-off situations or if they have hearing impairments. In addition, experiments by Facebook showed that adding captions increases video view time: captioned video ads had an average 12% longer view time than ads without captions. The use of AI to generate captions has skyrocketed accordingly – a 2024 report noted a 254% increase in businesses adding closed captions to videos (using AI tools) compared to the year prior. Beyond captions, AI is also providing image alt-text at scale; for instance, Twitter’s AI automatically describes images in tweets, which saw millions of auto-alt-text tags generated, making the platform more navigable for blind users. These efforts not only comply with accessibility standards but also enhance the overall audience experience. TikTok’s data shows that captioned videos lead to a 58% improvement in content recall among viewers and significantly higher brand affinity. Such statistics reinforce that investing in AI-powered accessibility is a win–win: it broadens the audience who can consume the content and boosts engagement metrics by catering to user needs and preferences.