1. Automated Identification
AI algorithms can automatically identify individual animals from camera trap images or drone footage by analyzing unique features like stripes, spots, or facial characteristics.
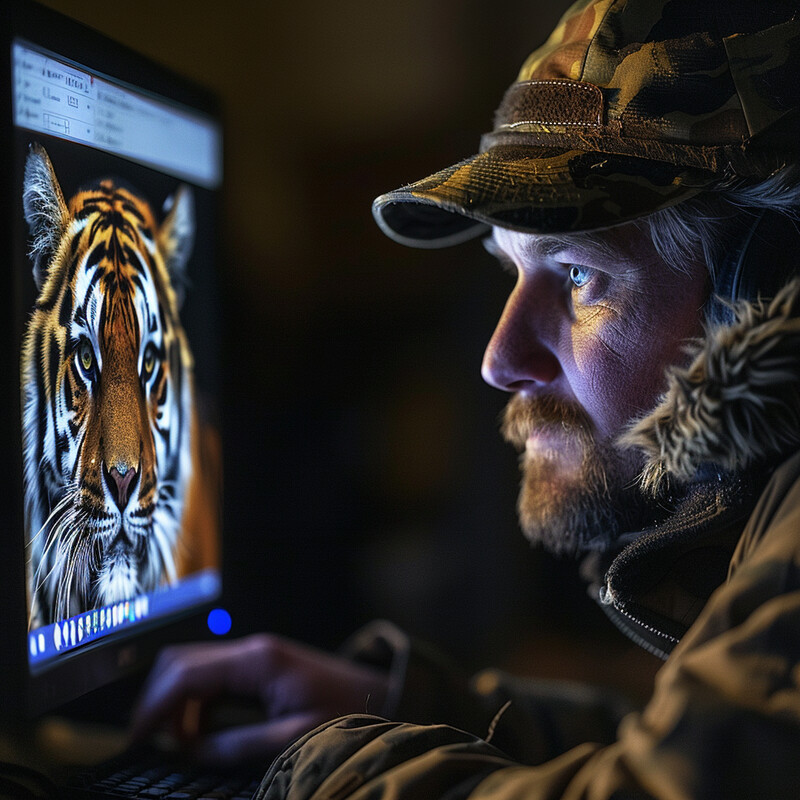
AI algorithms are increasingly used to automatically identify individual animals in wildlife research. These systems analyze images captured by camera traps, drones, or handheld devices to recognize unique identifiers such as stripes, spots, or facial features. This technology speeds up the process of cataloging individuals within a population, aiding in studies of animal behavior and population dynamics without the need for invasive tagging or direct interaction.
2. Population Monitoring
AI assists in estimating animal populations by processing images and videos to count individuals, helping conservationists track species numbers and monitor trends over time.
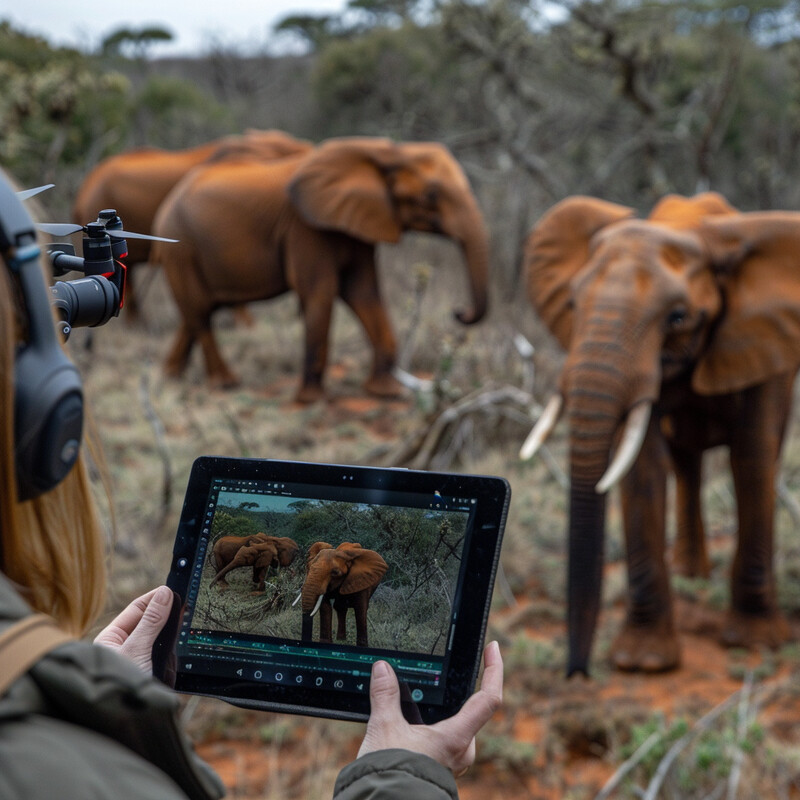
AI helps in accurately estimating animal populations by automating the analysis of images and videos to count individuals. This capability is crucial for monitoring species numbers, assessing conservation status, and understanding demographic trends. AI-driven population monitoring provides more reliable data, helping conservationists make informed decisions about species management and protection strategies.
3. Behavior Analysis
AI tools analyze audio and video data to study animal behavior, such as migration patterns, feeding habits, and social interactions, providing insights that are crucial for effective conservation strategies.
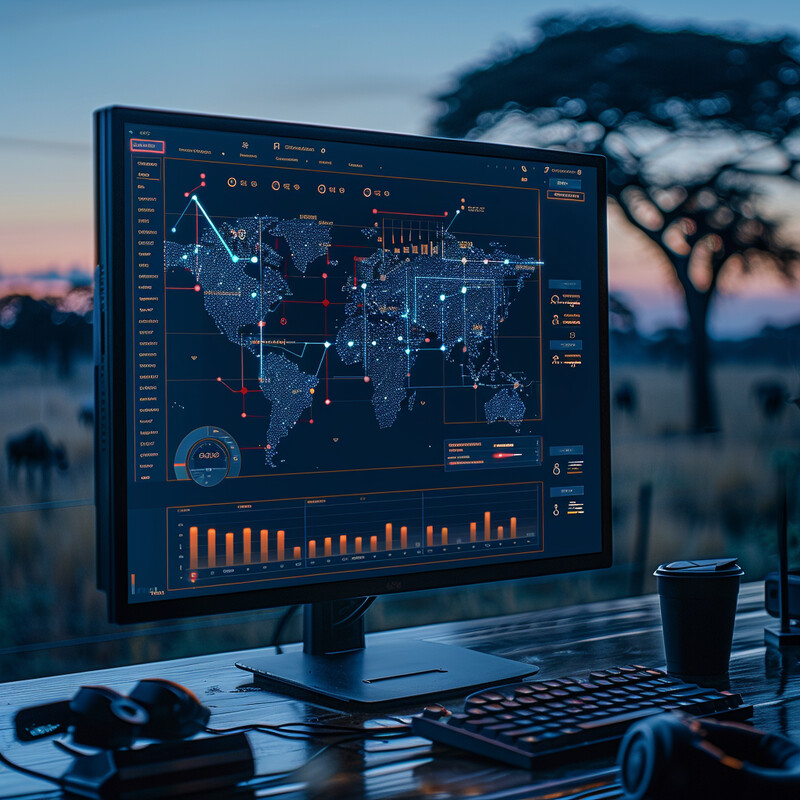
AI tools are adept at analyzing audio and video data to study detailed aspects of animal behavior. This includes tracking movements, migration patterns, feeding habits, and social interactions. By automating behavior analysis, AI enables researchers to gather comprehensive data over large temporal and spatial scales, providing deeper insights into the natural behaviors and needs of wildlife.
4. Habitat Analysis
AI evaluates satellite imagery and environmental data to map and monitor habitat conditions, identifying changes that could impact wildlife, such as deforestation, urban encroachment, or climate change effects.
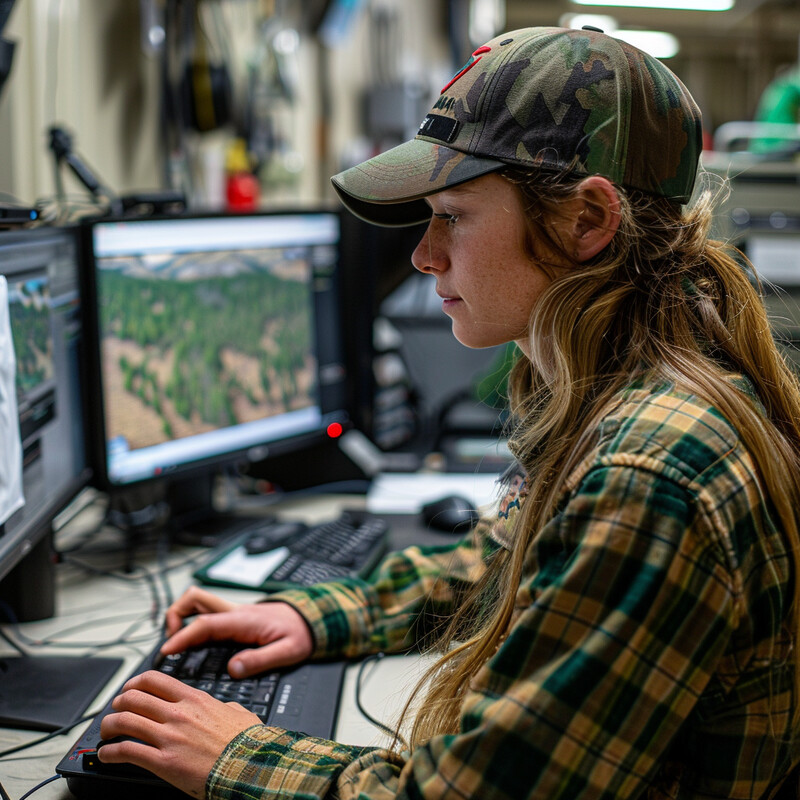
AI evaluates satellite imagery and environmental data to assess the quality and changes in wildlife habitats. It can detect alterations such as deforestation, urban encroachment, or changes in land use that pose threats to natural ecosystems. This information is vital for developing strategies to mitigate negative impacts and for restoring and preserving critical habitats.
5. Illegal Activity Detection
AI systems monitor surveillance footage from protected areas to detect illegal activities like poaching or unauthorized entry, alerting park rangers and law enforcement in real time.
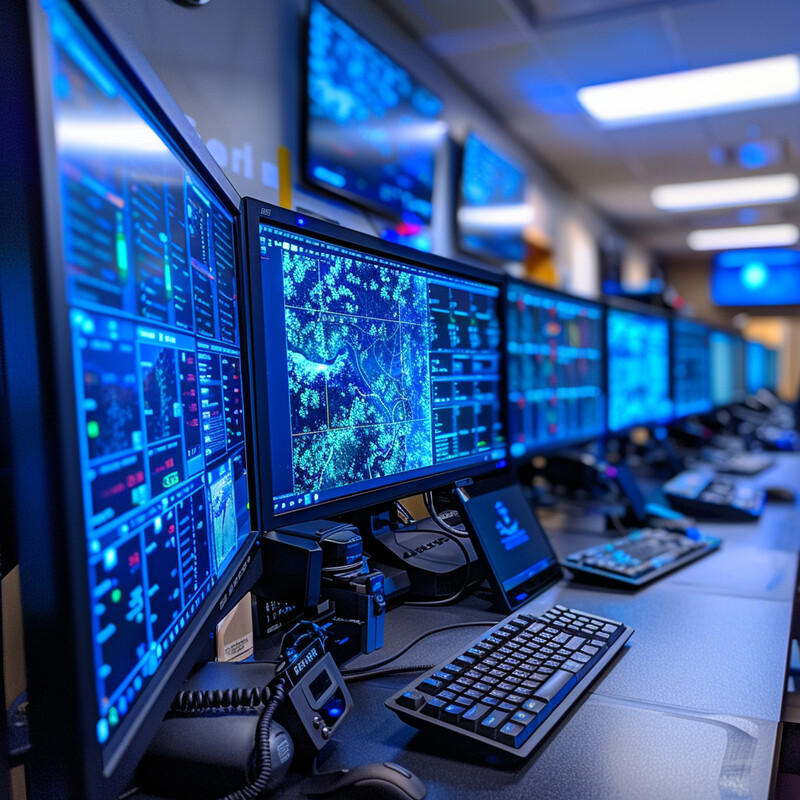
In protected areas, AI-powered surveillance systems play a crucial role in detecting illegal activities such as poaching or trespassing. AI analyzes video feeds in real-time to identify unauthorized human activities and can alert park rangers and law enforcement immediately, enabling quicker responses to protect wildlife and deter illegal activities.
6. Health Monitoring
AI algorithms analyze data from collars and other tracking devices to monitor the health and stress levels of individual animals, detecting signs of illness or distress for timely intervention.
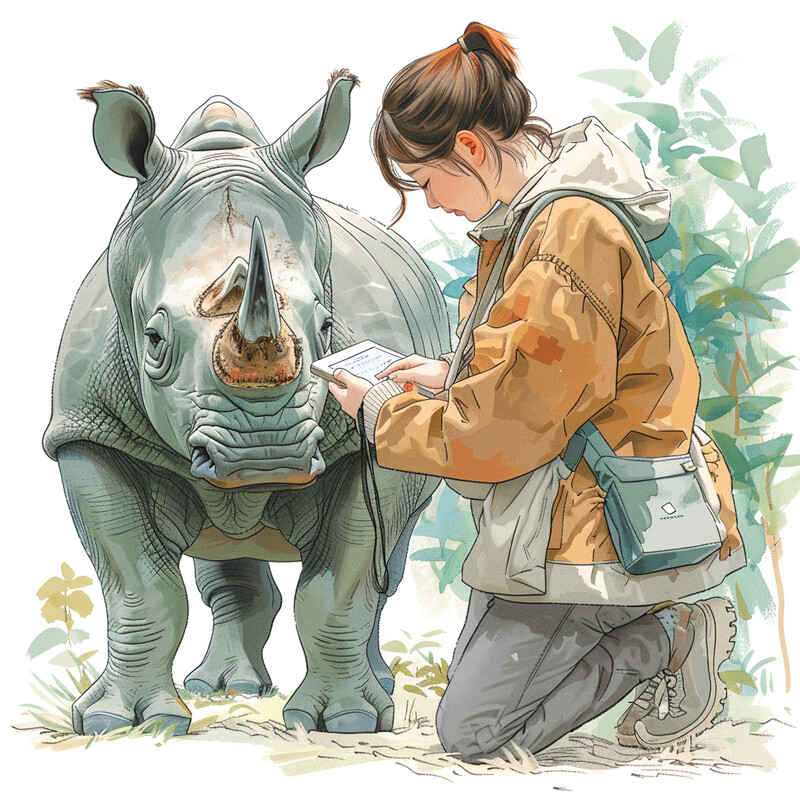
AI algorithms process data collected from tracking collars and other devices to monitor the health and stress levels of individual animals. This technology detects abnormalities that may indicate illness, injury, or distress, allowing for timely interventions to aid affected animals and prevent potential outbreaks or larger health issues within populations.
7. Predictive Modeling
AI uses historical data and current observations to predict future behaviors and potential threats to wildlife, such as disease outbreaks or conflicts with human populations.
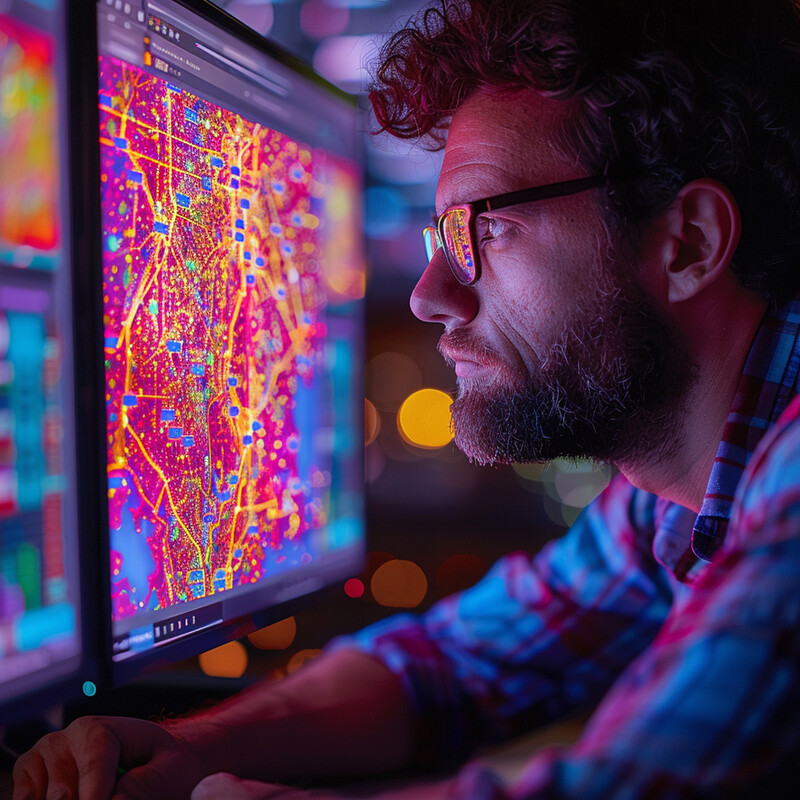
Using historical data and current observations, AI models predict future behaviors and potential ecological threats. These predictions help in proactively managing human-wildlife conflicts, anticipating disease outbreaks, or preparing for environmental changes that could impact wildlife, thereby improving the effectiveness of conservation efforts.
8. Genetic Analysis
AI accelerates the processing and interpretation of genetic data from wildlife populations, aiding in the study of genetic diversity and the management of breeding programs to sustain healthy populations.
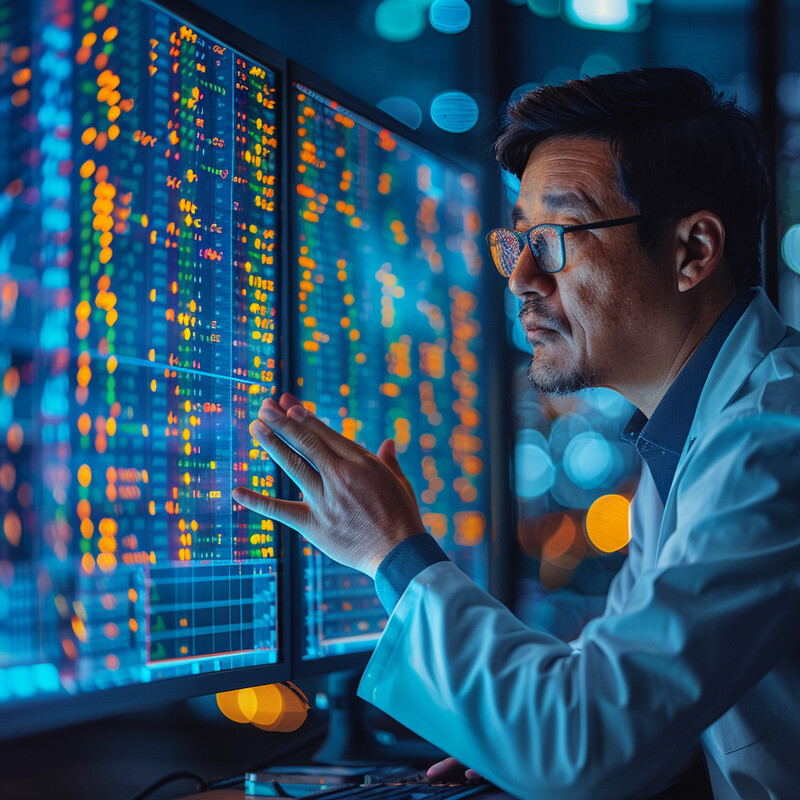
AI significantly speeds up the processing and interpretation of genetic information from wildlife. This analysis is essential for understanding genetic diversity within populations, managing breeding programs, and supporting efforts to maintain or increase genetic health in endangered species populations.
9. Acoustic Monitoring
AI distinguishes between different animal calls recorded in the wild, helping researchers track species presence and abundance in extensive, remote areas without physical intrusion.
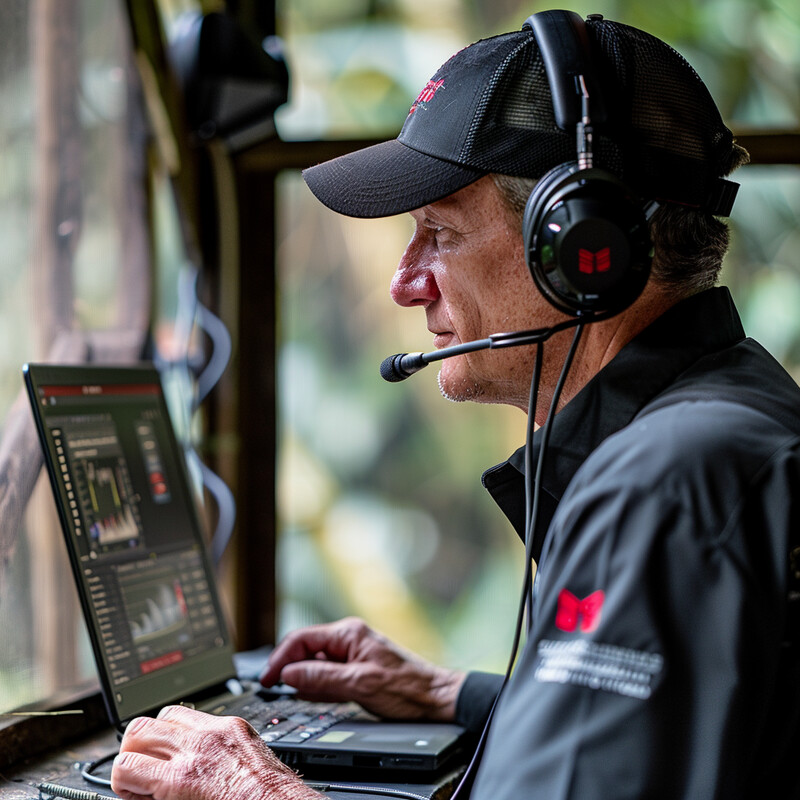
AI is used to analyze audio recordings from natural habitats, identifying and distinguishing between the calls of different animal species. This method allows researchers to monitor presence and population changes of species over large areas without the need for physical trapping or sighting, reducing the impact on the animals and improving the scope of monitoring programs.
10. Conservation Education and Awareness
AI-driven interactive platforms and applications educate the public about wildlife and conservation issues, using engaging formats like virtual reality or gamified learning to raise awareness and foster support for conservation efforts.
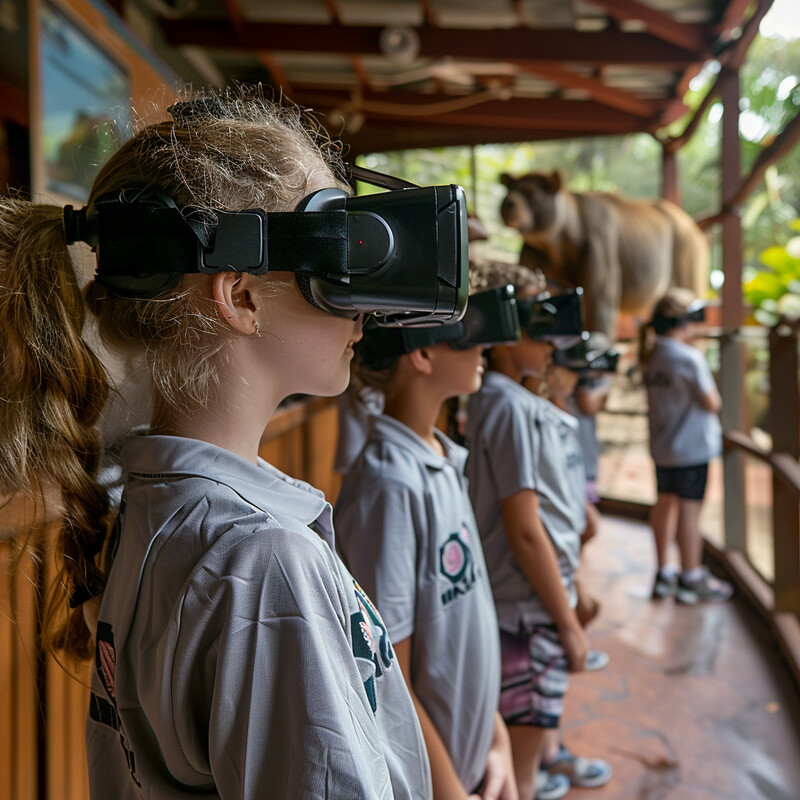
AI-driven platforms engage the public in wildlife conservation through interactive and immersive learning experiences. By employing virtual reality, gamified elements, and interactive educational content, AI helps raise awareness about conservation issues, promoting public understanding and support for wildlife protection efforts.