The rise of generative artificial intelligence (AI) technologies has transformed various fields, from creating realistic images and text to synthesizing speech and music. These innovations, driven by deep learning models, require vast amounts of high-quality data for training. However, as the costs of obtaining such datasets are high, many developers are turning to synthetic data produced by the models themselves. While this approach can be cost-effective, it introduces significant challenges that need careful management to maintain the integrity and performance of AI systems. The paper titled "When AI Eats Itself: On the Caveats of Data Pollution in the Era of Generative AI" by Xiaodan Xing et al. addresses the critical issue of data pollution resulting from the use of synthetic data in training generative AI models.
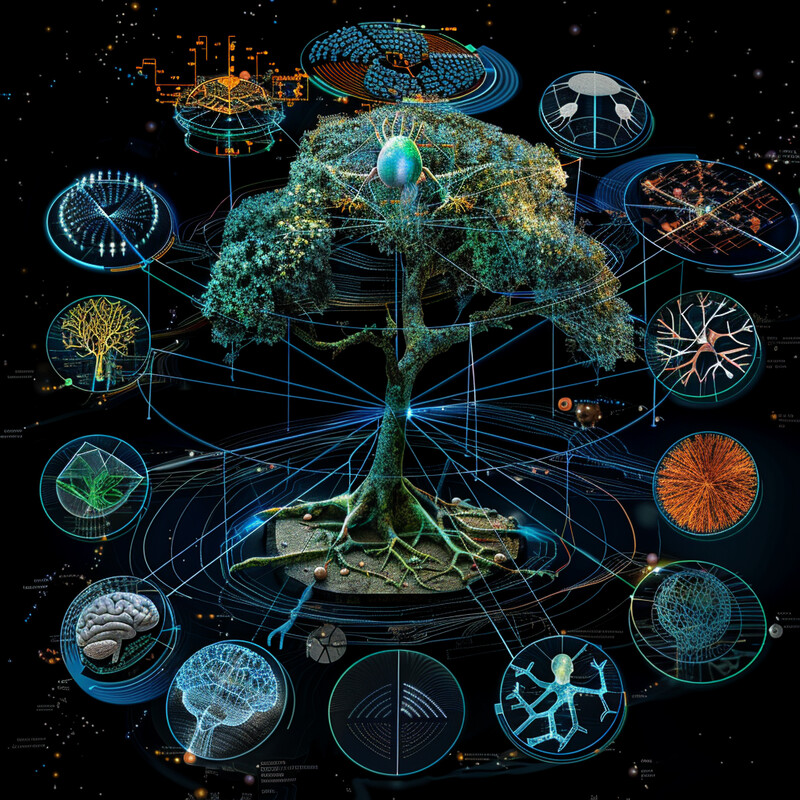
A key issue emerging from the use of synthetic data is the phenomenon known as AI autophagy, where AI systems consume their outputs to generate new data. This recursive process can lead to quality degradation and a loss of variety in the data over successive generations. For instance, generative models like StyleGAN2 and GPT-3.5 have shown patterns of performance decline, with image models developing visual artifacts and text models producing repetitive or nonsensical phrases. The risk is that, over time, these models may become less accurate and reliable, affecting their utility in real-world applications.
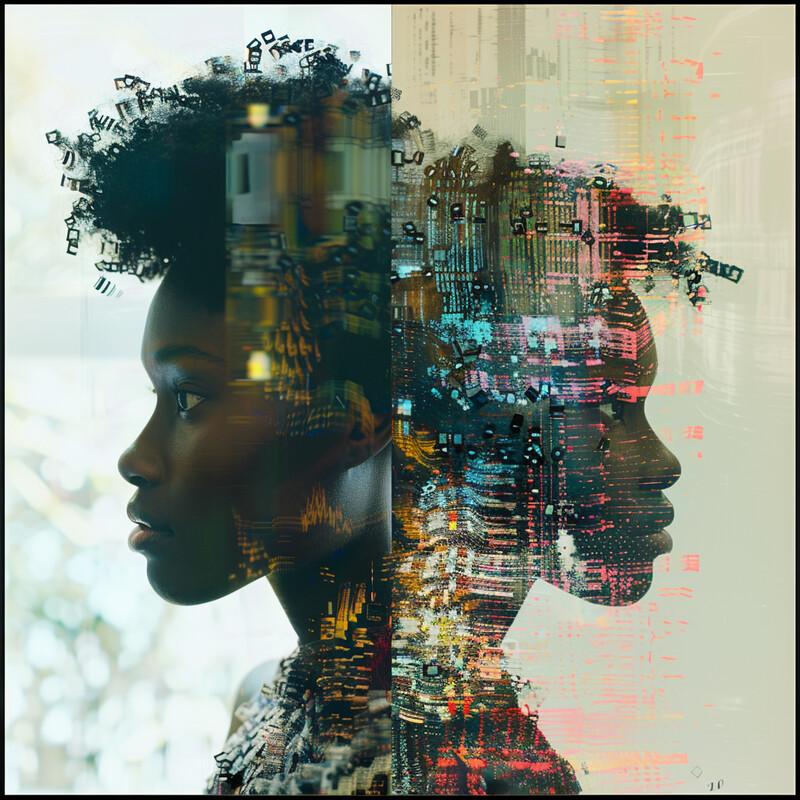
To address these challenges, it is crucial to implement technical strategies that can mitigate the negative impacts of AI autophagy. One effective approach is watermarking, which involves embedding identifiable markers within synthetic content. This technique helps distinguish AI-generated data from human-generated data, ensuring that synthetic content does not unintentionally degrade the training datasets. Additionally, detection methods that identify synthetic data based on inherent differences from real data can be employed. These methods can enhance the ability to filter out low-quality synthetic content before it affects model training.
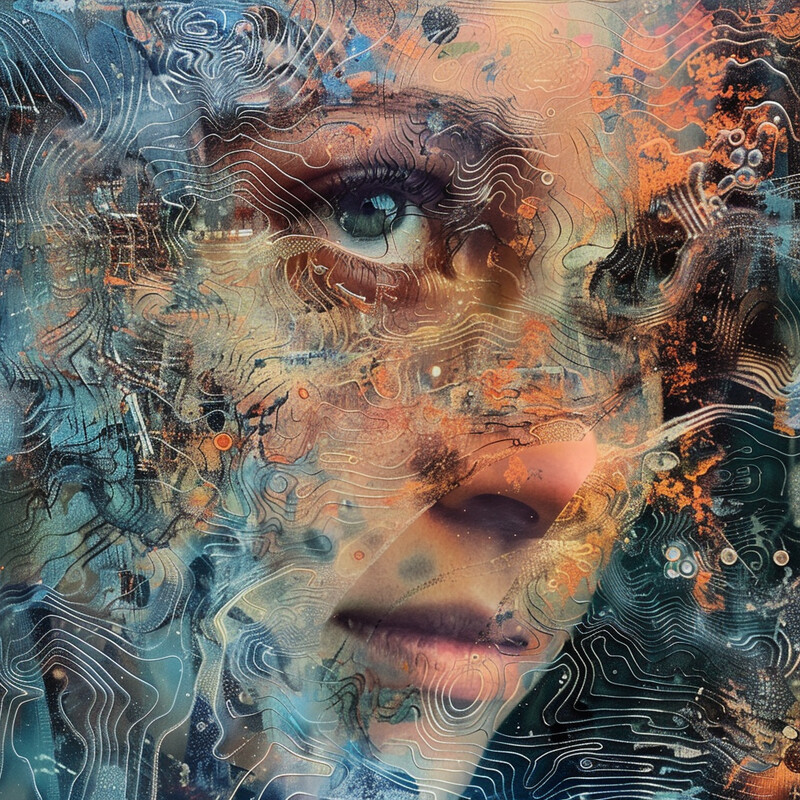
However, technical solutions alone are not sufficient. Regulatory measures play a vital role in managing the dissemination and use of synthetic data. Policies requiring clear labeling of AI-generated content and real-name verification for content creators can improve transparency and accountability. These regulations can help prevent the spread of low-quality synthetic data and ensure that users are aware of the origins of the content they encounter. By combining technical and regulatory strategies, it is possible to develop a more sustainable approach to using synthetic data in generative AI.
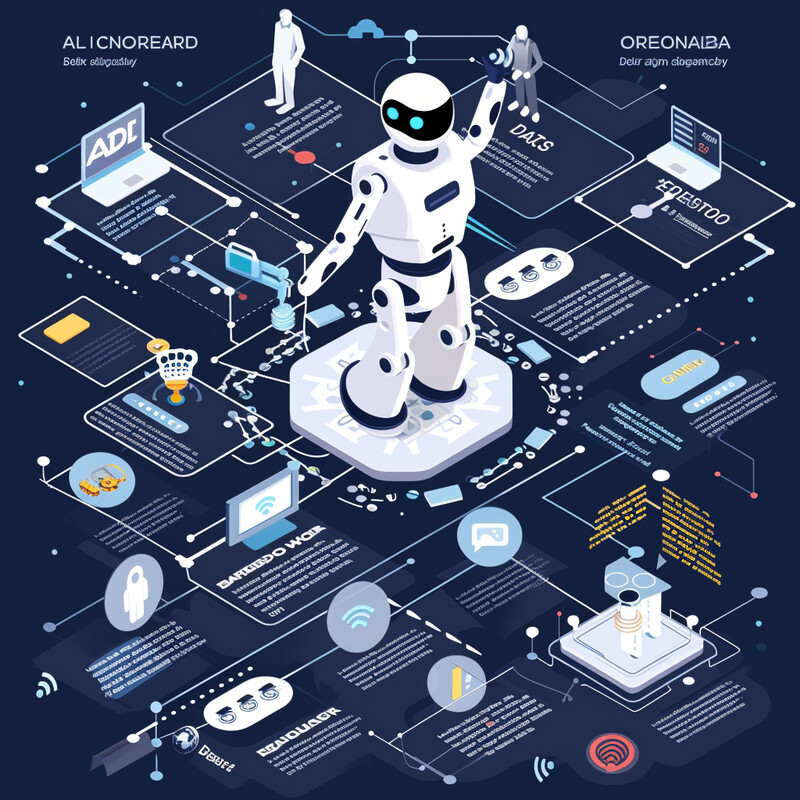
Ultimately, the sustainable development of generative AI technologies depends on a collaborative effort among technology developers, regulatory bodies, and the wider society. By recognizing the risks of AI autophagy and implementing comprehensive strategies to manage synthetic data, we can harness the full potential of generative AI while safeguarding the quality and integrity of the data that fuels these innovations. This balanced approach will support the continued advancement of AI technologies, enabling them to contribute positively to various domains without compromising their reliability or ethical standards.
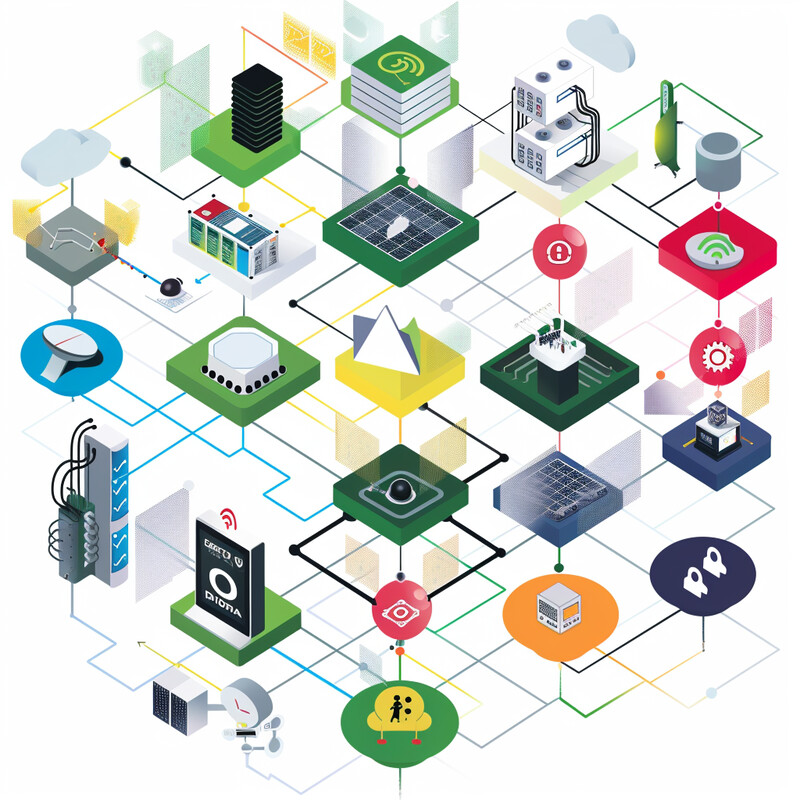
Reference: Xiaodan Xing et al., "When AI Eats Itself: On the Caveats of Data Pollution in the Era of Generative AI," arXiv:2405.09597 [cs.LG], 2024. https://doi.org/10.48550/arXiv.2405.09597