1. Site Detection and Mapping
AI algorithms analyze satellite imagery and aerial photographs to identify potential archaeological sites, helping researchers locate dig sites more efficiently.
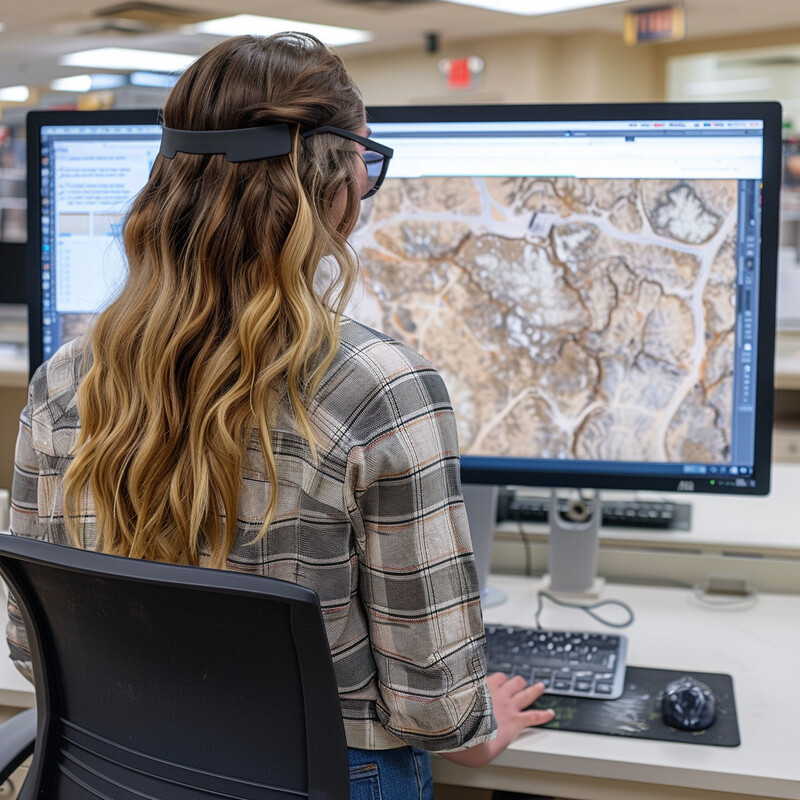
AI analyzes satellite images and aerial photography using advanced algorithms to detect anomalies in the landscape that may indicate the presence of archaeological sites. By processing large datasets rapidly, AI identifies patterns that are often imperceptible to the human eye, enabling archaeologists to pinpoint potential dig sites and plan expeditions more efficiently, thereby saving time and resources.
2. Artifact Analysis
AI aids in the classification and analysis of artifacts, using image recognition to quickly sort, identify, and catalog items based on shape, size, and patterns.
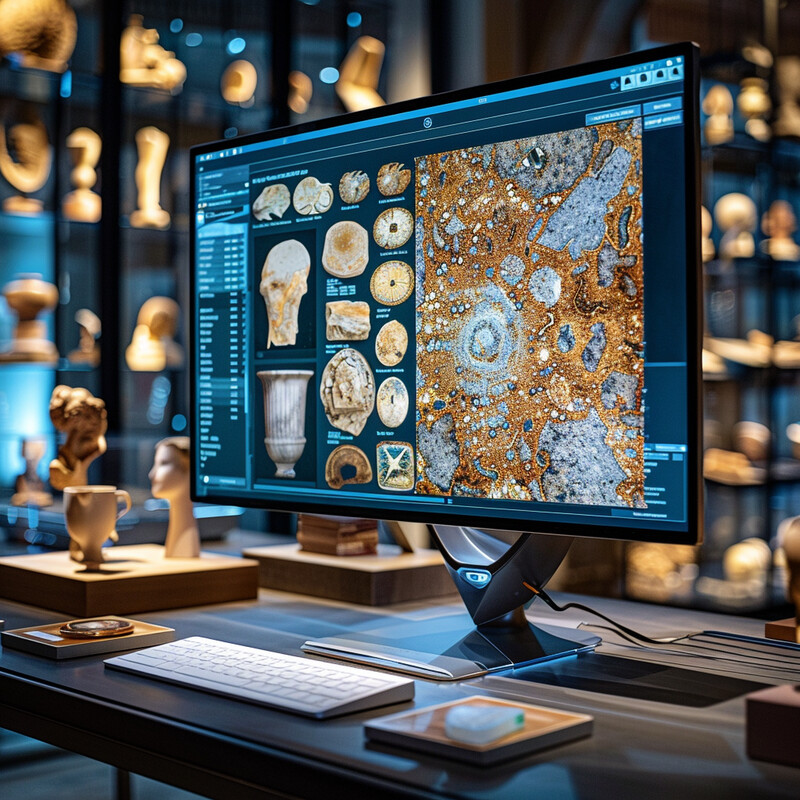
Using image recognition technologies, AI assists archaeologists in the classification and analysis of artifacts. It quickly sorts through large quantities of items, identifying and cataloging them based on distinctive features such as shape, size, material, and decoration patterns. This automation speeds up the initial sorting process and ensures a high level of accuracy in artifact classification.
3. Predictive Modeling of Site Locations
AI uses data from previous excavations and geographical information to predict where undiscovered archaeological sites might be located.
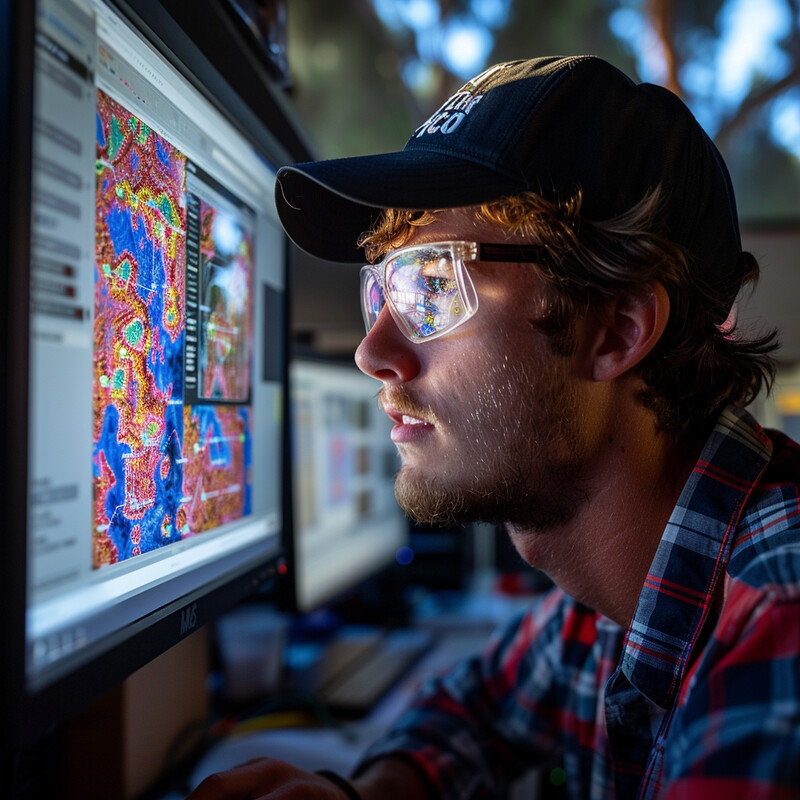
AI leverages data from previously discovered sites and integrates it with environmental and geographic information to model and predict the locations of yet-to-be-discovered archaeological sites. This predictive capability allows researchers to focus their efforts more strategically and increases the likelihood of successful discoveries.
4. Restoration of Artifacts
AI algorithms can predict the original appearance of damaged artifacts or structures, providing visual reconstructions that help in understanding historical contexts.
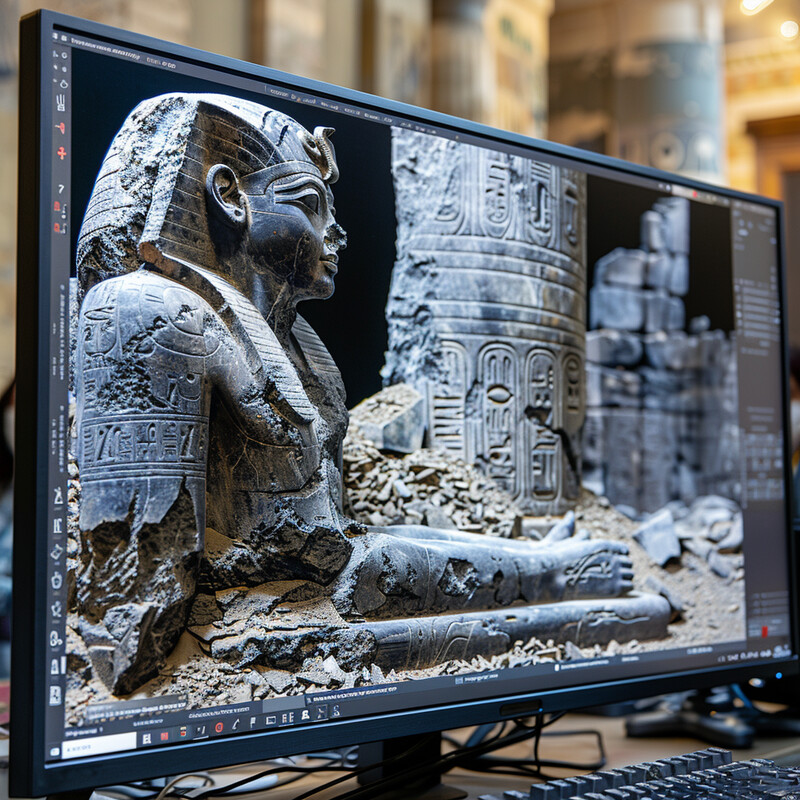
AI can reconstruct how damaged artifacts and structures might have originally appeared by analyzing their remnants and comparing them with similar intact findings. These AI-driven reconstructions provide valuable visual insights that help archaeologists and historians understand the original context and usage of these artifacts.
5. Deciphering Ancient Texts
AI helps in decoding and translating ancient scripts by recognizing character patterns and suggesting possible meanings based on linguistic databases.
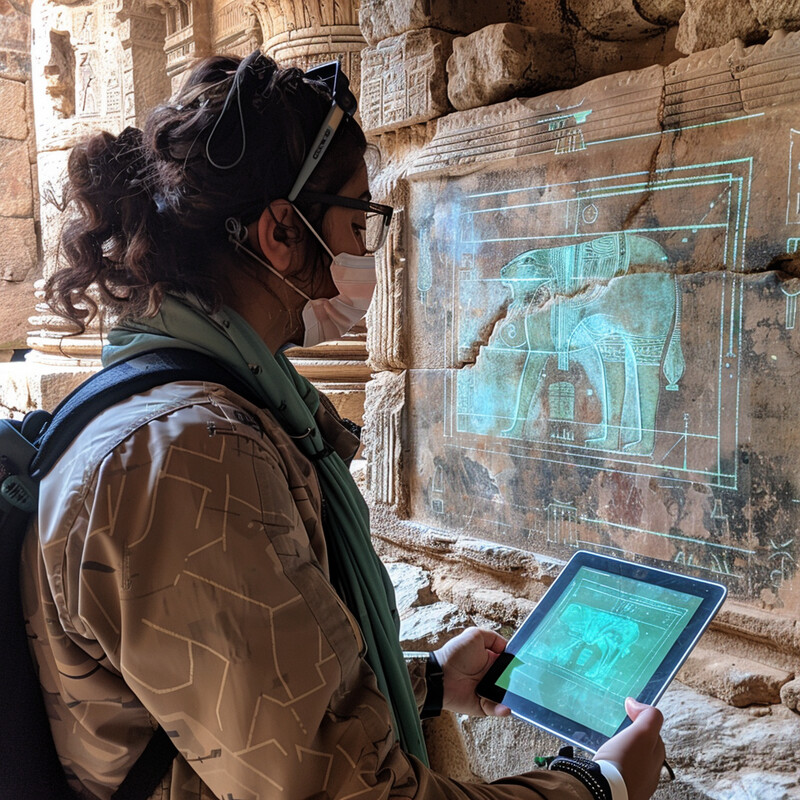
AI enhances the decipherment of ancient texts by using pattern recognition to identify recurring symbols and characters. It correlates these with known languages and scripts to suggest possible translations. This tool is particularly useful in cases where the language has not been fully decoded, offering new ways to unlock historical narratives.
6. 3D Modeling and Simulations
AI assists in creating 3D models of archaeological sites and artifacts, which can be used for virtual tours, detailed analysis, and educational purposes.
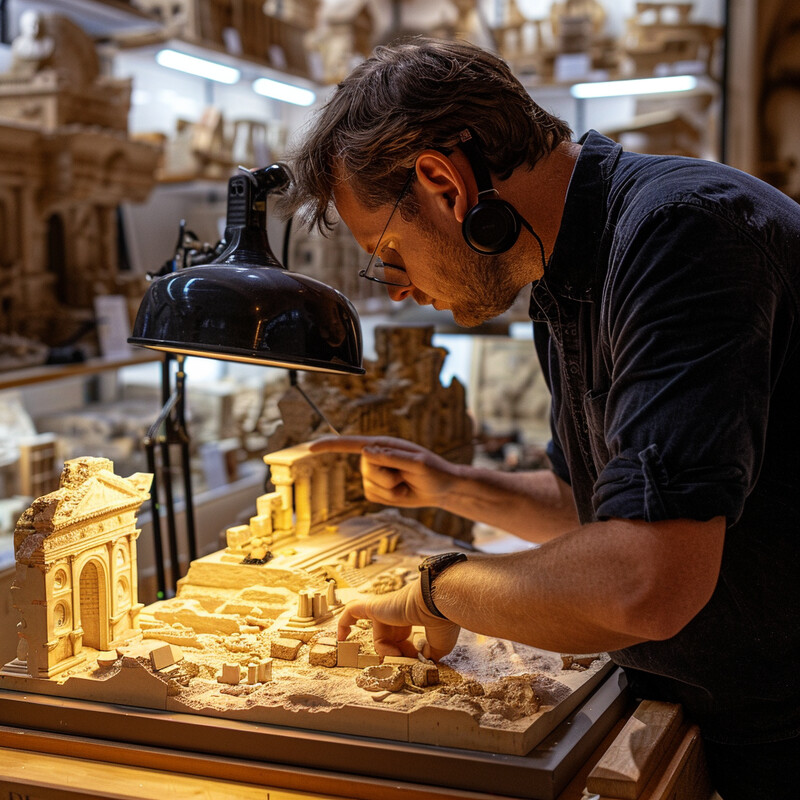
AI facilitates the creation of detailed 3D models of archaeological sites and artifacts. These models can be used for more than just virtual tours; they provide a platform for conducting simulations, engaging in digital preservation, and sharing detailed replicas with the global academic community, enhancing collaborative research and education.
7. Temporal Analysis
AI examines the stratification of archaeological sites to help determine the chronological sequence of historical layers, enhancing the understanding of how a site was used over time.
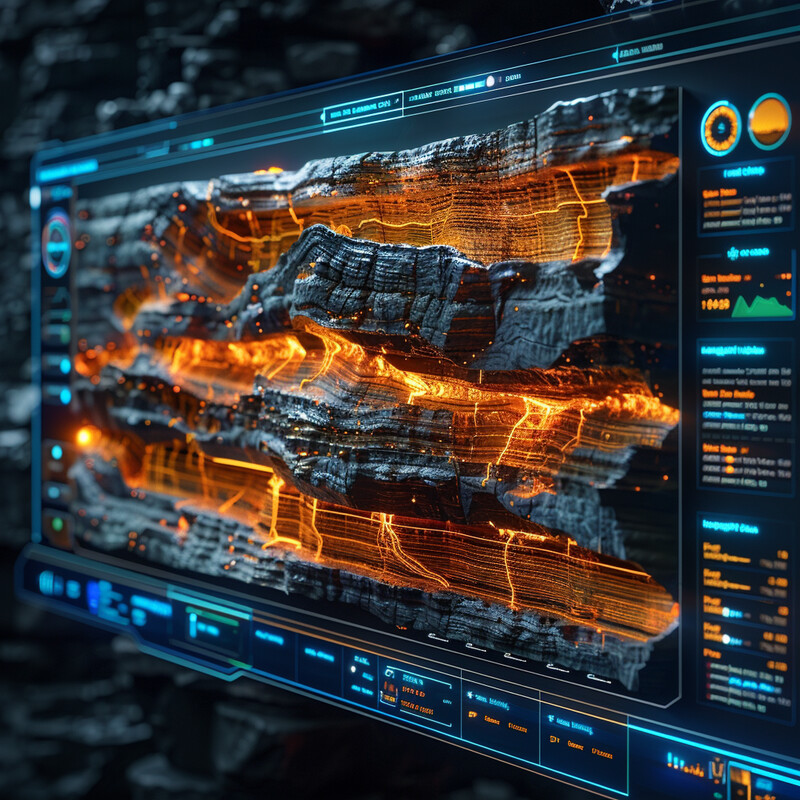
AI examines the layers of soil and deposition at archaeological sites to help determine the chronological order of cultural deposits. By automating the analysis of stratification, AI aids archaeologists in reconstructing the timeline of how a site was used, which is crucial for understanding the historical context of the findings.
8. Analysis of Human Remains
AI techniques in bioarchaeology help determine age, sex, diet, and health of human remains found at archaeological sites, providing insights into past human populations.
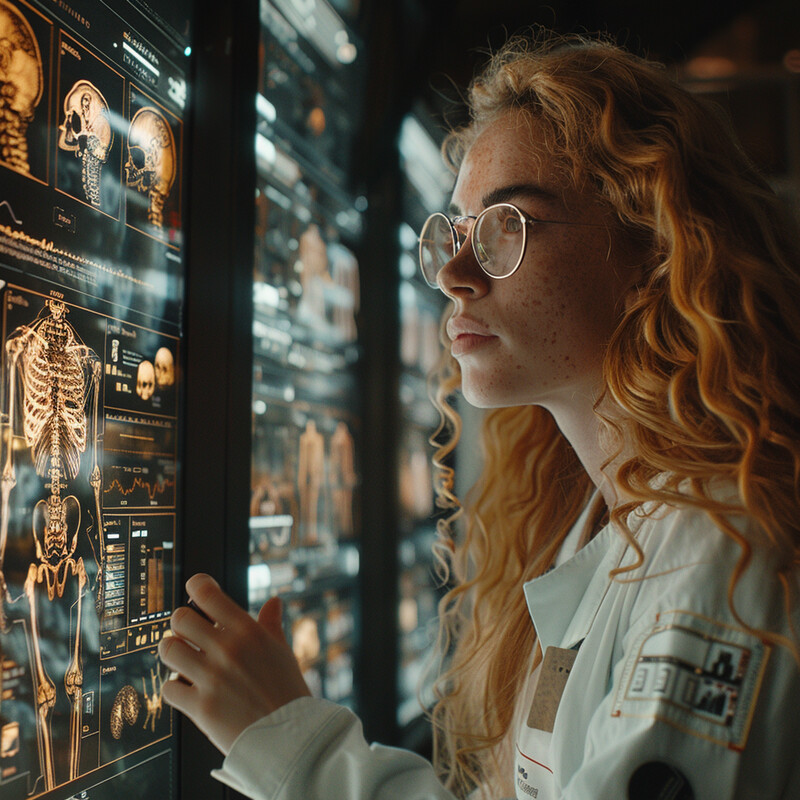
In bioarchaeology, AI techniques are applied to analyze human remains more precisely. AI can assess factors such as age at death, biological sex, diet, disease, and overall health based on measurements and patterns identified in skeletal remains, offering deeper insights into the lives of past populations.
9. Remote Sensing Data Analysis
AI processes data from remote sensing technologies, such as LIDAR, to uncover hidden features of landscapes, revealing buried structures or modifications made by ancient civilizations.
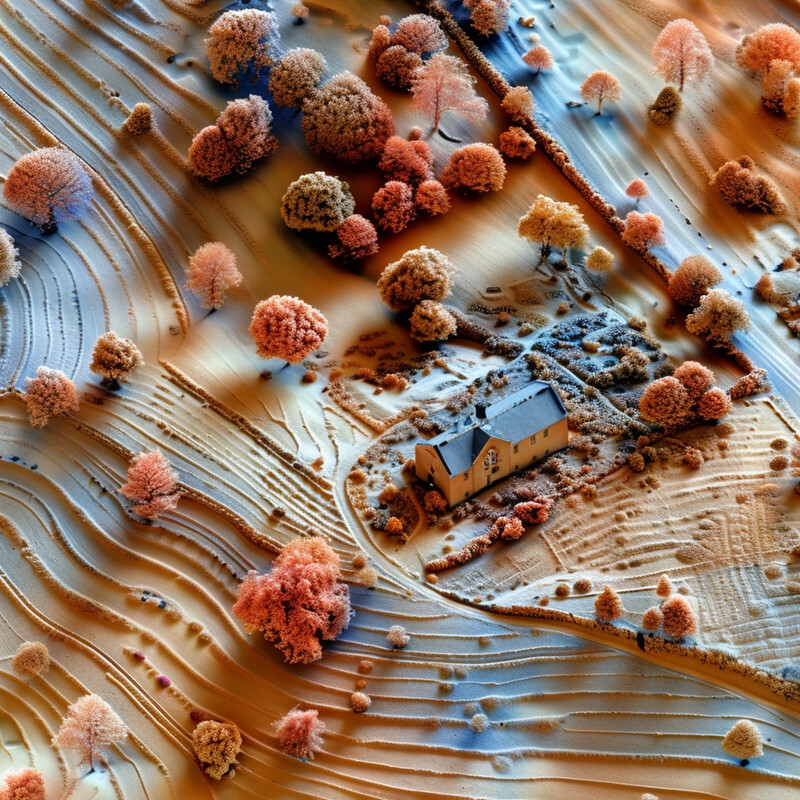
AI processes large volumes of data from remote sensing technologies, such as LIDAR, which penetrates forest canopies to reveal ground features. AI's ability to analyze this data efficiently helps uncover hidden structures and landscape modifications made by ancient civilizations, providing a clearer picture of historical land use.
10. Automated Documentation
AI streamlines the documentation process by automatically recording data during excavations, organizing information into databases, and ensuring detailed and accurate record-keeping.
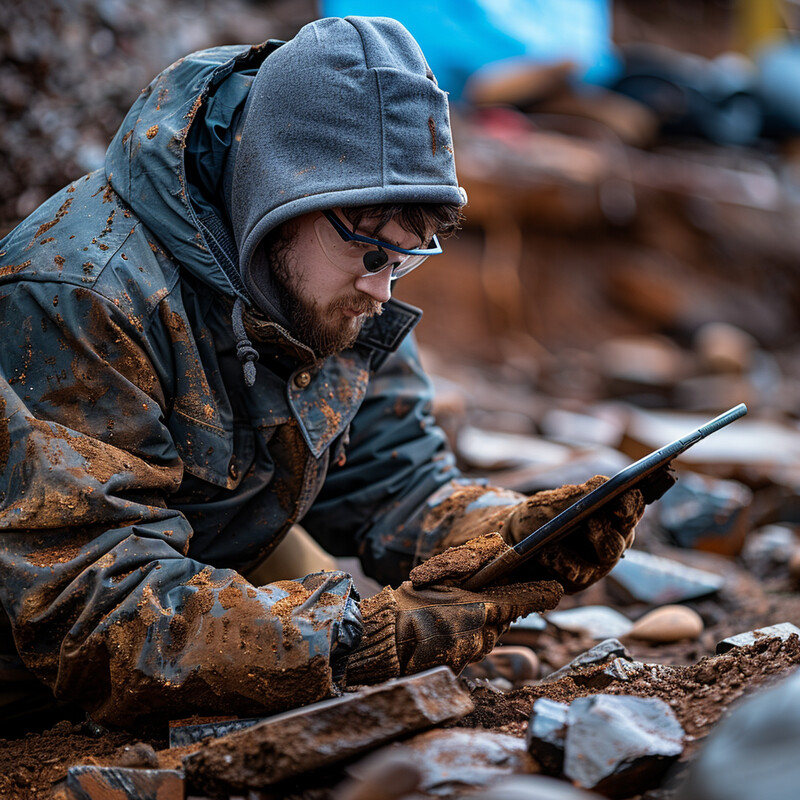
During excavations, AI helps in the automated documentation of findings. It systematically records details about the location, depth, and context of each artifact or feature uncovered, ensuring that all data is meticulously cataloged and easily accessible for future analysis. This streamlined approach improves the accuracy of field reports and enhances the archival process.